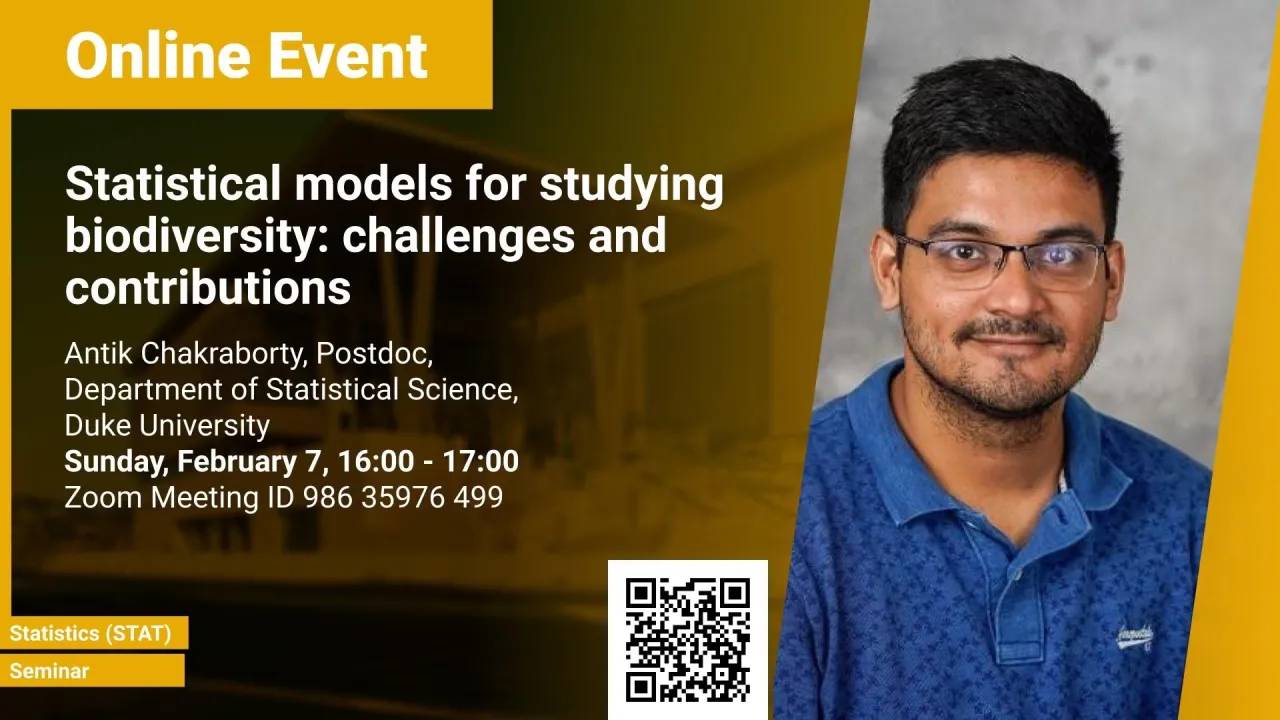
Statistical models for studying biodiversity: challenges and contributions
- Antik Chakraborty, Postdoc, Department of Statistical Science, Duke University
KAUST
A healthy ecosystem is essential to our well being and to ensure that we need a better understanding of the life or biodiversity around us. In the first part of this talk, I will introduce how modern technology is being used to collect data on biodiversity across space and time. These data come in complex forms such as DNA sequences, high-dimensional binary vectors, sound signals, etc. I will briefly touch upon the statistical challenges involved in making sense of these data. In the second part, I will elaborate on a project motivated by studying temporal patterns in bird vocalizations. I will introduce a new class of semiparametric latent variable models for long memory discretized event data. The proposed class of FRActional Probit (FRAP) models are based on the thresholding of a latent process consisting of an additive expansion of a smooth Gaussian process with a fractional Brownian motion. I will describe a Bayesian approach to inference using Markov chain Monte Carlo. Results from applying the model on Amazon bird vocalization data will be presented which provide substantial evidence for self-similarity and non-Markovian/Poisson dynamics. A hierarchical extension of the proposed model to accommodate vocalizations of multiple birds at the same time will also be discussed.
Overview
Abstract
A healthy ecosystem is essential to our well being and to ensure that we need a better understanding of the life or biodiversity around us. In the first part of this talk, I will introduce how modern technology is being used to collect data on biodiversity across space and time. These data come in complex forms such as DNA sequences, high-dimensional binary vectors, sound signals, etc. I will briefly touch upon the statistical challenges involved in making sense of these data.
In the second part, I will elaborate on a project motivated by studying temporal patterns in bird vocalizations. I will introduce a new class of semiparametric latent variable models for long memory discretized event data. The proposed class of FRActional Probit (FRAP) models are based on the thresholding of a latent process consisting of an additive expansion of a smooth Gaussian process with a fractional Brownian motion. I will describe a Bayesian approach to inference using Markov chain Monte Carlo. Results from applying the model on Amazon bird vocalization data will be presented which provide substantial evidence for self-similarity and non-Markovian/Poisson dynamics. A hierarchical extension of the proposed model to accommodate vocalizations of multiple birds at the same time will also be discussed.
Brief Biography
I am a Postdoctoral Associate at the Department of Statistical Sciences, Duke University since August 2018. I work with Prof. David Dunson on Bayesian statistical methods with a special focus on binary or count data types. Before coming to Duke, I was at Texas A&M University where I obtained my Ph.D. under the joint supervision of Prof. Bani K. Mallick and Prof. Anirban Bhattacharya from the Department of Statistics.