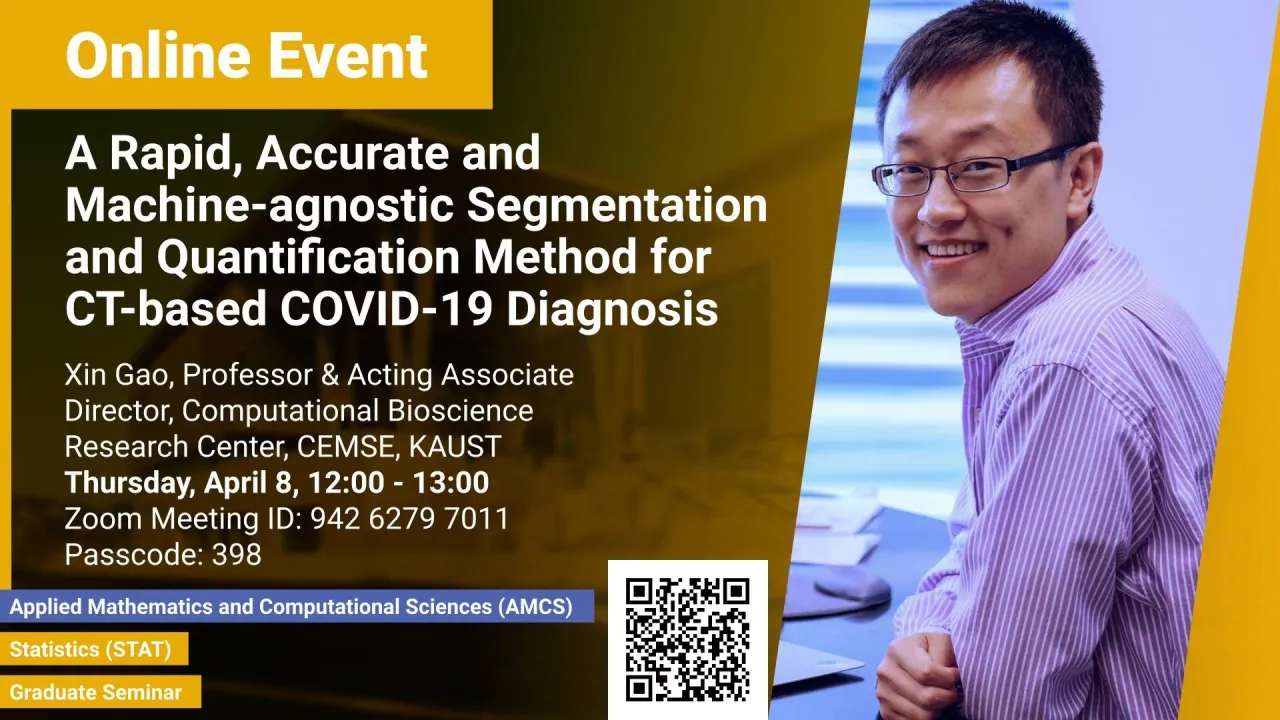
A Rapid, Accurate and Machine-agnostic Segmentation and Quantification Method for CT-based COVID-19 Diagnosis
COVID-19 has caused a global pandemic and become the most urgent threat to the entire world. Tremendous efforts and resources have been invested in developing diagnosis. Despite the various, urgent advances in developing artificial intelligence (AI)-based computer-aided systems for CT-based COVID-19 diagnosis, most of the existing methods can only perform classification, whereas the state-of-the-art segmentation method requires a high level of human intervention. In this talk, I will introduce our recent work on a fully-automatic, rapid, accurate, and machine-agnostic method that can segment and quantify the infection regions on CT scans from different sources. Our method is founded upon three innovations: 1) an embedding method that projects any arbitrary CT scan to a same, standard space, so that the trained model becomes robust and generalizable; 2) the first CT scan simulator for COVID-19, by fitting the dynamic change of real patients’ data measured at different time points, which greatly alleviates the data scarcity issue; and 3) a novel deep learning algorithm to solve the large-scene-small-object problem, which decomposes the 3D segmentation problem into three 2D ones, and thus reduces the model complexity by an order of magnitude and, at the same time, significantly improves the segmentation accuracy. Comprehensive experimental results over multi-country, multi-hospital, and multi-machine datasets demonstrate the superior performance of our method over the existing ones and suggest its important application value in combating the disease.
Overview
Abstract
COVID-19 has caused a global pandemic and become the most urgent threat to the entire world. Tremendous efforts and resources have been invested in developing diagnosis.
Despite the various, urgent advances in developing artificial intelligence (AI)-based computer-aided systems for CT-based COVID-19 diagnosis, most of the existing methods can only perform classification, whereas the state-of-the-art segmentation method requires a high level of human intervention. In this talk, I will introduce our recent work on a fully-automatic, rapid, accurate, and machine-agnostic method that can segment and quantify the infection regions on CT scans from different sources. Our method is founded upon three innovations: 1) an embedding method that projects any arbitrary CT scan to a same, standard space, so that the trained model becomes robust and generalizable; 2) the first CT scan simulator for COVID-19, by fitting the dynamic change of real patients’ data measured at different time points, which greatly alleviates the data scarcity issue; and 3) a novel deep learning algorithm to solve the large-scene-small-object problem, which decomposes the 3D segmentation problem into three 2D ones, and thus reduces the model complexity by an order of magnitude and, at the same time, significantly improves the segmentation accuracy. Comprehensive experimental results over multi-country, multi-hospital, and multi-machine datasets demonstrate the superior performance of our method over the existing ones and suggest its important application value in combating the disease.
Brief Biography
Dr. Xin Gao is a professor of computer science in CEMSE Division at KAUST. He is also the Acting Associate Director of the Computational Bioscience Research Center (CBRC), Deputy Director of the Smart Health Initiative (SHI), and the Lead of the Structural and Functional Bioinformatics (SFB) Group at KAUST. Prior to joining KAUST, he was a Lane Fellow at Lane Center for Computational Biology in School of Computer Science at Carnegie Mellon University. He earned his bachelor degree in Computer Science in 2004 from Tsinghua University and his Ph.D. degree in Computer Science in 2009 from University of Waterloo.
Dr. Gao’s research interest lies at the intersection between computer science and biology. In the field of computer science, he is interested in developing machine learning theories and methodologies related to deep learning, probabilistic graphical models, kernel methods and matrix factorization. In the field of bioinformatics, his group works on building computational models, developing machine learning techniques, and designing efficient and effective algorithms to tackle key open problems along the path from biological sequence analysis, to 3D structure determination, to function annotation, to understanding and controlling molecular behaviors in complex biological networks, and, recently, to biomedicine and healthcare.
He has published more than 240 papers in the fields of bioinformatics and machine learning. He is the associate editor of Genomics, Proteomics & Bioinformatics, BMC Bioinformatics, Journal of Bioinformatics and Computational Biology, and Quantitative Biology, and the guest editor-in-chief of IEEE/ACM Transactions on Computational Biology and Bioinformatics, Methods, and Frontiers in Molecular Bioscience.