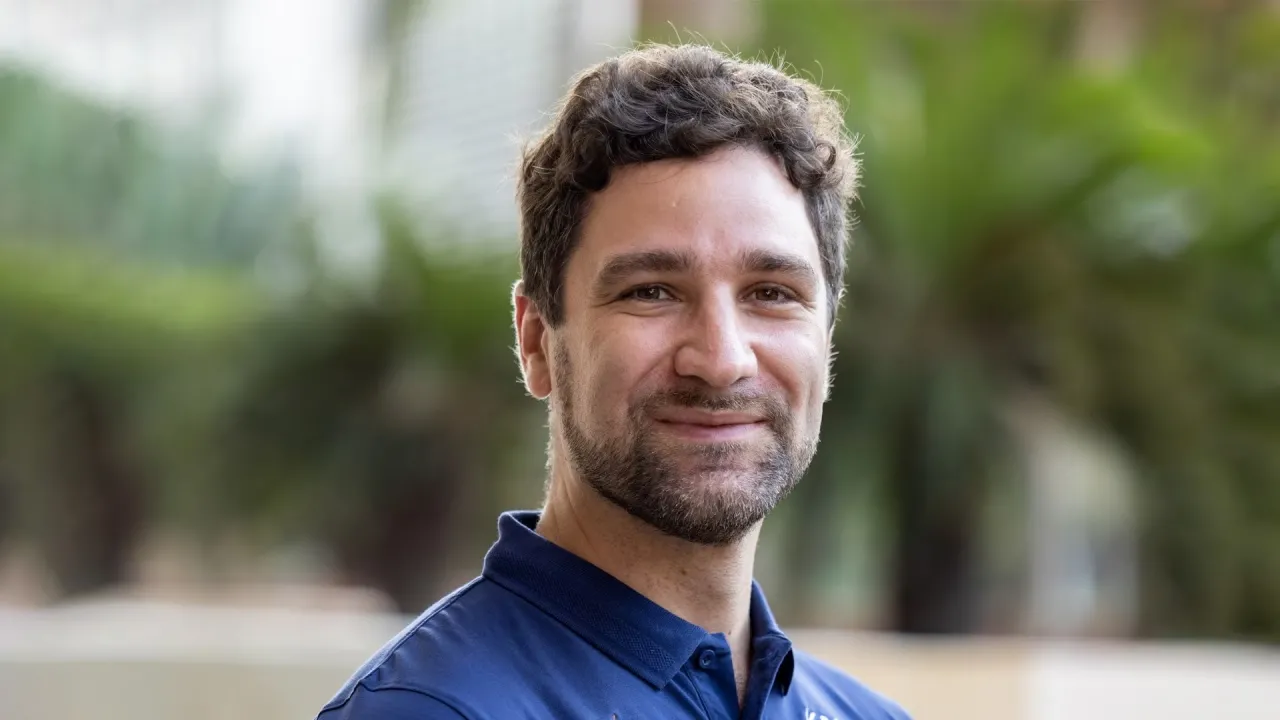
Raphaël Huser
- Associate Professor, Statistics
- Principal Investigator, Extreme Statistics
Professor Huser develops novel statistical methodology and machine learning solutions to model and predict extreme events in various applications ranging from climate and earth sciences to finance and neuroscience.
Biography
Raphaël Huser is an Associate Professor of Statistics and the principal investigator of the Extreme Statistics (XSTAT) research group. He is also affiliated with the Applied Mathematics and Computational Science (AMCS) Program.
Professor Huser received his Ph.D. in Statistics in 2013 from the Swiss Federal Institute of Technology, Switzerland, under the supervision of Professor Anthony C. Davison. He also holds a B.S. in Mathematics and an M.S. in Applied Mathematics from École polytechnique fédérale de Lausanne (EPFL), Switzerland.
After completing his Ph.D., Huser joined KAUST as a postdoctoral research fellow in January 2014. He was appointed Assistant Professor in March 2015 and promoted to Associate Professor of Statistics in 2022.
Research Interests
Raphaël Huser’s research primarily focuses on statistics of extreme events and risk assessment, including developing specialized statistical models with appealing statistical properties. Additionally, he studies efficient machine learning methods designed for massive datasets from complex spatio-temporal processes.
Huser’s novel methodological contributions are motivated and inspired by a wide variety of real data applications, which include the modeling of natural hazards in climate and earth sciences (e.g., heavy rainfall, heat waves, extreme sea surface temperatures, strong wind gusts, devastating landslides), the assessment of financial risk (e.g., turbulence in stock markets or cryptomarkets), and the characterization of brain signals during extreme stimuli (e.g., epileptic seizures).
Beyond creating new models with interesting statistical properties, one crucial aspect is fitting these complex models to big data. A critical area of Huser's current research is developing general-purpose, likelihood-free, fast and statistically efficient neural Bayes estimators. Being deeply anchored in statistical decision theory and relying on advanced deep-learning techniques, which makes them attractive both from a theoretical and a computational perspective, these estimators truly provide a paradigm shift challenging traditional statistical inference techniques for complex models with intractable likelihoods.
Huser, with collaborators, is contributing extensively to the early development of such estimators and their application to spatial (e.g., extreme) and multivariate models.
Awards and Distinctions
- Laureate of the Abdel El-Sharaawi Early Investigator Award, The Environmetrics Society (TIES), association of the International Statistical Institute (ISI), 2022
- Laureate of the ENVR Early Investigator Award, The Section on Statistics and the Environment (ENVR) of the American Statistical Association (ASA), 2019
- Best Paper Award, The International Biometric Conference (IBC) in Barcelona, Spain, 2018
- Elected Member of the International Statistical Institute (ISI), 2016
- Laureate of the Lambert Award for young statisticians, The Swiss Statistical Society (SSS), 2015
- Laureate of the EPFL Doctorate Award, The Swiss Federal Institute of Technology (EPFL), 2014
- 1st prize: Ph.D. poster competition at the Workshop on Environmetrics, NCAR, Boulder CO, US., 2010
- 2nd prize: M.S. project poster competition, Institute of Mathematics, Swiss Federal Institute of Technology (EPFL), 2009
- Publication Lombardo et al. (2018) highlighted among the top 10 most downloaded 2018 papers in Springer's Environmental Sciences Journals, Stochastic Environmental Research and Risk Assessment (SERRA) journal, 2019
- Award for Best 2016 Paper (Huser and Genton), Journal of Agricultural, Biological and Environmental Statistics (JABES), 2018
Education
- Doctor of Philosophy (Ph.D.)
- Statistics, Swiss Federal Institute of Technology Lausanne EPFL, Switzerland, 2013
- Master of Science (M.S.)
- Applied Mathematics, Swiss Federal Institute of Technology Lausanne EPFL, Switzerland, 2009
- Bachelor of Science (B.S.)
- Mathematics, Swiss Federal Institute of Technology Lausanne EPFL, Switzerland, 2007
Related Media
Quote
"Low-probability-high-impact events affect us, as a society, more than ever before. In the Extreme Statistics Research Group, we develop novel statistical methodology and machine learning methods to better model, infer, understand, and ultimately predict these extreme events in a wide range of applications ranging from climate and earth sciences, to finance, and neuroscience."
Research Achievements
Current:
- 2022-present: Associate Editor for Statistics and Computing
- 2022-present: Associate Editor for the Journal of the Royal Statistical Society: Series C (Applied Statistics)
- 2020-present: Associate Editor for the journal Environmetrics.
- 2019-present: Associate Editor for the Journal of Agricultural, Biological and Environmental Statistics (JABES).
- 2017-present: Associate Editor for the Extremes journal.
Past:
- 2020-2021: Associate Editor for the Journal of the Korean Statistical Society (JKSS)
- 2020: Guest Editor for the journal Extremes for the Special Issue on the "EVA 2019 Data Competition on Spatio-Temporal Prediction of Red Sea Surface Temperature Extremes"
- 2019-2021: Associate Editor for the Econometrics and Statistics (EcoSta) journal.
More details about editorial and reviewing activities on publons.
Questions and Answers
Why KAUST?
In 2014, I joined KAUST as a Postdoctoral Research Fellow. In 2015, I accepted a faculty position at KAUST (CEMSE Division), where I have been since that time (first, as an Assistant Professor, now as an Associate Professor) working on different aspects of statistics of extremes and spatio-temporal statistics. Beyond the extraordinary research environment at KAUST, I enjoy the diverse multicultural community, which is part of the DNA of KAUST and makes it so unique.
Why statistics of extremes?
I first got exposed to statistics of extremes when I was an undergraduate student in mathematics at the Swiss Federal Institute of Technology (EPFL), Lausanne, Switzerland. Since then, I have always been fascinated by the fact that there is a mathematically rigorous theory that precisely describes the probabilistic behavior of rare (i.e., low-probability) events, and that can be exploited in statistics to assess the risk of future, unprecedented extreme events, whose level may exceed our past experience. What I like about statistics of extremes is that it has both solid mathematical foundations, as well as a wide range of interesting applications in various fields, including Climate Science and Finance among others.