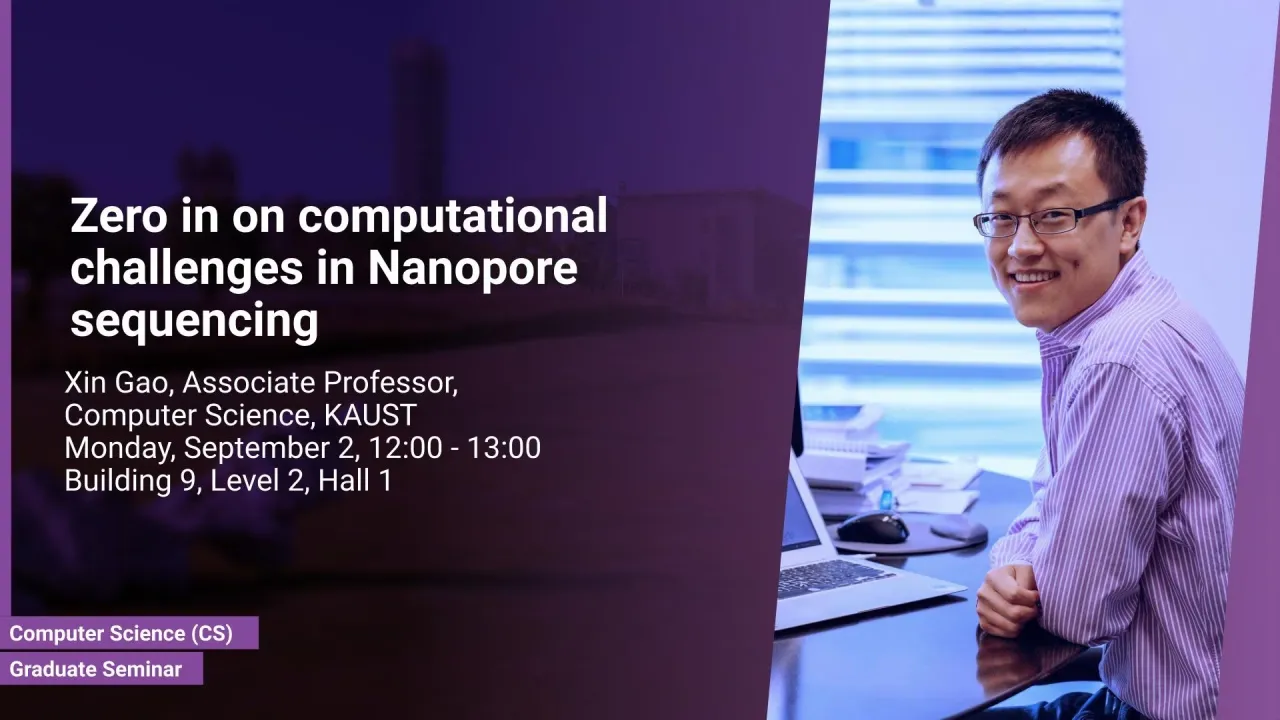
Zero in on computational challenges in Nanopore sequencing
In this talk, I will first give an overview of the research activities in Structural and Functional Bioinformatics Group (http://sfb.kaust.edu.sa). I will then focus on our efforts on developing computational methods to tackle key open problems in Nanopore sequencing. In particular, I will introduce our recent works on developing a collection of computational methods to decode raw electrical current signal sequences into DNA sequences, to simulate raw signals of Nanopore, and to efficiently and accurately align electrical current signal sequences with DNA sequences. Then, I will further introduce their applications in clinical and environmental fields.
Overview
Abstract
In this talk, I will first give an overview of the research activities in Structural and Functional Bioinformatics Group (http://sfb.kaust.edu.sa). I will then focus on our efforts on developing computational methods to tackle key open problems in Nanopore sequencing.
Oxford Nanopore sequencing is a rapidly developed sequencing technology in recent years. It owns the advantages of long-reads, point of care, and PCR-free. However, Nanopore data analysis poses great challenges to the computational community. I will introduce our recent works on developing a collection of computational methods to decode raw electrical current signal sequences into DNA sequences, to simulate raw signals of Nanopore, and to efficiently and accurately align electrical current signal sequences with DNA sequences. I will further introduce their applications in clinical and environmental fields.
Brief Biography
Dr. Xin Gao is an associate professor of computer science in the CEMSE Division at KAUST. He is also a PI in CBRC at KAUST. Prior to joining KAUST, he was a Lane Fellow at Lane Center for Computational Biology in School of Computer Science at Carnegie Mellon University, U.S.. He earned his bachelor degree in Computer Science in 2004 from Computer Science and Technology Department at Tsinghua University, China, and his Ph.D. degree in Computer Science in 2009 from David R. Cheriton School of Computer Science at University of Waterloo, Canada.
Dr. Gao’s research interest lies at the intersection between computer science and biology. In the field of computer science, he is interested in developing machine learning theories and methodologies. In the field of bioinformatics, his group works on building computational models, developing machine learning techniques, and designing efficient and effective algorithms, to tackle key open problems along the path from biological sequence analysis, to 3D structure determination, to function annotation, to understanding and controlling molecular behaviors in complex biological networks, and to biomedicine and healthcare. He has published more than 170 research articles in the fields of bioinformatics and machine learning.