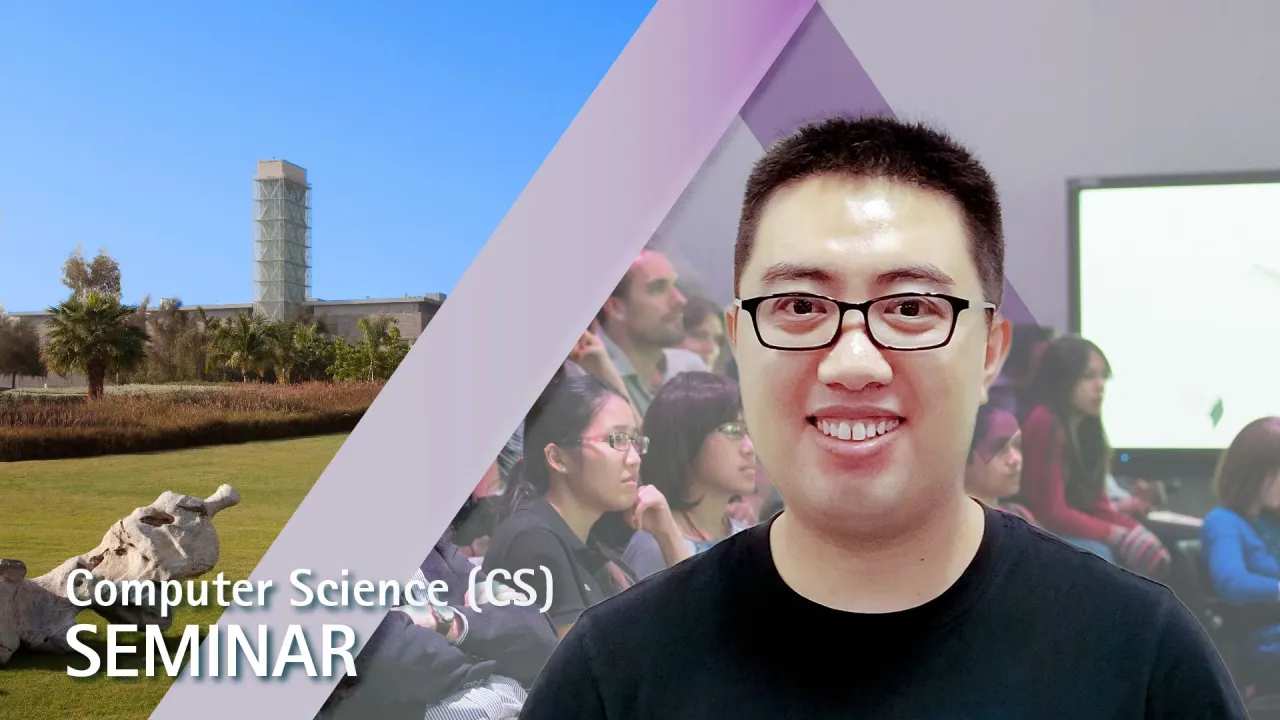
Deep Predictive Models for Mining Electronic Health Records
There is an increasing growth in the amount of electronic health records (EHRs) being collected by healthcare facilities. Data mining techniques hold great potential to systematically use such data for identifying not only inefficiencies but also best practices that improve care and reduce costs. However, due to the complexity of EHR data, directly applying traditional machine learning techniques may yield unsatisfactory predictive performance. Recent advances in deep learning-based methods provide unprecedented ability to predict patients’ future health status, but they still suffer from the sparsity issue of EHR data.
Overview
Light lunch will be available from 11:45AM.
Abstract
There is an increasing growth in the amount of electronic health records (EHRs) being collected by healthcare facilities. Data mining techniques hold great potential to systematically use such data for identifying not only inefficiencies but also best practices that improve care and reduce costs. However, due to the complexity of EHR data, directly applying traditional machine learning techniques may yield unsatisfactory predictive performance. Recent advances in deep learning-based methods provide unprecedented ability to predict patients’ future health status, but they still suffer from the sparsity issue of EHR data. Typically, EHR data only record the information regarding a few visits of a patient, which would be not enough to fully unleash the power of deep predictive models. In this talk, I will discuss my work on improving the predictive performance by incorporating doctors’ experience (i.e., prior medical knowledge) into the state-of-the-art deep learning based models. Besides, due to little prior medical knowledge and the limited number of patients on rare diseases, the sparsity issue in rare disease prediction is more severe and its prediction is more challenging. I will introduce my recent work on rare disease prediction by designing an effective system using deep generative models and reinforcement learning techniques.
Biography
Fenglong Ma is a final year Ph.D. candidate of the Department of Computer Science and Engineering at University at Buffalo, The State University of New York. He will join Pennsylvania State University from August 2019 as a tenure-track Assistant Professor. His research interests lie in data mining and machine learning, with an emphasis on mining health-related data. His research interests also include Crowdsourcing, Internet of Things, Social Network Mining and Security. He has published over 30 papers in top conferences and journals such as KDD, WWW, CIKM, WSDM, ICDM, SDM, BIBM, MobiCom, INFOCOM and TKDE. More information can be found at his website: http://www.acsu.buffalo.edu/~fenglong/.