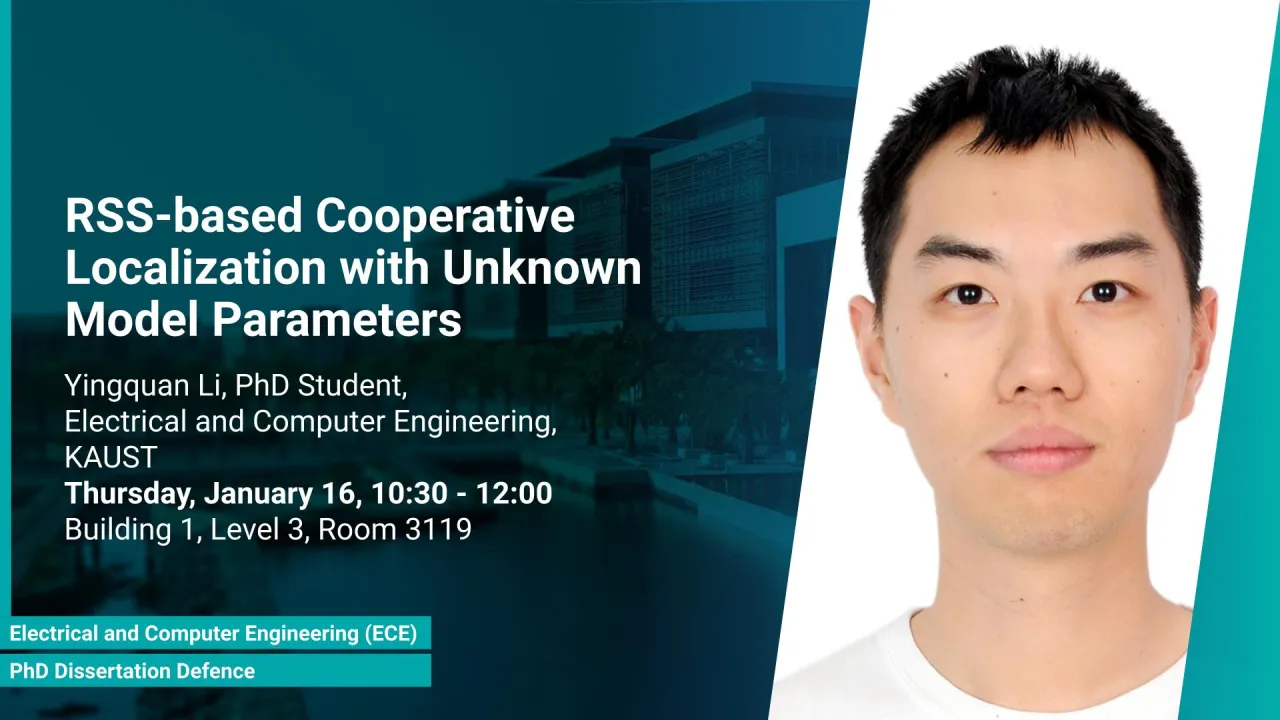
RSS-based Cooperative Localization with Unknown Model Parameters
B1, L3, R3119
The Internet of Things (IoT) has become a popular research direction in 6G era, with wireless sensor networks (WSNs) serving as an important functional infrastructure. WSNs enable key functionalities such as tracking, prediction, and navigation through data collected by pre-deployed sensors. The performance of WSNs is significantly impacted by the accuracy of node localization, as in many applications, the functionality of the system is inherently related to the precise location of nodes. This emphasizes the importance of developing localization techniques.
Overview
A number of existing localization techniques rely on the global positioning system (GPS), which presents challenges in terms of high cost and limited applicability in certain scenarios, especially those involving passive or hostile targets. Therefore, considerable research has focused on developing localization techniques using wireless communication links among nodes within WSNs. In particular, received signal strength (RSS)-based localization has attracted significant attention due to its minimal hardware requirements and cost-effectiveness. Localization techniques that utilize measurements from links between target nodes are termed cooperative, which usually improves performance over non-cooperative techniques that do not leverage such links.
Therefore, in this thesis, we propose various RSS-based cooperative localization techniques aiming at simultaneously localizing multiple target nodes within WSNs. Since RSS-based techniques leverage the relationship between signal attenuation and inter-node distance to estimate the location of unknown nodes, the target nodes’ transmit powers, the path loss exponent (PLE), and the precious locations of anchor nodes become key parameters for location estimation. We refer to them as model parameters in this thesis. However, model parameters are usually uncertain or unknown in practice, particularly in large-scale WSNs where precise pre-calibration is costly and impractical. To address this issue, our proposed localization techniques involve scenarios where model parameters are partially or entirely unknown, and we develop estimators to jointly estimate these parameters alongside the unknown target nodes’ locations.
Firstly, we focus on the scenario where the transmit power is unknown. We develop a mixed semidefinite programming (SDP)-second-order cone programming (SOCP) method to tackle the maximum likelihood (ML) problem for RSS-based localization. ML is widely used in parameter estimation due to its asymptotic optimality, but the ML objective function for RSS-based localization is non-convex, discontinuous, and non-differentiable. To overcome this challenge, we use first-order Taylor expansion and semidefinite relaxation (SDR) to convert the non-convex ML problem into an SDP formulation. However, due to the high complexity of SDP, we further introduce epigraph variables to transform the SDP problem into a mixed SDP-SOCP framework, resulting in reducing the computational complexity. We refer to the proposed technique as Fast Cooperative localization with Unknown Power (FCUP), which enables the joint estimation of both the target nodes’ locations and their transmit powers. FCUP takes advantage of the high accuracy of SDP and the low complexity of SOCP. Extensive simulations show that FCUP achieves superior performance in both location and power estimation accuracy compared to existing techniques, while also significantly reducing computational complexity.
Secondly, we extend the mixed SDP-SOCP framework to account for scenarios where the model parameters (target node’s transmit powers, PLE, and precious anchor node locations) are all unknown. We propose Cooperative localization Techniques with Unknown Parameters (CTUPs), which allow for the joint estimation of target node locations and unknown model parameters. To validate CTUPs, We deployed an extensive network comprising 50 BLE nodes covering an area of 640~m $\times$ 180~m to gather RSS data. The precise location of the nodes is obtained using real-time kinematics global positioning system (RTK-GPS), which is treated as the ground truth. Furthermore, to replicate real-world scenarios, we recorded the positions of the anchor nodes using a standard GPS, thereby introducing uncertainty into the anchor node locations. Extensive simulation and hardware experimentation demonstrate the superior performance of CTUP compared to existing techniques.
Lastly, we develop an augmented Lagrangian-based technique named Convex Relaxation with Centralized Armijo optimization (CR-CA) for scenarios where the target nodes’ transmit powers are unknown. CR-CA can significantly reduce the computational complexity by transforming the unconstrained ML problem into a constrained optimization problem using a convex approximation of the non-convex and discontinuous objective function. We demonstrate that the relaxed ML objective function possesses a Lipschitz continuous gradient. We solve the relaxed ML problem using the augmented Lagrange multiplier method and provide the theoretical proof of its convergence. Additionally, we derive the Cramer-Rao Lower Bound (CRLB) for RSS-based cooperative localization under scenarios where transmit power is unknown. We conduct extensive simulations and real-world experiments to verify the effectiveness of CR-CA, showcasing its superior accuracy in estimating nodes’ locations and transmit power. Simulations and experiments further validate that CRCA exhibits linear computational complexity with the number of wireless links, thus making it suitable for large-scale networks.
Presenters
Brief Biography
Yingquan Li is an M.S./Ph.D. candidate in the KAUST Communication Theory Lab under the supervision of Professor Mohamed-Slim Alouini. Before joining KAUST, Yingquan earned a bachelor's degree in electrical engineering from the University of Electronic Science and Technology of China.