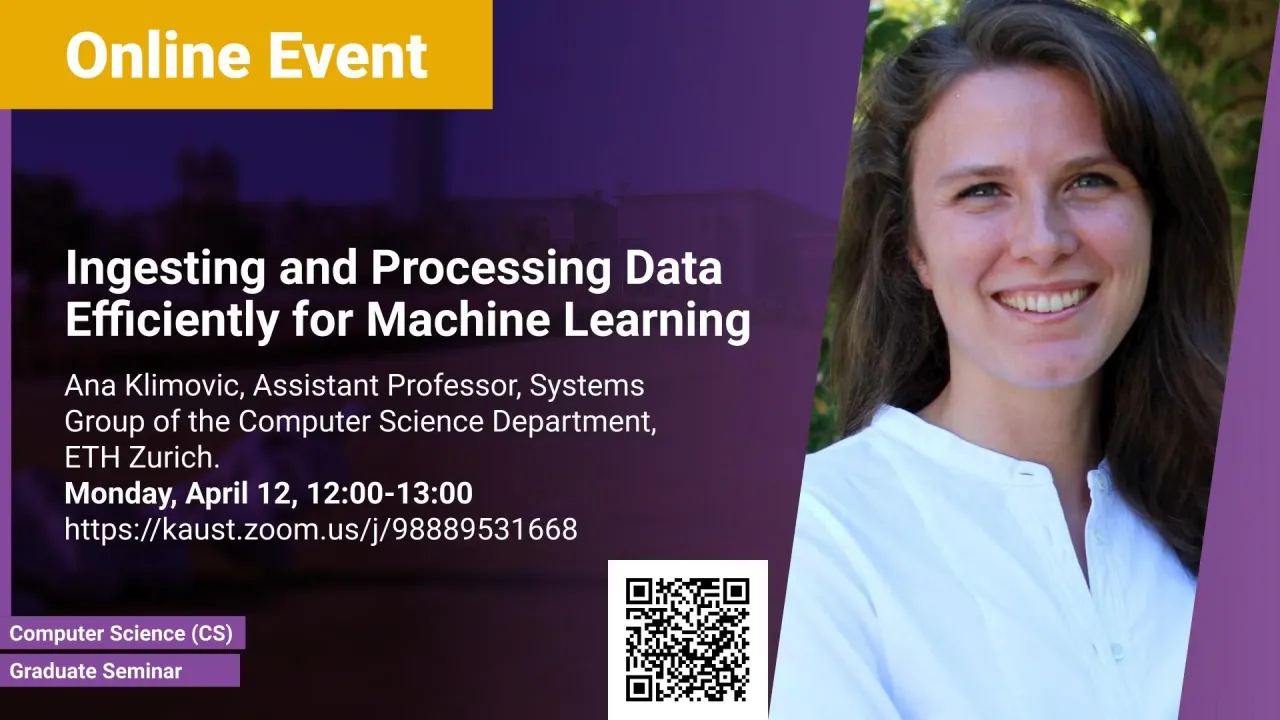
Ingesting and Processing Data Efficiently for Machine Learning
- Ana Klimovic, Assistant Professor, Systems Group of the Computer Science Department, ETH Zurich.
KAUST
Machine learning applications have sparked the development of specialized software frameworks and hardware accelerators. Yet, in today’s machine learning ecosystem, one important part of the system stack has received far less attention and specialization for ML: how we store and preprocess training data. This talk will describe the key challenges for implementing high-performance ML input data processing pipelines.
Overview
Abstract
Machine learning applications have sparked the development of specialized software frameworks and hardware accelerators. Yet, in today’s machine learning ecosystem, one important part of the system stack has received far less attention and specialization for ML: how we store and preprocess training data. This talk will describe the key challenges for implementing high-performance ML input data processing pipelines. We analyze millions of ML jobs running in Google's fleet and find that input pipeline performance significantly impacts end-to-end training performance and resource consumption. Our study shows that ingesting and preprocessing data on-the-fly during training consumes 30% of end-to-end training time, on average. Our characterization of input data pipelines motivates several systems research directions, such as disaggregating input data processing from model training and caching commonly reoccurring input data computation subgraphs. We present the multi-tenant input data processing service that we are building at ETH Zurich, in collaboration with Google, to improve ML training performance and resource usage.
Brief Biography
Ana Klimovic is an Assistant Professor in the Systems Group of the Computer Science Department at ETH Zurich. Her research interests span operating systems, computer architecture, and their intersection with machine learning. Ana's work focuses on computer system design for large-scale applications such as cloud computing services, data analytics, and machine learning. Before joining ETH in August 2020, Ana was a Research Scientist at Google Brain and completed her Ph.D. in Electrical Engineering at Stanford University in 2019. Her dissertation research was on the design and implementation of fast, elastic storage for cloud computing.