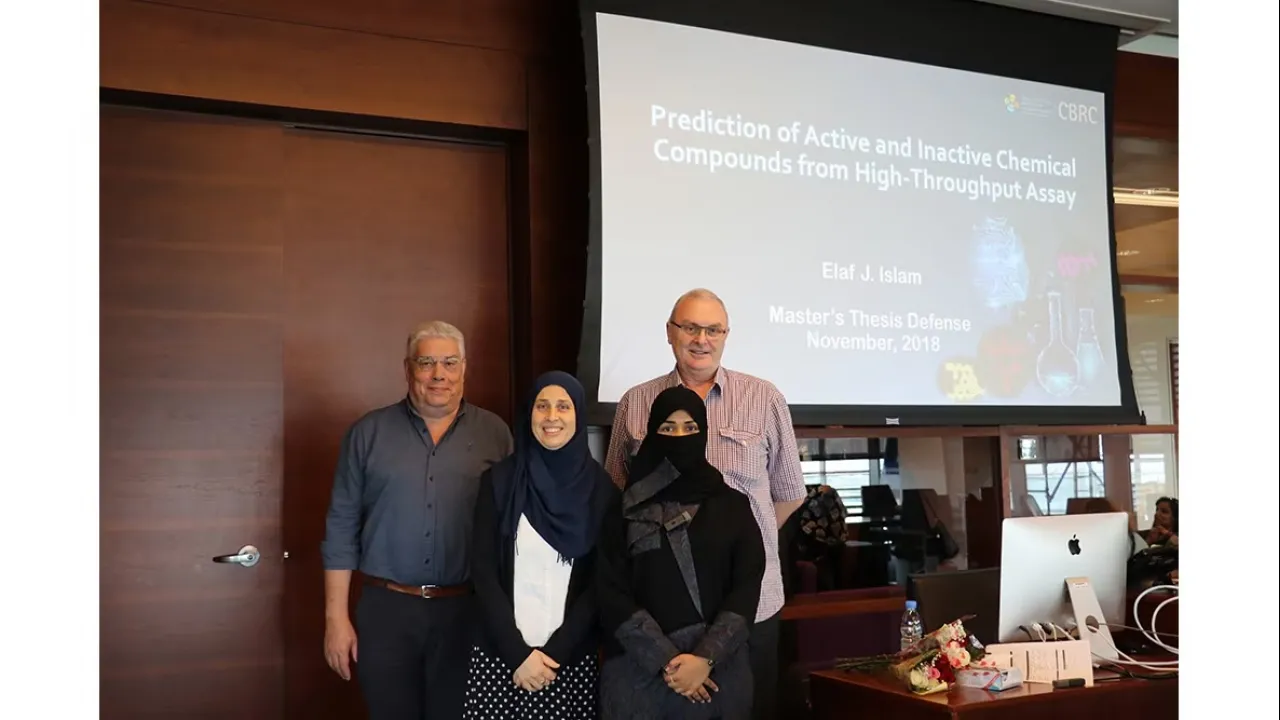
Prediction of active and inactive chemical compounds from high-throughput assays
Overview
Abstract
This study considers chemical compounds that can exert their activity by interacting with a target protein or other molecular receptor. Our aim is to develop machine learning models that can predict if a chemical compound will be active in a particular test/assay. We will use data from assays that are present in the PubChem knowledge base, specifically in its segment called BioAssays which reports the results of many high-throughput screening experiments. PubChem BioAssays is a valuable resource that contains information from a large number of experiments. In one assay, sometimes many hundreds or even many thousands of chemicals are tested. Data from these experimental assays contain information about chemicals that are active as well as chemicals that are not active in an assay. These represent an interesting resource of experimental data that are well suited for classification purposes. We will approach the problem by evaluating different ways that chemical compounds can be numerically described by means of so-called fingerprints, and then apply different machine learning (ML) and deep learning (DL) models to classify active and inactive chemicals for a number of assays. In this study, we will make comprehensive comparisons of the types of ML /DL models and types of fingerprint features that describe chemicals, and evaluate combinations of models and fingerprints that work best for the problem in question. Our focus is on finding those combinations which are useful for distinguishing active from inactive compounds in single PubChem assays. We will evaluate the methods across 10 assays and will examine the effects of 11 types of fingerprints. For example, PubChem fingerprints and MACCS keys fingerprints. For the evaluation, up to now we performed 88 experiments for each dataset and 968 in total for all 10 PubChem assays. These experiments involved approximately 6000 interactions between chemicals and their targets. The implementation of this project has been done using MATLAB toolbox. Based on these and additional experiments, we will be in a position to propose which combination of fingerprints and ML/DL models works best in the above-mentioned task. Such modeling will be useful to predict activity for chemicals that are not tested.
Brief Biography
Elaf Jameel Islam is a Master Student in Computer Science. She joint Knowledge Mining Laboratory in 2017. She was a teaching assistant at Taif University, Taif, Saudi Arabia. Her interests are bioinformatics, Data mining, Machine Learning, Deep Learning. Her researches focus on applying machine learning classifiers to predict drug activities, and evaluation of deep learning methods in recognition of genomic signals.