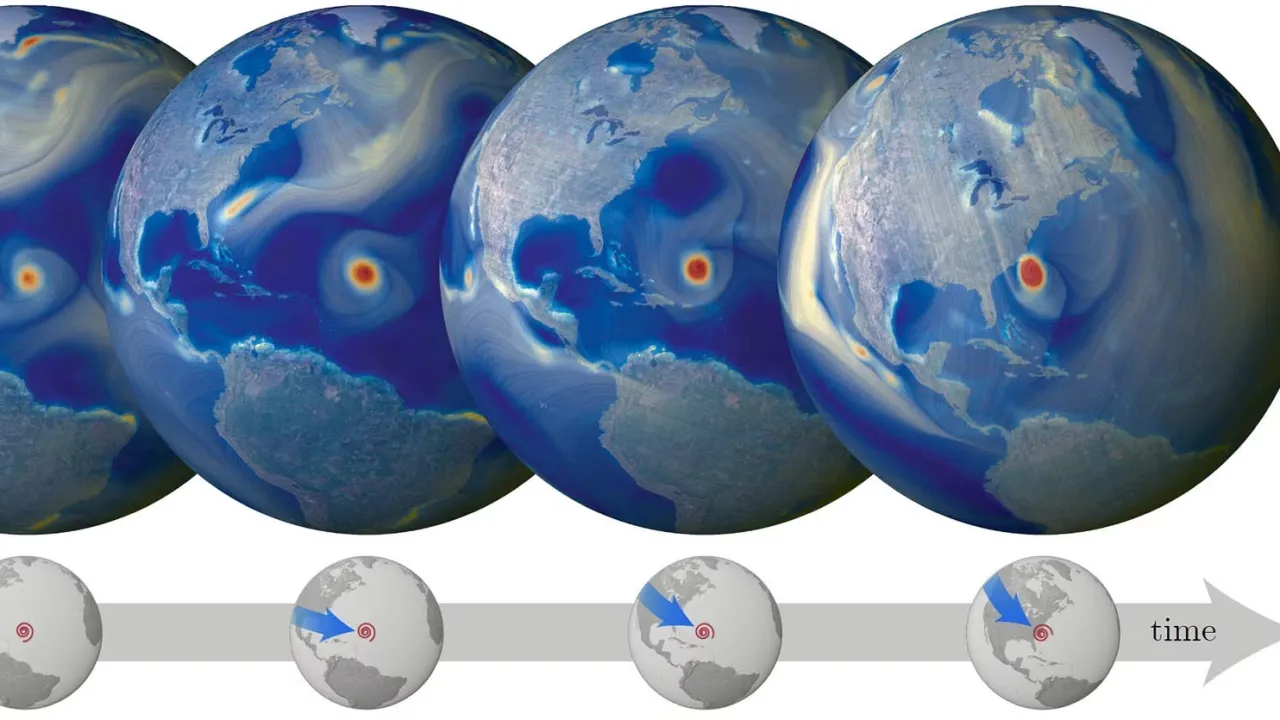
KAUST VCCVIS researchers win IEEE Scientific Visualization (SciVis) 2020 Best Paper Award
Researchers from the KAUST High-Performance Visualization Group (VCCVIS) have won the IEEE Scientific Visualization (SciVis) 2020 Best Paper Award at the IEEE Visualization Conference (IEEE VIS) 2020. Held virtually from October 25-30, IEEE VIS 2020 brought together a host of researchers and practitioners from academia, government, and industry to discuss advances in theory, methods and visual analytics applications.
About
By David Murphy
Researchers from the KAUST High-Performance Visualization Group (VCCVIS) have won the IEEE Scientific Visualization (SciVis) 2020 Best Paper Award at the IEEE Visualization Conference (IEEE VIS) 2020. Held virtually from October 25-30, IEEE VIS 2020 brought together a host of researchers and practitioners from academia, government, and industry to discuss advances in theory, methods and visual analytics applications.
The group’s winning paper titled, “Objective Observer-Relative Flow Visualization in Curved Spaces for Unsteady 2D Geophysical Flows,” was deemed a worthy winner based on its “potential impact to the community,” coupled with technical challenges addressed by its findings. The multi-authored paper is the result of a research collaboration between the KAUST VCCVIS Group, the KAUST Visualization Core Lab and the Harvard Visual Computing Group.
It details how physical phenomena in fluids have a drastically different appearance when viewed from the perspective of different moving observers; for example, the visualization of one phenomenon like a moving hurricane. A hurricane can look vastly different, depending on the position of the observer. An observer statically placed on the ground perceives the hurricane as moving, while an observer that moves with the storm perceives it to remain in place while the earth moves underneath.
“When we visualize and analyze a hurricane (a phenomenon that takes place over several days) in a short sequence, a moving observer can better communicate the phenomenon. A visual as well as computational analysis profits from this supposedly simple change of observer,” Peter Rautek, a research scientist in the VCCVIS and paper co-author, explained.
“We developed a method for the computation of a moving observer that optimally represents the phenomenon at hand and several methods that visualize the flow-fields relative to this observer. Our methods are based on differential geometry, allowing us to compute them on curved surfaces like the earth.
“It is a great honor to receive the SciVis award - and it also increases the visibility of our research and KAUST. And the more people know of KAUST as a place where excellent research happens, the easier it becomes for us to attract top talent in the future. The benefit of having your research put in the spotlight and made highly visible to the entire community is extremely satisfying,” he added.
Developing next-gen data-structures and visualization algorithms
The KAUST VCCVIS Group, which is led by Professor Markus Hadwiger, works on visualization challenges that arise from other domain sciences or in industry scenarios. The group develops novel visualization methods that support data exploration, analysis and communication. VCCVIS researchers focus on the visualization of extreme-scale data from a diverse set of domains. These include electron microscopy data from neuroscience, particle data from material science, as well as fluid flow data for climate research and reservoir simulations for the oil industry.
“In the age of ever-growing data acquisition rates and increasing complexity of data, we develop the next generation of data-structures, visualization algorithms, and software prototypes, that enable users to visualize their data interactively,” Rautek noted.
“At the moment, we are working on making our novel method more robust, more adaptive, more scalable, and faster. Further, using our observer-relative techniques, we are developing more computational methods that will be used to identify flow phenomena in the data automatically.
“And, moving forward, we want to apply this observer-relative technique to different kinds of flow phenomena that occur in disciplines like aerodynamics, oil reservoirs, and weather and climate simulation,” he concluded.