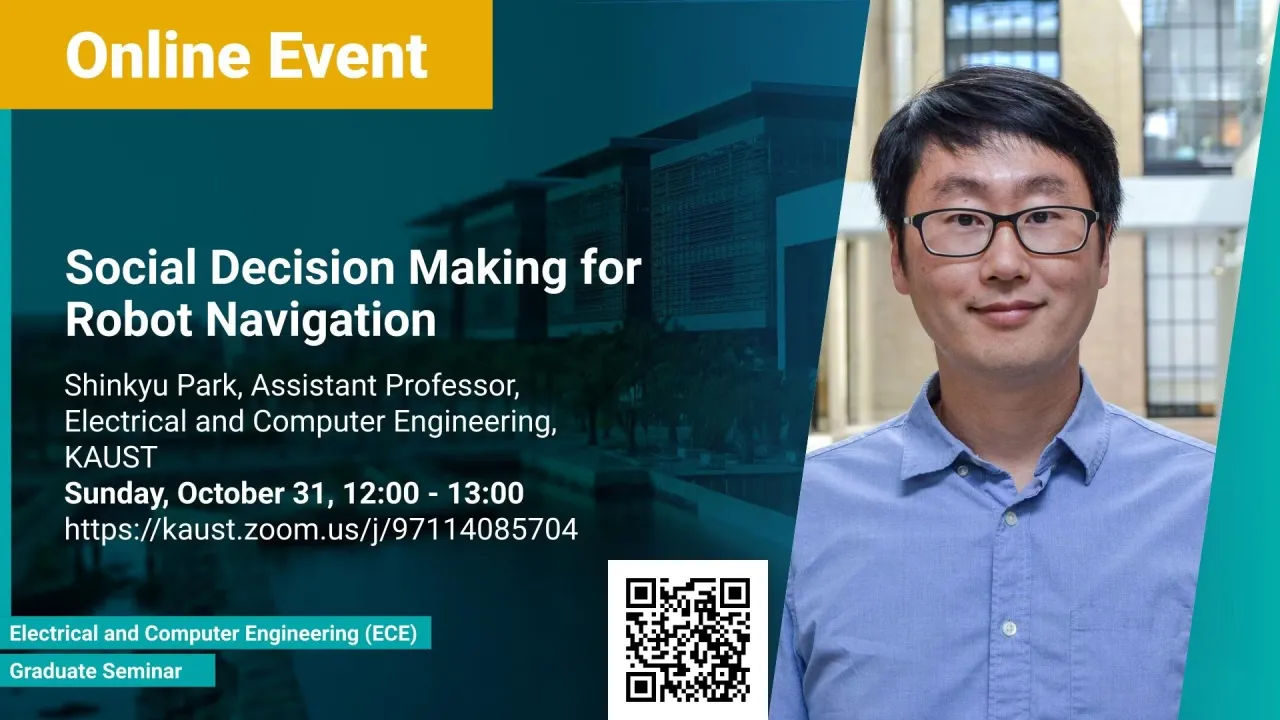
Social Decision Making for Robot Navigation
Robot navigation typically comprises of decision making at two different levels - global planning to compute a viable trajectory to the robot's destination and strategic (local) interaction to elicit cooperation and resolve any conflicts with other robots/pedestrians that would arise while navigating along the trajectory. Robot navigation in crowded environments is particularly challenging as the robot needs to exhibit navigation behaviors that are conceived as socially compliant by human pedestrians or vehicles they maneuver at both of the levels. In this presentation, I will introduce some of relevant works from my research group.
Overview
Abstract
Robot navigation typically comprises of decision making at two different levels - global planning to compute a viable trajectory to the robot's destination and strategic (local) interaction to elicit cooperation and resolve any conflicts with other robots/pedestrians that would arise while navigating along the trajectory. Robot navigation in crowded environments is particularly challenging as the robot needs to exhibit navigation behaviors that are conceived as socially compliant by human pedestrians or vehicles they maneuver at both of the levels. In this presentation, I will introduce some of relevant works from my research group.
In the first part of the presentation, I will present research on trajectory planning where we aim to enable autonomous surface vessels to perform socially compliant navigation in canal environments. We adopt an inverse reinforcement learning framework for autonomous vessels to learn navigation behavior of human-operated vessels, and we formalize the trajectory planning as data-driven optimal control to reward/penalize the robot's movements with respect to learned behaviors of human-operated vessels. We will discuss key features of the approach that allow the autonomous vessels to learn/exhibit socially compliant navigation behaviors and how the algorithm improves safety in canal navigation.
Next, I will describe research on designing a multi-agent decision-making model that elicits cooperation in social dilemmas. As a preliminary step, we will look into the prisoner's dilemma, which is a prototypical example of two-agent social dilemmas where individually rational agents prefer not to cooperate, and discuss a so-called opinion dynamics model that provides a principled and systematic means to investigate multi-agent decision making based on individual rationality and reciprocity, both of which are key features in human decision making that lead to cooperation. I will explain how dynamical system theory can be applied to assess stability of the model and explain the implication of the stability on the emergence of cooperation in the prisoner's dilemma.
Brief Biography
Shinkyu Park is an assistant professor of Electrical and Computer Engineering. Prior to joining KAUST, he was Associate Research Scholar at Princeton University engaged in cross-departmental robotics projects. He received the Ph.D. degree in electrical engineering from the University of Maryland College Park in 2015. Later he held Postdoctoral Researcher positions at the National Geographic Society (2016) and Massachusetts Institute of Technology (2016-2019). Park's research focuses on multi-robot learning/cooperation, underwater robotics, feedback control theory, and game theory. His past research projects include designing animal-borne sensor networks to monitor wild animal groups in their natural habitats. He also created a fleet of urban autonomous surface vessels capable of transporting people, providing deliveries, and trash removal services through urban canal networks.