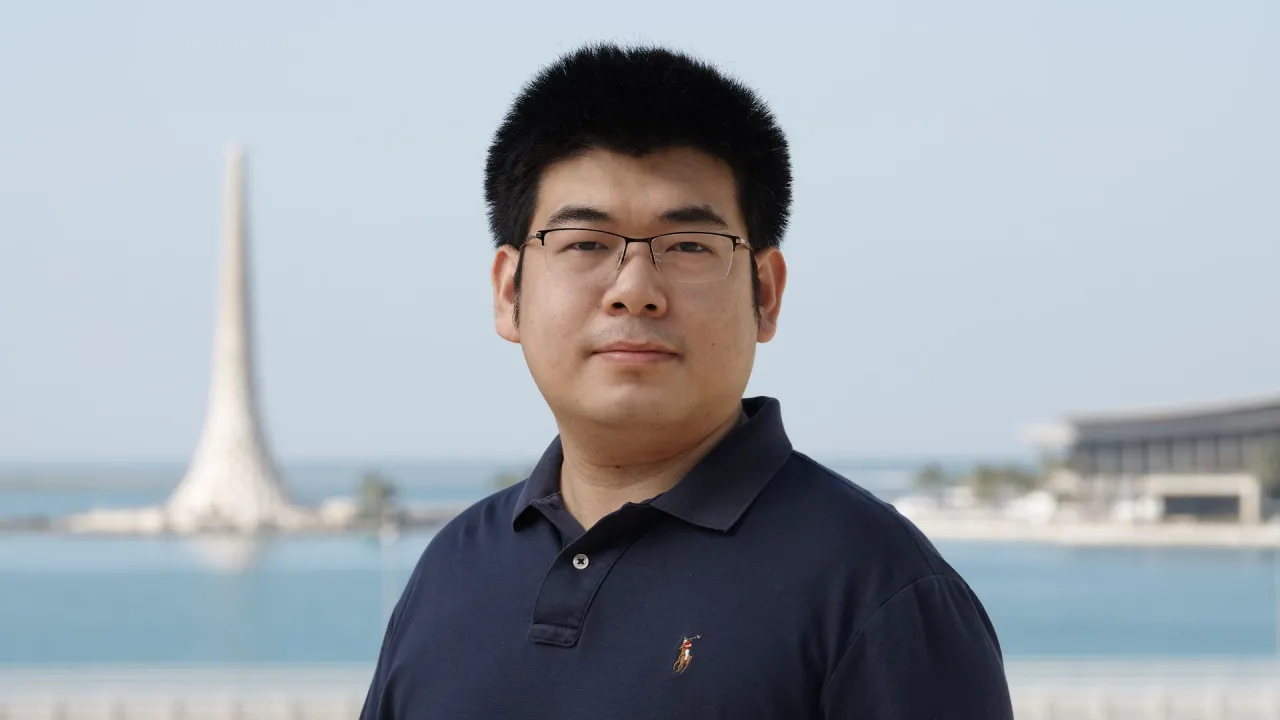
Di Wang
- Assistant Professor, Computer Science
- Principal Investigator, Privacy Awareness, Responsibility and Trustworthy Lab
Professor Wang's research addresses issues and societal concerns arising from machine learning, particularly in the areas of privacy, security, safety, fairness, robustness, interpretability and transparency. He aims to develop provable and practical algorithms to address trustworthiness issues in machine learning.
Biography
Di Wang is an assistant professor of Computer Science and the principal investigator of the KAUST Provable Responsible AI and Data Analytics (PRADA) Lab.
Before joining KAUST, he obtained his Ph.D. in Computer Science and Engineering ('20) from the State University of New York (SUNY) at Buffalo, U.S.; a M.S. in Mathematics ('15) from the University of Western Ontario, Canada; and a B.S. in Mathematics and Applied Mathematics ('14) from Shandong University, China.
Research Interests
Professor Wang’s research interests include machine learning (ML), security, theoretical computer science and data mining. His overall research focuses on solving issues and societal concerns arising from ML and data mining algorithms, such as privacy, fairness, robustness, transferability and transparency.
His PART team develops accurate learning algorithms that are equally private, fair, explainable and robust. These algorithms are supported by rigorous mathematical and cryptographic guarantees.
His research includes three perspectives: theory, practice and system. The theoretical component of his work provides rigorous mathematical guarantees for PART’s algorithms. The practical part develops trustworthy learning algorithms for biomedical, health care, genetic and social data, with a final focus on deploying trustworthy learning systems for healthcare and other applicable industries.
Education
- Doctor of Philosophy (Ph.D.)
- Computer Science and Engineering, The State University of New York, United States, 2020
- Master of Science (M.S.)
- Mathematics, University of Western Ontario, Canada, 2015
- Bachelor of Science (B.S.)
- Mathematics and Applied Mathematics, Shandong University, China, 2014
Selected Publications
- Lijie Hu, Shuo Ni, Hanshen Xiao and Di Wang. "High Dimensional Differentially Private Stochastic Optimization with Heavy-tailed Data". To appear in the 41st ACM Symposium on Principles of Database Systems (PODS 2022)
- Di Wang, Marco Gaboardi, Adam Smith, and Jinhui Xu. "Empirical Risk Minimization in the Non-interactive Local Model of Differential Privacy." Journal of machine learning research 21, no. 200 (2020).
- Di Wang, and Jinhui Xu. "On Sparse Linear Regression in the Local Differential Privacy Model." IEEE Transactions on Information Theory (2020).
- Di Wang, Hanshen Xiao, Srini Devadas, and Jinhui Xu. "On Differentially Private Stochastic Optimization with Heavy-tailed Data" In International Conference on Machine Learning. 2020. (ICML 2020)
- Yunus Esencayi, Marco Gaboardi, Shi Li, and Di Wang. "Facility Location Problem in Differential Privacy Model Revisited." Advances in neural information processing systems (2019). (NeurIPS 2019)
- Di Wang, Changyou Chen, and Jinhui Xu. "Differentially private empirical risk minimization with non-convex loss functions." In International Conference on Machine Learning, pp. 6526-6535. 2019. (ICML 2019)
- Di Wang, and Jinhui Xu. "On sparse linear regression in the local differential privacy model." In International Conference on Machine Learning, pp. 6628-6637. 2019. (ICML 2019)
- Di Wang, Marco Gaboardi, and Jinhui Xu. "Empirical risk minimization in non-interactive local differential privacy revisited." In Advances in Neural Information Processing Systems, pp. 965-974. 2018. (NeurIPS 2018)
- Di Wang, Minwei Ye, and Jinhui Xu. "Differentially private empirical risk minimization revisited: Faster and more general." In Advances in Neural Information Processing Systems, pp. 2722-2731. 2017. (NuerIPS 2017)