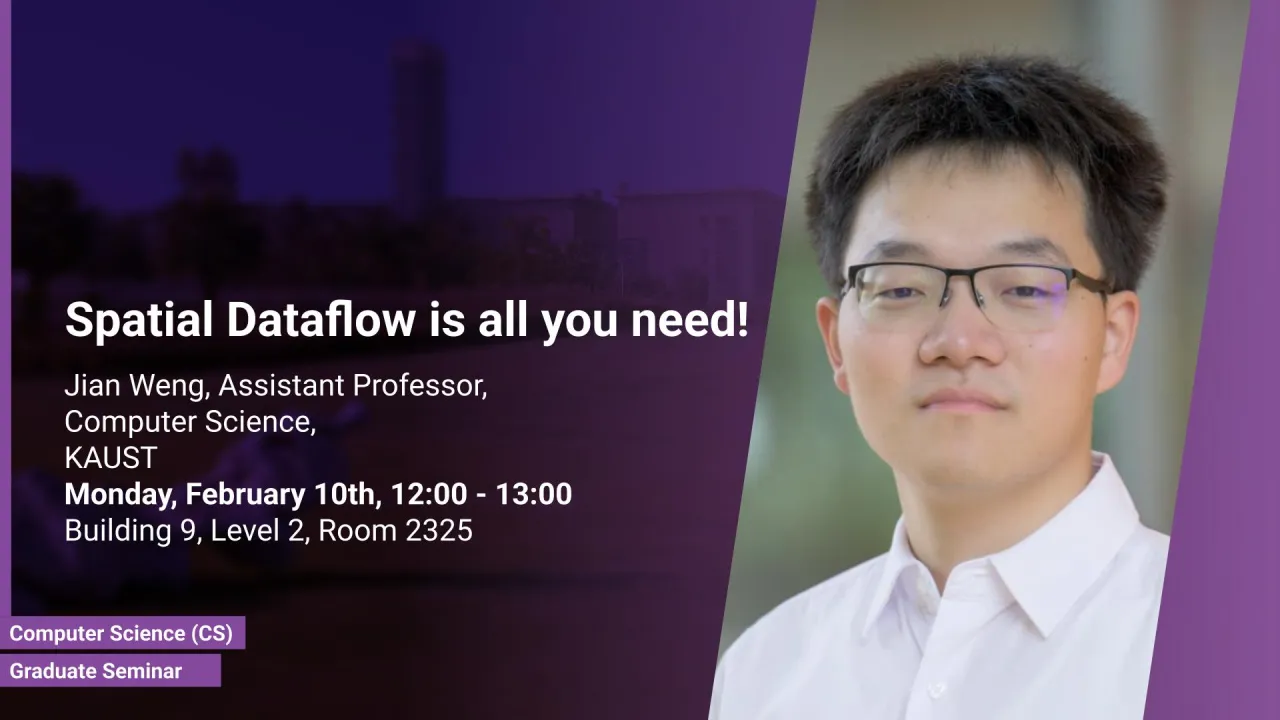
Spatial Dataflow is all you need!
Jian Weng's research, inspired by spatial dataflow, proposes a unified hardware design and implementation framework that leverages a familiar software programming paradigm, enabling concise and efficient designs while significantly reducing code complexity and achieving near-handcrafted performance, with future applications in ML inference systems targeting memory bandwidth limitations.
Overview
Transistor scaling not only offers more and more on-chip resources to improve the chip performance, but also urges system developers to design and implement better computer architectures to better utilize these resources. However, existing architectural design and implementation approaches still rely on separate codebases for both simulation-based design decision modeling, and RTL implementation. Jian Weng’s research view these technical challenges in a view of spatial dataflow, and proposes a brand-new abstraction for unified design and implementation. By taking advantage of a widely existing programming paradigm in software engineering, a concise, expressive, and efficient hardware design and implementation framework is proposed. This approach achieves near handcraft performances, while saving 64% LoC. Moreover, Jian Weng is going to apply the spatial dataflow methodology on a next-generation ML inference system by taking advantage of the larger and larger on-chip memory to amortize the memory bottleneck.
Presenters
Brief Biography
Jian Weng is an assistant professor of computer science at KAUST. Professor Weng joined KAUST from the University of California, Los Angeles (UCLA), U.S., where he completed his Ph.D. in Computer Science in 2023, advised by Professor Tony Nowatzki. He received a Bachelor of Engineering from Shanghai Jiao Tong University, China, in 2017.
Weng’s work has been recognized with an IEEE Micro Top Picks Honorable Mention, and an IEEE/ACM International Symposium on Microarchitecture (MICRO) Best Paper Runner-Up Award.