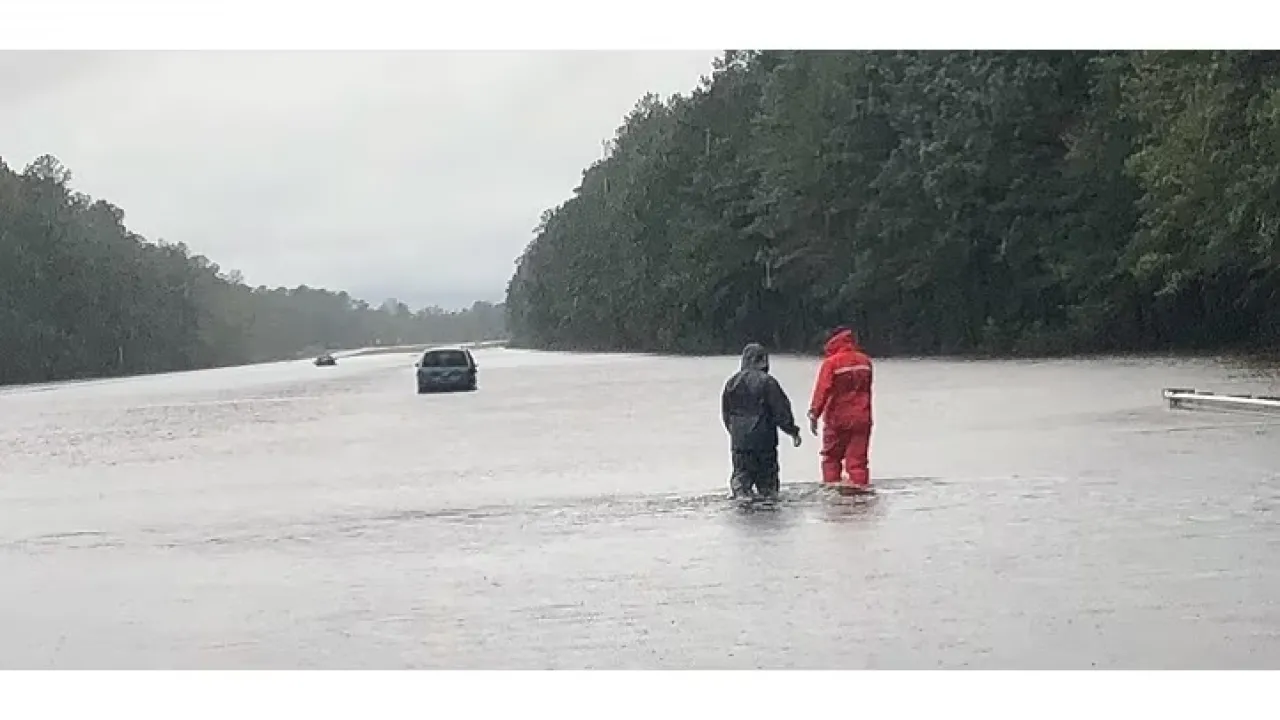
Expanding the scale of dangerous weather prediction
A more accurate and efficient method of capturing the local factors that lead to extreme rainfall enables better flood prediction across larger regions.
About
A statistical model that better characterizes the changing nature of extreme weather over larger areas could help climate experts plan for weather-related disasters.
An increase in the severity of extreme weather events around the world, such as droughts and floods, is creating a need for information that will help us better plan for these extreme events. Although vast volumes of weather data are recorded every day across the globe, extracting crucial information on extreme events puts huge demands on computing power and is limited to analysis across only a few locations.
Raphael Huser and his former postdoc Daniela Castro-Camilo have now developed a more computationally efficient statistical model to address these constraints.
“One of the main challenges in extreme weather statistics is to describe the relationship between extreme observations, such as rainfall changes across multiple locations,” explains Castro-Camilo. “Current models and methods can deal with only a limited number of locations at once and are not flexible enough to capture all the different dynamics we see in precipitation data.”
To accurately predict the frequency and magnitude of an extreme weather event across an area, Castro-Camilo and Huser focused on the dependence structure, which describes how strongly, and in what way, the data at several locations are related to one another.
“As events become more extreme, they also tend to become less dependent,” says Castro-Camilo. “This behavior is well recognized in climatological data, but classical extreme-value models are not able to describe this characteristic. Our model can do this.”
Read the full article