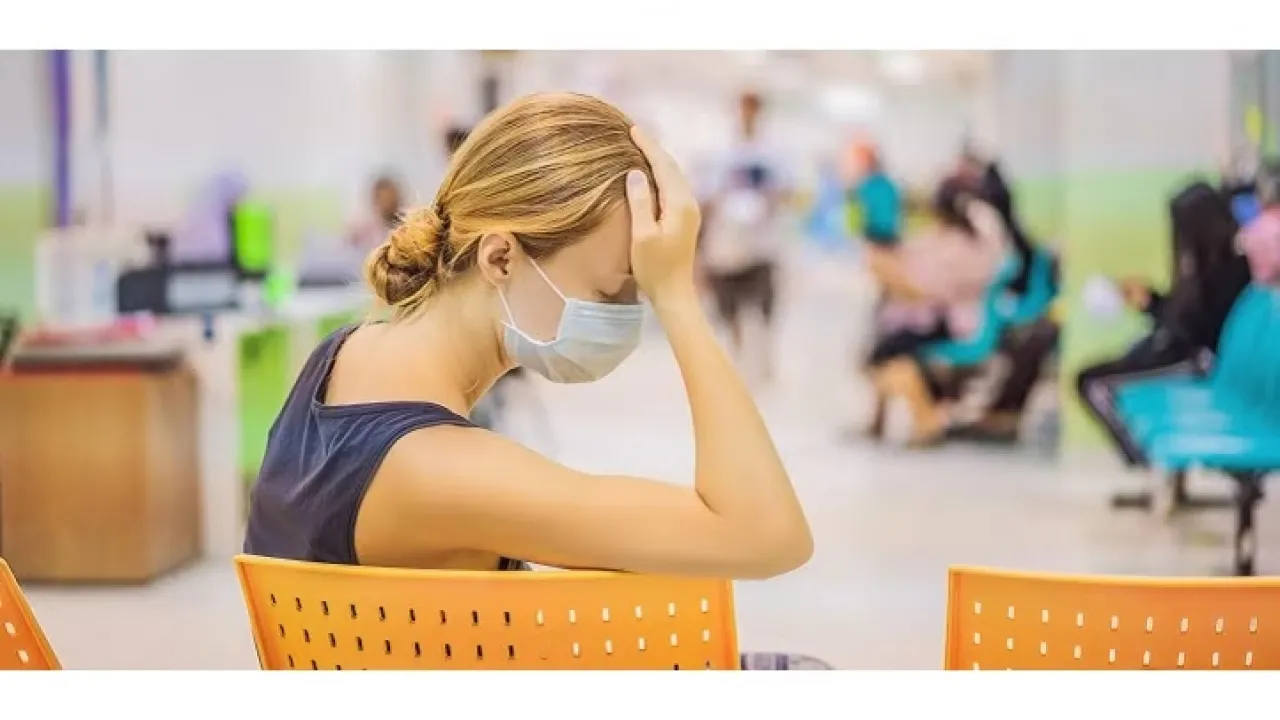
Deep learning in the emergency department
Harnessing the power of deep learning leads to better predictions of patient admissions and flow in emergency departments.
About
Using a deep-learning model designed for high-dimensional data, KAUST researchers have shown that it is possible to predict emergency department overcrowding from complex hospital records. This application of the "Variational AutoEncoder" deep-learning model is an example of how machine learning can be used to interpret and extract meaning from difficult data sets that are too voluminous or complex for humans to decipher.
Machine learning is an important aspect of artificial intelligence (AI) that involves training an AI model using training data. An AI model could learn, for example, to recognize images of the number three by training it using a data set containing thousands of versions of handwritten numerals. A simple neural network model—comprising interconnected "neurons" that take an input, apply a rule and produce an output— becomes increasingly accurate as it is exposed to more training data and the rules on each neuron are refined.
By adding hidden intermediate layers of neurons into these networks, however, the model can be prompted to self-learn the relationships in the input data without the rules being specified in advance. Such models, known as deep-learning models, are extremely powerful because they allow us, for the first time, to interpret data that has previously been too large, heterogeneous or multiparametered to meaningfully analyze any other way.
“Deep learning has emerged as a promising line of research in modeling and forecasting, in both academia and industry,” says Fouzi Harrou, a research scientist at KAUST. “These models can automatically extract information from voluminous datasets with limited human instruction, such as implicit relationships between variables, complicated pattern recognition and descriptions of dependencies in time series data.”
Read the full article