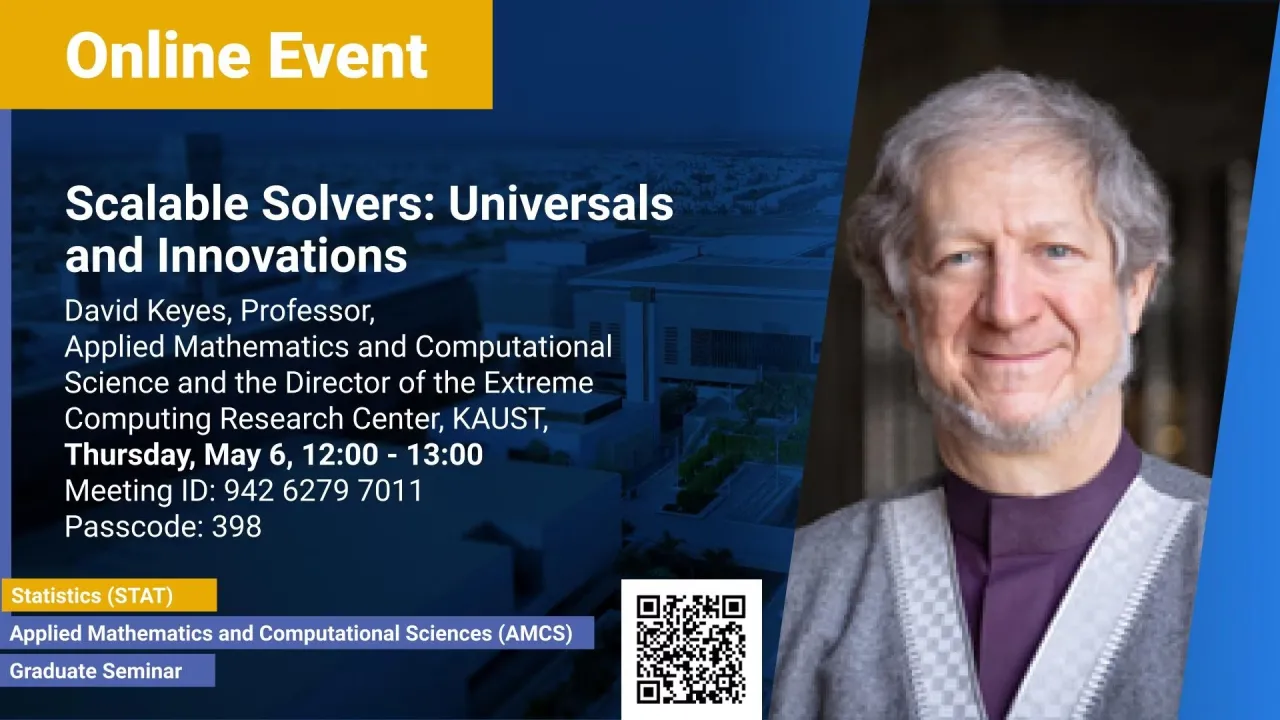
Scalable Solvers: Universals and Innovations
Overview
Abstract
As simulation and analytics enter the exascale era, numerical algorithms must span a widening gap between ambitious applications and austere architectures. We present fifteen universals for researchers in scalable solvers and some innovations that allow approaching lin-log complexity in storage and operation count in many important algorithmic kernels.
Applications are becoming ambitious in many senses: large physical space, phase space or parameter dimensions; resolution of many scales of space and/or time; high fidelity physical modeling; linking together of multiple complex models; placement of “forward problems” inside outer loops for inversion, assimilation, optimization, control, or machine learning; quantification of uncertainty; improvement of statistical estimates; and dynamic adaptation.
Architectures are austere in several senses: chiefly low memory per core, low memory bandwidth per core, and low power per operation. Low power implies using lower numerical precision where possible and many slower cores rather than fewer faster cores. It also puts a premium on building resilience into software rather than into fully reliable hardware, since hardware resilience is usually achieved through time or resource redundancy.
Algorithms must adapt to span this gap especially by means that lead to less uniformity and less predetermined schedulability of operations. Billions of dollars worth of open-source scientific software worldwide predicated upon single-program multiple data (SPMD) message-passing programming, representing 30 years of successful performance advances (as a measure, for instance, by the Gordon Bell Prizes), hangs in the balance. Without such algorithmic adaption, exascale computers are limited to petascale performance. “
Brief Biography
David Keyes is Professor of Applied Mathematics and Computational Science and the Director of the Extreme Computing Research Center, having served as the Dean of the Division of Mathematical and Computer Sciences and Engineering at KAUST for its first 3.5 years. Also an Adjunct Professor and former Fu Foundation Chair Professor in Applied Physics and Applied Mathematics at Columbia University, and an affiliate of several laboratories of the U.S. Department of Energy, Keyes graduated in Aerospace and Mechanical Sciences from Princeton in 1978 and earned a doctorate in Applied Mathematics from Harvard in 1984. Before joining KAUST among the founding faculty, he led scalable solver software projects in the ASCI and SciDAC programs of the U.S. Department of Energy.
Keyes works at the algorithmic interface between parallel computing and the numerical analysis of partial differential equations, with a focus on implicit scalable solvers for emerging architectures and their use in the many large-scale applications in energy and environment governed by conservation laws that demand high performance because of high resolution, high dimension, high fidelity physical models, or the "multi-solve" requirements of optimization, control, sensitivity analysis, inverse problems, data assimilation, or uncertainty quantification.
He has named and contributed to Newton-Krylov-Schwarz (NKS), Additive Schwarz Preconditioned Inexact Newton (ASPIN), and Algebraic Fast Multipole (AFM) methods for large sparse linear and nonlinear systems arising from PDEs. Through the ECRC, he now works on meeting the requirements of drastic reductions in communication and synchronization, increases in concurrency for cores sharing memory locally, local load redistribution, and algorithm-based fault tolerance for these and other algorithms.