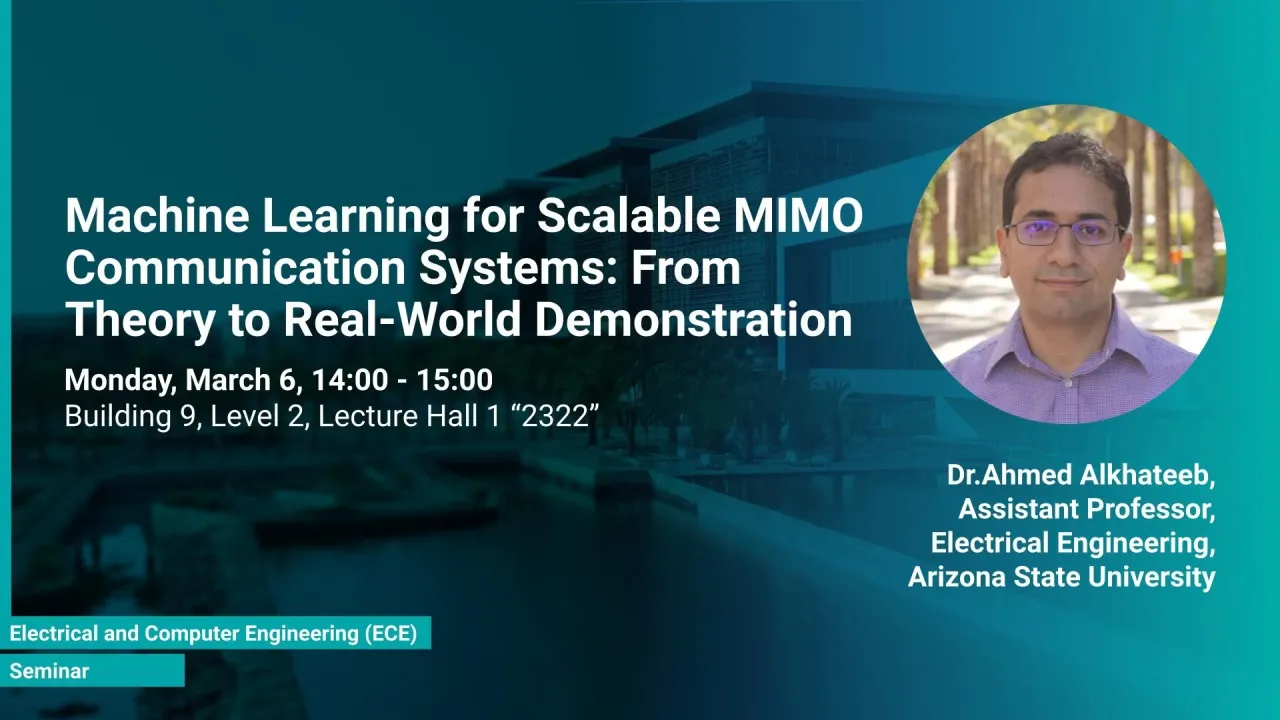
Machine Learning for Scalable MIMO Communication Systems: From Theory to Real-World Demonstration
- Dr. Ahmed Alkhateeb, Electrical Engineering , Arizona State University
B9 L2 H1
Large-scale MIMO communication (at sub-6GHz, mmWave, and sub-THz bands) is a key enabler for 5G, 6G, and beyond. Scaling MIMO systems, however, is subject to critical challenges, such as the large channel acquisition and beam training overhead and the sensitivity to channel estimation errors (especially at lower frequencies) and blockages (at higher frequencies). These challenges make it difficult for MIMO systems to support applications that have high mobility and strict reliability constraints. In this talk, I will first motivate the use of machine learning and sensory data to address these challenges. Then, I will present a few key machine-learning roles, enabling datasets, and recent hardware proof-of-concept prototypes that demonstrate the machine-learning gains in real-world environments.
Overview
Abstract
Large-scale MIMO communication (at sub-6GHz, mmWave, and sub-THz bands) is a key enabler for 5G, 6G, and beyond. Scaling MIMO systems, however, is subject to critical challenges, such as the large channel acquisition and beam training overhead and the sensitivity to channel estimation errors (especially at lower frequencies) and blockages (at higher frequencies). These challenges make it difficult for MIMO systems to support applications that have high mobility and strict reliability constraints. In this talk, I will first motivate the use of machine learning and sensory data to address these challenges. Then, I will present a few key machine-learning roles, enabling datasets, and recent hardware proof-of-concept prototypes that demonstrate the machine-learning gains in real-world environments.
Brief Biography
Ahmed Alkhateeb received his B.S. and M.S. degrees in Electrical Engineering from Cairo University, Egypt, in 2008 and 2012, and his Ph.D. degree in Electrical Engineering from The University of Texas at Austin, USA, in 2016. After the Ph.D., he spent some time as a Wireless Communications Researcher at the Connectivity Lab, Facebook, before joining Arizona State University (ASU) in Spring 2018, where he is currently an Assistant Professor in the School of Electrical, Computer, and Energy Engineering. His research interests are in the broad areas of wireless communications, signal processing, machine learning, and applied math. Dr. Alkhateeb is the recipient of the 2012 MCD Fellowship from The University of Texas at Austin, the 2016 IEEE Signal Processing Society Young Author Best Paper Award for his work on hybrid precoding and channel estimation in millimeter-wave communication systems, and the NSF CAREER Award 2021 to support his research on leveraging machine learning for large-scale MIMO systems.