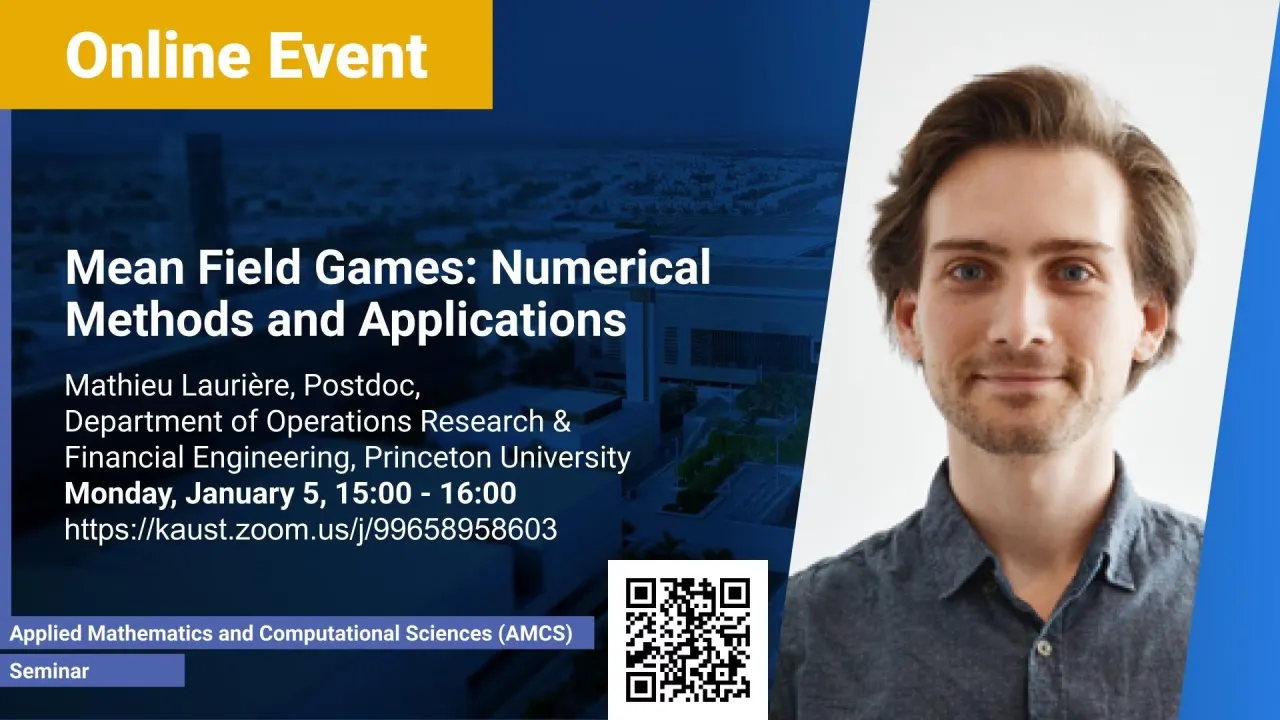
Mean Field Games: Numerical Methods and Applications
- Mathieu Laurière, Postdoc, Department of Operations Research and Financial Engineering, Princeton University
KAUST
In this talk, several numerical methods will be presented and illustrated on examples. Borrowing tools from stochastic analysis, optimization, partial differential equations and machine learning, these methods enable us to solve mean field games with possibly complex sources of noise or high dimensional state variables.
Overview
Abstract
In today’s highly interconnected world, many situations involve a large number of agents taking decisions. Mean field games have been introduced to cope with the fact that individual interactions become intractable as the size of the population grows. The key idea is to replace the microscopic viewpoint by a macroscopic one, which focuses on the interaction between a representative infinitesimal player and the population’s distribution. Nash equilibria or social optima can then be characterized through systems of stochastic or partial differential equations, consisting of a forward equation for the distribution and a backward equation for the optimal control. Mean field games have found a wide range of applications, from economic markets, to financial systemic risk or crowd motion. To solve concrete problems, computing solutions is a crucial step, which remains challenging, particularly due to the aforementioned forward-backward structure. In this talk, several numerical methods will be presented and illustrated on examples. Borrowing tools from stochastic analysis, optimization, partial differential equations and machine learning, these methods enable us to solve mean field games with possibly complex sources of noise or high dimensional state variables.
Brief Biography
Mathieu Laurière is currently a Postdoctoral Research Associate at Princeton University, in the Operations Research and Financial Engineering (ORFE) department. Prior to joining Princeton University, he was a Global Postdoctoral Fellow at the NYU-ECNU Institute of Mathematical Sciences at New York University Shanghai. He obtained his MS from Sorbonne University (Paris 6) and Ecole Normale Supérieure (ENS Cachan), and his PhD from Paris University (Paris 7). His research interests include mean field games, computational methods, stochastic optimal control, partial differential equations and machine learning.