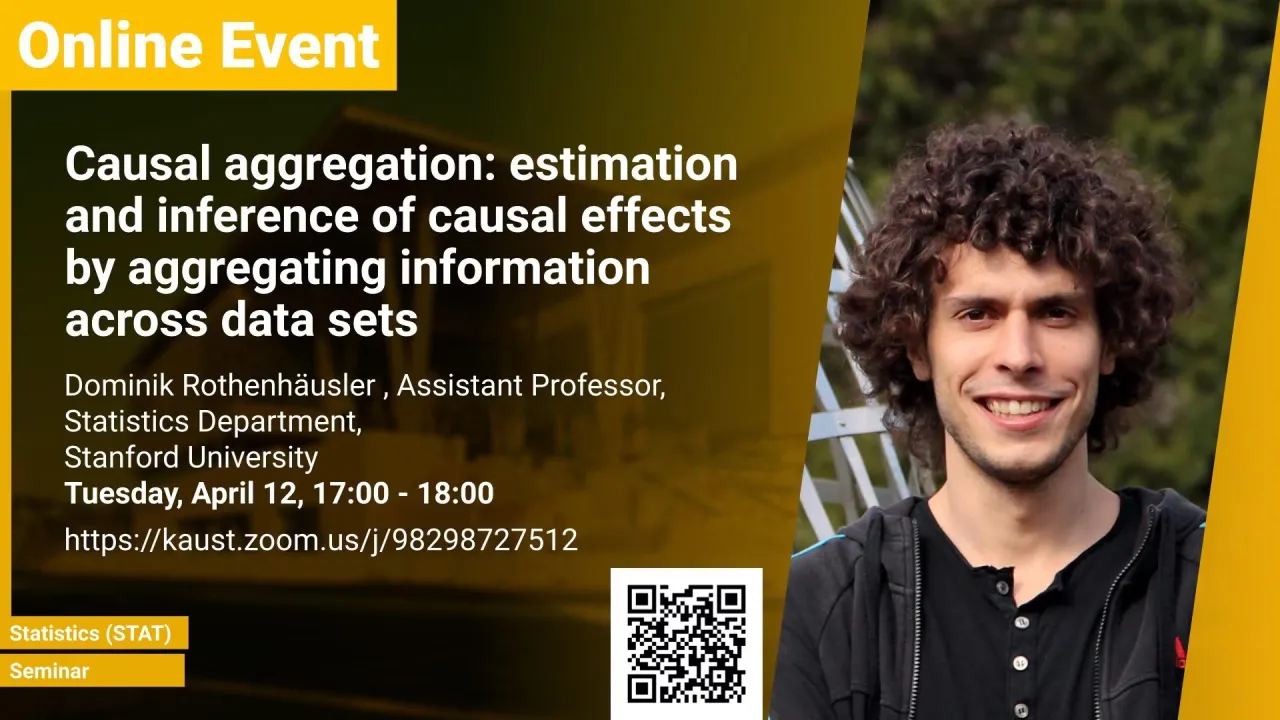
Causal aggregation: estimation and inference of causal effects by aggregating information across data sets
Randomized experiments are the gold standard for causal inference. In experiments, usually, one variable is manipulated and its effect is measured on an outcome. However, practitioners may also be interested in the effect of simultaneous interventions on multiple covariates on a fixed target variable.
Overview
Abstract
Randomized experiments are the gold standard for causal inference. In experiments, usually, one variable is manipulated and its effect is measured on an outcome. However, practitioners may also be interested in the effect of simultaneous interventions on multiple covariates on a fixed target variable. We discuss a method that allows estimating the effect of joint interventions using data from different experiments in which only very few variables are manipulated. If the joint causal effect is linear, the proposed method can be used for estimation and inference of joint causal effects. We describe extensions to the non-linear and high-dimensional case and discuss conditions under which direct causal effects can be consistently estimated. The proposed method allows to combine data sets arising from randomized experiments as well observational data sets for which IV assumptions or unconfoundedness hold. We demonstrate the effectiveness of the proposed method on synthetic and semi-synthetic data.
Brief Biography
Dominik Rothenhäusler completed his Ph.D. studies under the supervision of Nicolai Meinshausen and Peter Bühlmann at ETH Zürich. After a short stint as a post-doc at UC Berkeley, he joined the Statistics Department at Stanford as an assistant professor in Fall 2019. His research interests are causal inference, heterogeneous data, and replicability.