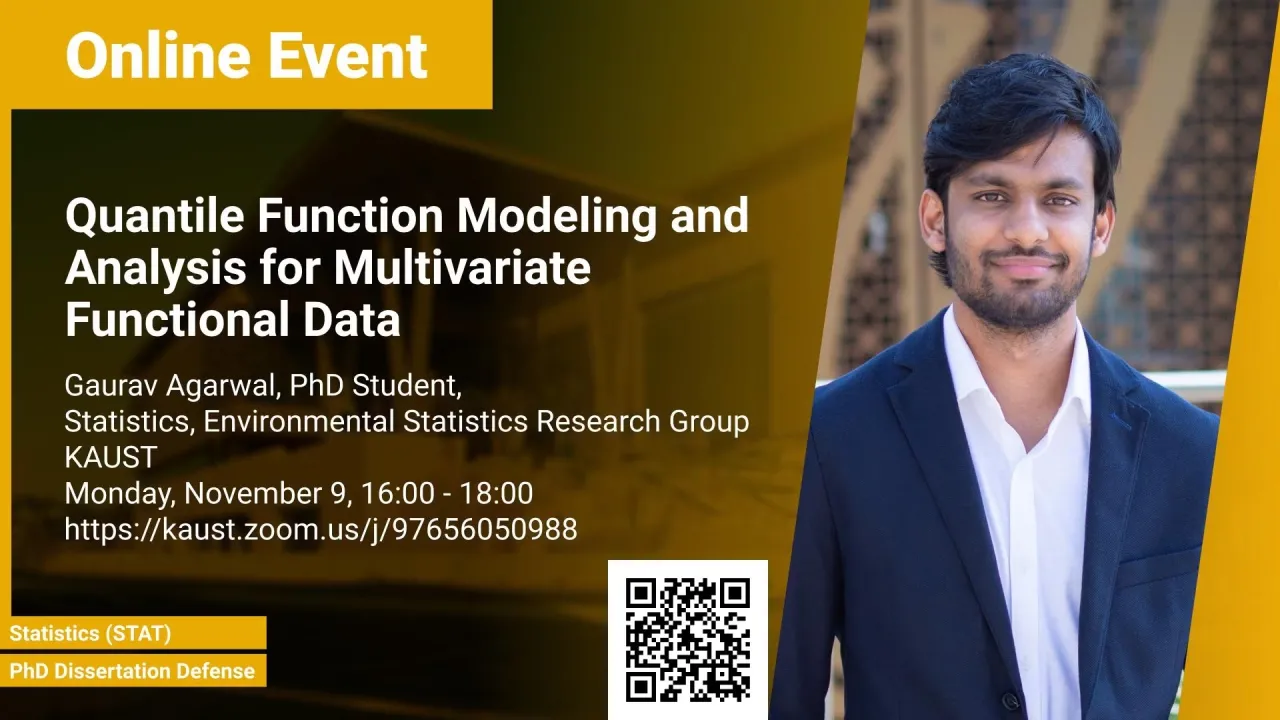
Quantile Function Modeling and Analysis for Multivariate Functional Data
This thesis presents a set of quantile analysis methods for multivariate data and multivariate functional data, with an emphasis on environmental applications, and consists of four significant contributions.
Overview
Abstract
Quantile function modeling is a more robust, comprehensive, and flexible method of statistical analysis than the commonly used mean-based methods. More and more data are collected in the form of multivariate, functional, and multivariate functional data, for which many aspects of quantile analysis remain unexplored and challenging. This thesis presents a set of quantile analysis methods for multivariate data and multivariate functional data, with an emphasis on environmental applications, and consists of four significant contributions. Firstly, it proposes bivariate quantile analysis methods that can predict the joint distribution of bivariate response and improve on conventional univariate quantile regression. The proposed robust statistical techniques are applied to examine barley plants grown in saltwater and freshwater conditions providing interesting insights into barley's responses, informing future crop decisions. Secondly, it proposes modeling and visualization of bivariate functional data to characterize the distribution and detect outliers. The proposed methods provide an informative visualization tool for bivariate functional data and can characterize non-Gaussian, skewed, and heavy-tailed distributions using directional quantile envelopes. The radiosonde wind data application illustrates our proposed quantile analysis methods for visualization, outlier detection, and prediction. However, the directional quantile envelopes are convex by definition. This feature is shared by most existing methods, which is not desirable in nonconvex and multimodal distributions. Thirdly, this challenge is addressed by modeling multivariate functional data for flexible quantile contour estimation and prediction. The estimated contours are flexible in the sense that they can characterize non-Gaussian and nonconvex marginal distributions. The proposed multivariate quantile function enjoys the theoretical properties of monotonicity, uniqueness, and the consistency of its contours. The proposed methods are applied to air pollution data. Finally, we perform quantile spatial prediction for non-Gaussian spatial data, which often emerges in environmental applications. We introduce a copula-based multiple indicator kriging model, which makes no distributional assumptions on the marginal distribution, thus offers more flexibility. The method performs better than the commonly used variogram approach and Gaussian kriging for spatial prediction in simulations and application to precipitation data.
Brief Biography
Gaurav Agarwal is a Ph.D. candidate supervised by Prof. Ying Sun in the Statistics Program at King Abdullah University of Science and Technology. He received his Master in Statistics from the Indian Institute of Technology, Kanpur in 2016, and his Bachelor in Statistics from the Hindu College, University of Delhi in 2014. His research interests include robust statistics, quantile regression, functional data analysis, nonparametric statistics, and spatial statistics.