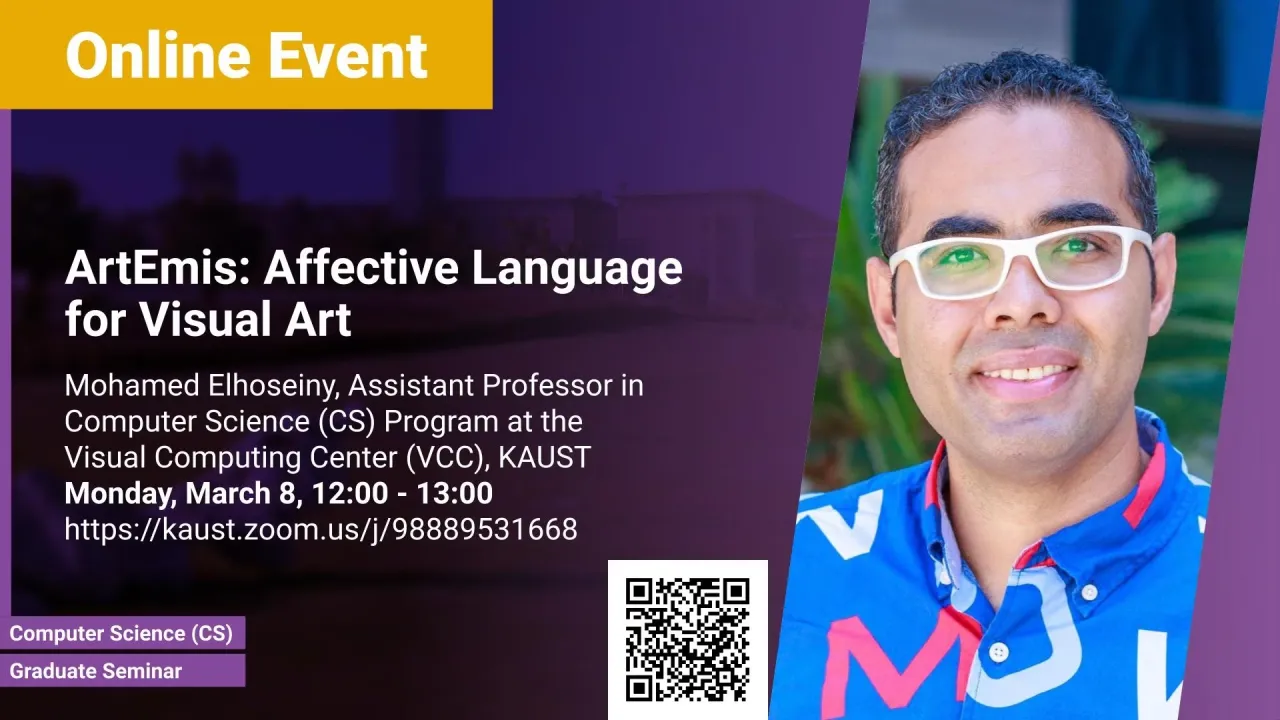
ArtEmis: Affective Language for Visual Art
We present a novel large-scale dataset and accompanying machine learning models aimed at providing a detailed understanding of the interplay between visual content, its emotional effect, and explanations for the latter in language. In contrast to most existing annotation datasets in computer vision, we focus on the affective experience triggered by visual artworks and ask the annotators to indicate the dominant emotion they feel for a given image and, crucially, to also provide a grounded verbal explanation for their emotion choice.
Overview
Abstract
We present a novel large-scale dataset and accompanying machine learning models aimed at providing a detailed understanding of the interplay between visual content, its emotional effect, and explanations for the latter in language. In contrast to most existing annotation datasets in computer vision, we focus on the affective experience triggered by visual artworks and ask the annotators to indicate the dominant emotion they feel for a given image and, crucially, to also provide a grounded verbal explanation for their emotion choice. As we demonstrate below, this leads to a rich set of signals for both the objective content and the affective impact of an image, creating associations with abstract concepts (e.g., "freedom" or "love"), or references that go beyond what is directly visible, including visual similes and metaphors, or subjective references to personal experiences. We focus on visual art (e.g., paintings, artistic photographs) as it is a prime example of imagery created to elicit emotional responses from its viewers. Our dataset, termed ArtEmis, contains 439K emotion attributions and explanations from humans, on 81K artworks from WikiArt. Building on this data, we train and demonstrate a series of captioning systems capable of expressing and explaining emotions from visual stimuli. Remarkably, the captions produced by these systems often succeed in reflecting the semantic and abstract content of the image, going well beyond systems trained on existing datasets. The collected dataset and developed methods are available at this URL. http://artemisdataset.org/
Brief Biography
Mohamed Elhoseiny is an assistant professor of Computer Science at KAUST and the director of the Vision-CAIR group. Previously, he was a visiting Faculty at Stanford Computer Science department (2019-2020), Visiting Faculty at Baidu Research (2019), Postdoc researcher at Facebook AI Research (2016-2019). Dr. Elhoseiny did his Ph.D. in 2016 at Rutgers University where also he spent time at SRI International in 2014 and at Adobe Research (2015-2016). His primary research interest is in computer vision and especially in efficient multimodal learning with limited data in zero/few-shot learning and Vision & Language. He is also interested in Affective AI and especially to understand and generate novel visual content (e.g., art and fashion). He received an NSF Fellowship in 2014 and the Doctoral Consortium award at CVPR’16. His zero-shot learning work was featured at the United Nations and his creative AI work was featured in MIT Tech Review, New Scientist Magazine, and HBO Silicon Valley. He is serving as Area Chair at CVPR21 and ICCV21 and has organized workshops at ICCV’15, ICCV’17, ICCV’19, and CVPR'21.
Visit https://cemse.kaust.edu.sa/vision-cair and http://www.mohamed-elhoseiny.com for more details.