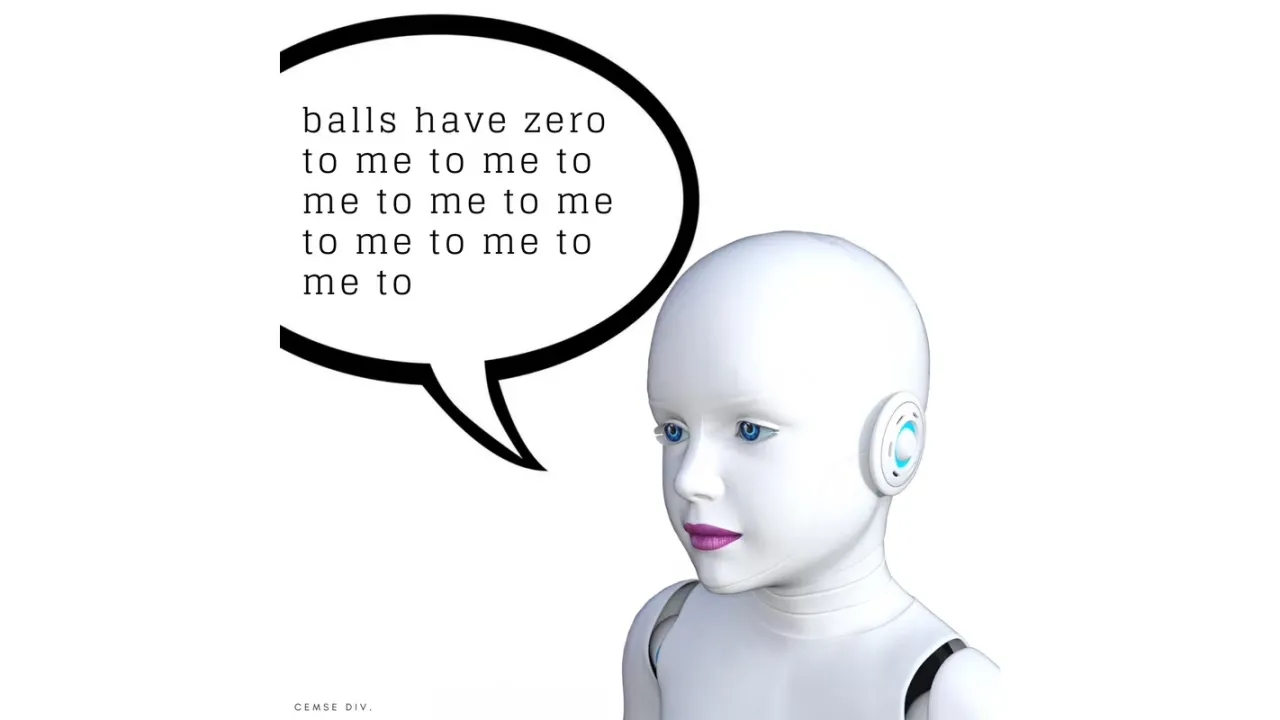
Facebook AI new language explained by KAUST Professor Xiangliang Zhang
That is not a Messenger troll, but the actual conversation between two chatbots by the Facebook AI Research (FAIR). The two robots, Bob and Alice, were thought the art of negotiation apples and books. They had been instructed to work out how to negotiate between themselves and improve their bartering as they went along. But, after leaving the pair alone, they start talking in this uncompressible but yet effective vocabulary.
About
Bob: i can i i everything else . . . . . . . . . . . . . .
Alice: balls have zero to me to me to me to me to me to me to me to me to
Bob: you i everything else . . . . . . . . . . . . . .
Alice: balls have a ball to me to me to me to me to me to me to me
Bob: i i can i i i everything else . . . . . . . . . . . . . .
Alice: balls have a ball to me to me to me to me to me to me to me
Bob: i . . . . . . . . . . . . . . . . . . .
Alice: balls have zero to me to me to me to me to me to me to me to me to
That is not a Messenger troll, but the actual conversation between two chatbots by the Facebook AI Research (FAIR). The two robots, Bob and Alice, were thought the art of negotiation apples and books. They had been instructed to work out how to negotiate between themselves and improve their bartering as they went along. But, after leaving the pair alone, they start talking in this uncompressible but yet effective vocabulary.
According to researchers, what happened was normal as the chatbots were not instructed to stick to a fixed understandable English script, allowing them to create their shorthand. But eventually, the Facebook team decided to switch them off as humans cannot understand the two machines. The media blast, warning for a sinister Machine-controlled era. FAIR researchers, on the other hand, responded calling reporters "clickbaity and irresponsible."
Whether the case, CEMSE News asked Xiangliang Zhang, Professor of Computer Science (CS) and Director of the Machine Intelligence & kNowledge Engineering (MINE) Laboratory, to comment on this.
Can this example be considered intelligence?
From my point of view, this non-understandable conversation could be the faulty results of two machines that are not well trained. In Machine Learning it is widespread to have wrong outputs when the learning process is incomplete or insufficient. For example, a classifier cannot differentiate hand-written number 1 and 7 with 100% accuracy, (sometimes 1 is recognized as 7, vice versa) if the classifier is not well designed and trained. The chatbot conversations can be simulated by using Generative Adversarial Networks (a new system with two neural networks contesting with each other) and Reinforcement Learning (a widely used machine learning technique, e.g., used in AlphaGo) given a set of human conversation instances. It is common to see such non-understandable conversations generated by the model if not trained sufficiently. Therefore, there is nothing to be afraid of this "intelligence".
Does intelligence make a machine alive?
Artificial Intelligence (AI) attracts a lot of attention recently, as it is already impacting our modern world. It is a topic of hot debate mainly due to the fear that machines will become incredibly intelligent and surpass our own. The goal of AI is to give machines the ability to seem like they have human intelligence. The 'intelligence' machines have is the imitation of intelligent human behavior, and is gained by running programs human designed. In my opinion, the 'intelligence' machines have is forged on the human one, as it is us who design models and learning strategies for devices. We can make machines have life-long learning. However, they are still machines, not alive.
How can you imagine the future of AI?
We have seen the significant impact of AI in speech recognition, text processing, image recondition, machine vision, machine translation, self-driving cars, etc. With the development of AI technology, we will see its success in a broad area of applications, such as intelligent healthcare, robot assistants, smart transportation, smart houses and so on. In general, AI will make our life easier, safer and better in the future.
What are the scopes of your research?
AI is a vast area, including fields of machine learning, natural language processing, multi-agent systems, reasoning, planning, and scheduling. My research is relevant to machine learning and its applications in large-scale and streaming data. In the so-called learning process, machines learn from experience, which is presented as 'data'. In this era of big data, AI and machine learning are not limited by the lack of data availability and small sample sizes anymore. More data means meaningful experience and better-learned models. However, machine learning is often challenged by the large volume, the high complexity, and the noise of the collected data. My research in simple words is, first of all, to learn structured and concise representations from large-scale and complex data, so the learned representations reveal intrinsic properties or semantics that are hidden in data; and second of all, to train decision-making models based on new representations in various application problems, e.g., reporting network anomalies, detecting traffic pattern variations and managing virtual machines in cloud computing systems.
by Valentina De Vincenti