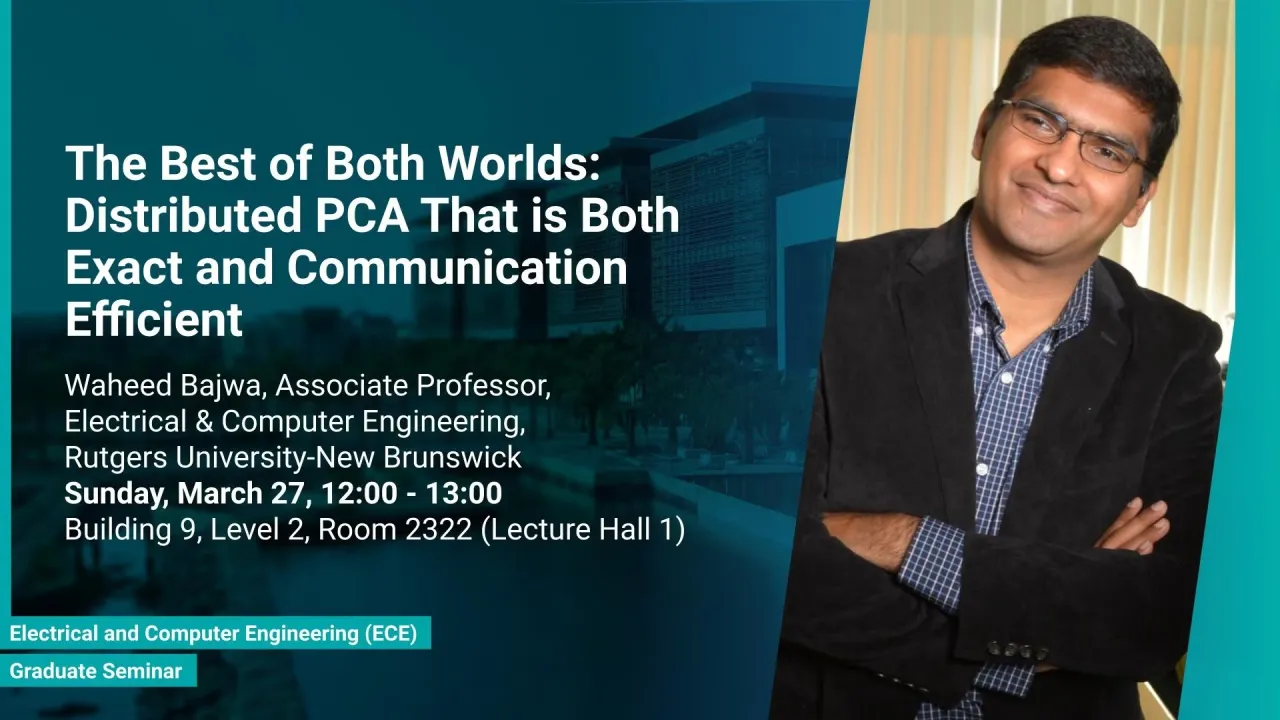
The Best of Both Worlds: Distributed PCA That is Both Exact and Communication Efficient
- Prof. Waheed Bajwa, Electrical and Computer Engineering, Rutgers University-New Brunswick
B9 L2 R2322 H1
Principal Component Analysis (PCA) is a fundamental data preprocessing tool in the world of machine learning.
Overview
Abstract
Principal Component Analysis (PCA) is a fundamental data preprocessing tool in the world of machine learning. While PCA is often thought of as a dimensionality reduction method, the purpose of PCA is actually two-fold: dimension reduction and uncorrelated feature learning. Unfortunately, the enormity of the dimensions and sample size in the modern day datasets have rendered the centralized PCA solutions unusable. In that vein, this talk reconsiders the problem of PCA when data samples are distributed across nodes in an arbitrarily connected network. While a few solutions for distributed PCA exist, those either overlook the uncorrelated feature learning aspect of the PCA, tend to have high communication overhead that makes them inefficient and/or lack 'exact' or 'global' convergence guarantees. To overcome these aforementioned issues, we introduce in this talk a new distributed PCA algorithm, termed FAST-PCA (Fast and exAct diSTributed PCA), that is both efficient in terms of communication and proven to converge linearly and exactly to the principal components, leading to dimension reduction as well as uncorrelated features.
Brief Biography
Waheed U. Bajwa, whose research interests include statistical signal processing, high-dimensional statistics, machine learning, inverse problems, and networked systems, is currently a visiting fellow in the Center for Statistics and Machine Learning at Princeton University, and an associate professor in the Department of Electrical and Computer Engineering and an associate member of the graduate faculty of the Department of Statistics at Rutgers University.
Dr. Bajwa has received several research and teaching awards including the Army Research Office Young Investigator Award (2014), the National Science Foundation CAREER Award (2015), Rutgers University’s Presidential Merit Award (2016), Rutgers University’s Presidential Fellowship for Teaching Excellence (2017), Rutgers University’s Engineering Governing Council ECE Professor of the Year Award (2016, 2017, 2019), and Rutgers University’s Warren I. Susman Award for Excellence in Teaching (2021). He is a co-investigator on a work that received the Cancer Institute of New Jersey’s Gallo Award for Scientific Excellence in 2017, a co-author on papers that received Best Student Paper Awards at IEEE IVMSP 2016 and IEEE CAMSAP 2017 workshops, and a Member of the Class of 2015 National Academy of Engineering Frontiers of Engineering Education Symposium.