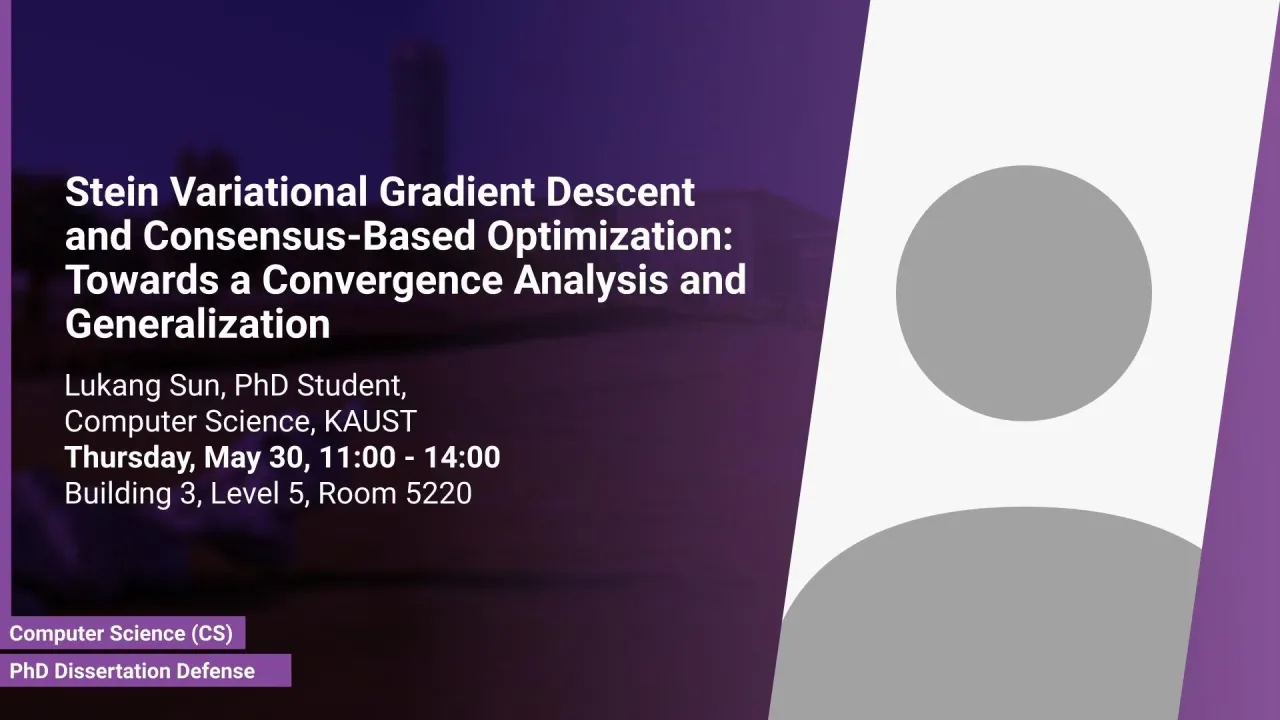
Stein Variational Gradient Descent and Consensus-Based Optimization: Towards a Convergence Analysis and Generalization
The first part of the dissertation presents a study on the convergence properties of Stein Variational Gradient Descent (SVGD), a sampling algorithm with applications in machine learning. The research delves into the theoretical analysis of SVGD in the population limit, focusing on its behavior under various conditions, including the Talagrand’s inequality T1 and the (L0, L1)−smoothness condition. The study also introduces an improved version of SVGD with importance weights, demonstrating its potential to accelerate convergence and enhance stability.
Overview
Abstract
The first part of the dissertation presents a study on the convergence properties of Stein Variational Gradient Descent (SVGD), a sampling algorithm with applications in machine learning. The research delves into the theoretical analysis of SVGD in the population limit, focusing on its behavior under various conditions, including the Talagrand’s inequality T1 and the (L0, L1)−smoothness condition. The study also introduces an improved version of SVGD with importance weights, demonstrating its potential to accelerate convergence and enhance stability.
In the second part, the dissertation explores the convergence of Consensus-Based Optimization (CBO) methods. We first propose the Consensus-Based Optimization with truncated noise, for this method, we provide theoretical guarantees for global convergence to the global minimizer of nonconvex and nonsmooth objective functions. We also design a CBO dynamic to find the global minimizers of objectives with multiple global minimizers. For this dynamic, we provide the theoretical guarantee that the dynamic will concentrate around the set of global minimizers of the target objectives.
Brief Biography
Lukang Sun is a Ph.D. student majoring in Computer Science under the supervision of Prof. Peter Richtarik since 2021. At KAUST, Lukang Sun’s research focuses on interacting particle systems and their applications to machine learning, engineering, etc.