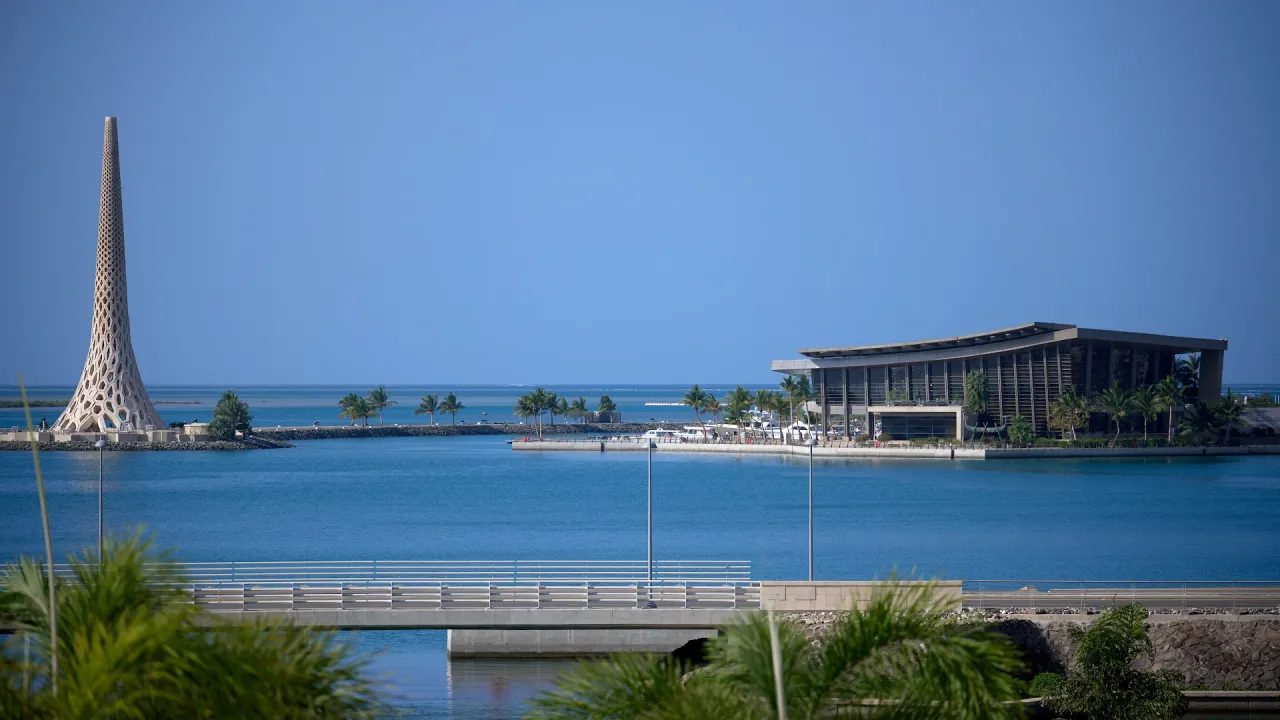
Challenging statistics of weather extremes
More accurate statistical modeling of extreme weather will improve forecasting and disaster mitigation.
About
By integrating previously distinct statistical paradigms into a single modeling scheme, Raphaël Huser from KAUST and Jennifer Wadsworth from Lancaster University in the UK have taken some of the guesswork out of modelling of weather extremes. This could greatly improve predictions of future extreme events.
Modeling the frequency and severity of possible weather extremes, such as intense rainfall, strong winds and heat waves, must account for nearby monitoring stations being spatially correlated. That is, heavy rain at one station often implies that there will be similarly heavy rain nearby.
However, as the severity of the event increases, this spatial dependence can weaken—the higher the rainfall intensity, for example, the less likely it is to occur across a wide region. Some extreme events may even be entirely localized around one station, with no correlation at all with those nearby.
Deciding whether the dependence changes with intensity, and to what extent, is a crucial step in the model selection process, but is often difficult to determine. For those involved in predicting weather disasters, a mismatch between model selection and the hidden character of the data can critically undermine the accuracy of predictions.
“It is very common with wind speeds or rainfall that spatial dependence weakens as events become more extreme, and eventually vanishes,” explains Huser. “If we restrict ourselves to ‘asymptotically’ dependent models, we might overestimate the spatial dependence strength of the largest extreme events; meanwhile, if we restrict ourselves to 'asymptotically' independent models, we might underestimate their dependence strength.”
Read the full article