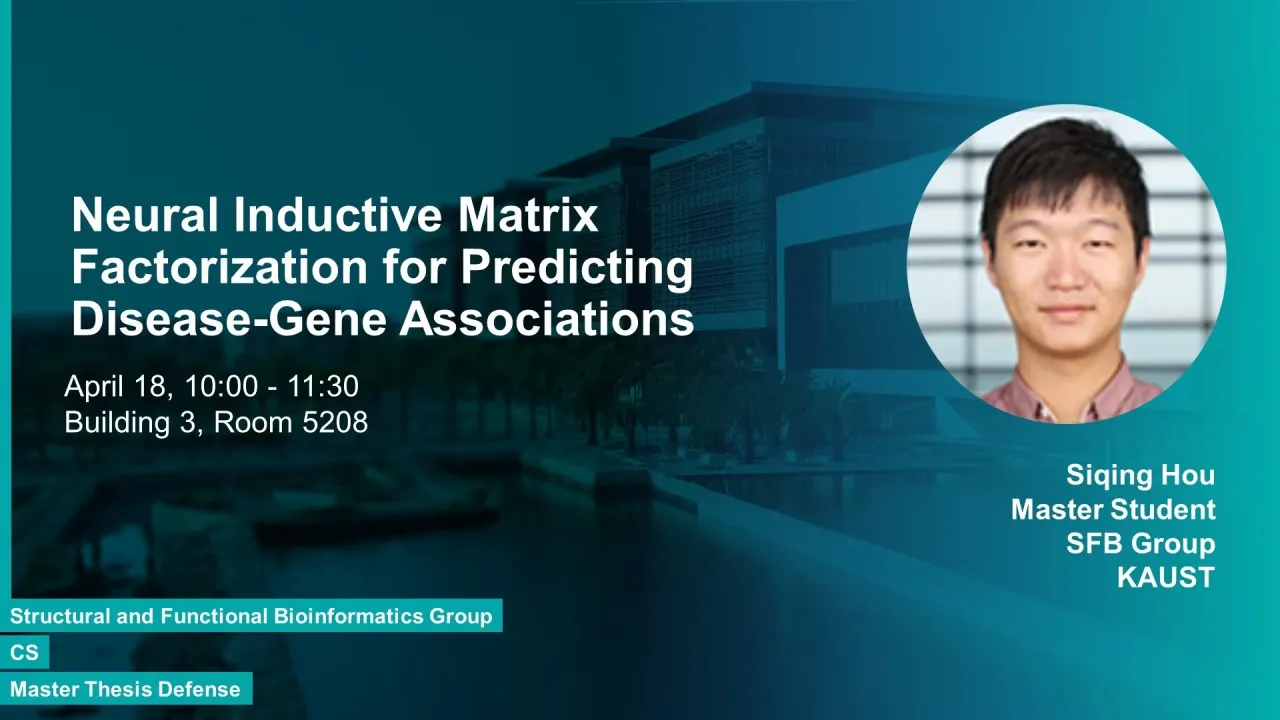
Neural Inductive Matrix Factorization for Predicting Disease-Gene Associations
In silico prioritization of undiscovered associations can help find causal genes of newly discovered diseases. Some existing methods are based on known associations and side information of diseases and genes. We exploit the possibility of using a neural network model, Neural Inductive Matrix Completion (NIMC) in disease-gene prediction.
Overview
Abstract
In silico prioritization of undiscovered associations can help find causal genes of newly discovered diseases. Some existing methods are based on known associations and side information of diseases and genes. We exploit the possibility of using a neural network model, Neural Inductive Matrix Completion (NIMC) in disease-gene prediction. Comparing to the state-of-the-art Inductive Matrix Completion method, using the neural networks allows us to learn latent features from non-linear functions of input features. Previous methods use disease features only from mining text. Comparing to text mining, disease ontology is a more informative way of discovering the correlation of diseases, from which we can calculate the similarities between diseases and help increase the performance of predicting disease-gene associations.
We compare the proposed method with other state-of-the-art methods for predicting associated genes for diseases from the Online Mendelian Inheritance in Man (OMIM) database. Results show that both new features and the proposed NIMC model can improve the chance of recovering an unknown associated gene in the top 100 predicted genes. Best results are obtained by using both the new features and the new model. Results also show the proposed method does better in predicting associated genes for newly discovered diseases.
Brief Biography
I am a master student in Prof. Xin Gao's group. I am working on machine learning and bioinformatics. I obtained my Bachelor of Engineering degree in Computer Science and Technology from the University of Science and Technology of China.