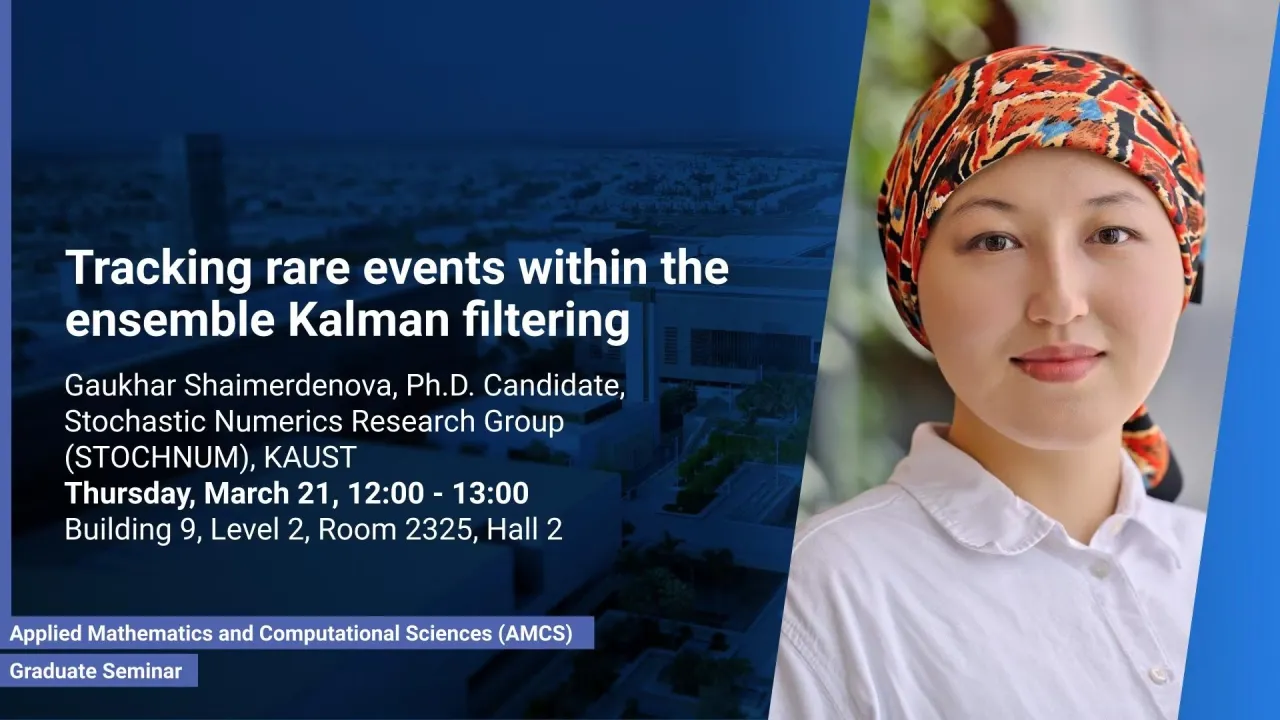
Tracking rare events within the ensemble Kalman filtering
In this work, we employ importance sampling (IS) techniques to track a small over-threshold probability of a running maximum associated with the solution of a stochastic differential equation (SDE) within the framework of ensemble Kalman filtering (EnKF).
Overview
Abstract
In this work, we employ importance sampling (IS) techniques to track a small over-threshold probability of a running maximum associated with the solution of a stochastic differential equation (SDE) within the framework of ensemble Kalman filtering (EnKF). Between two observation times of the EnKF, we propose to use IS with respect to the initial condition of the SDE, IS with respect to the Wiener process via a stochastic optimal control formulation, and combined IS with respect to both initial condition and Wiener process. Both IS strategies require the approximation of the solution of Kolmogorov Backward equation (KBE) with boundary conditions. Solving the KBE is computationally not feasible in high dimensions. To overcome this issue, we employ a Markovian projection dimension reduction technique to obtain an approximation of the solution of the KBE by just solving a one dimensional PDE. The proposed ideas are tested on two illustrative examples: Double Well SDE and Langevin dynamics, and showcase a significant variance reduction compared to the standard Monte Carlo method and another sampling-based IS technique, namely, multilevel cross entropy.
Brief Biography
A PhD candidate at Stochastic Numerics Research Group (STOCHNUM) under the supervision of Professor Raul F. Tempone, AMCS, KAUST. She obtained a Bachelor's degree in Mathematics at Eurasian National University, Kazakhstan, and a Master's degree in Financial Mathematics at University College London, UK.