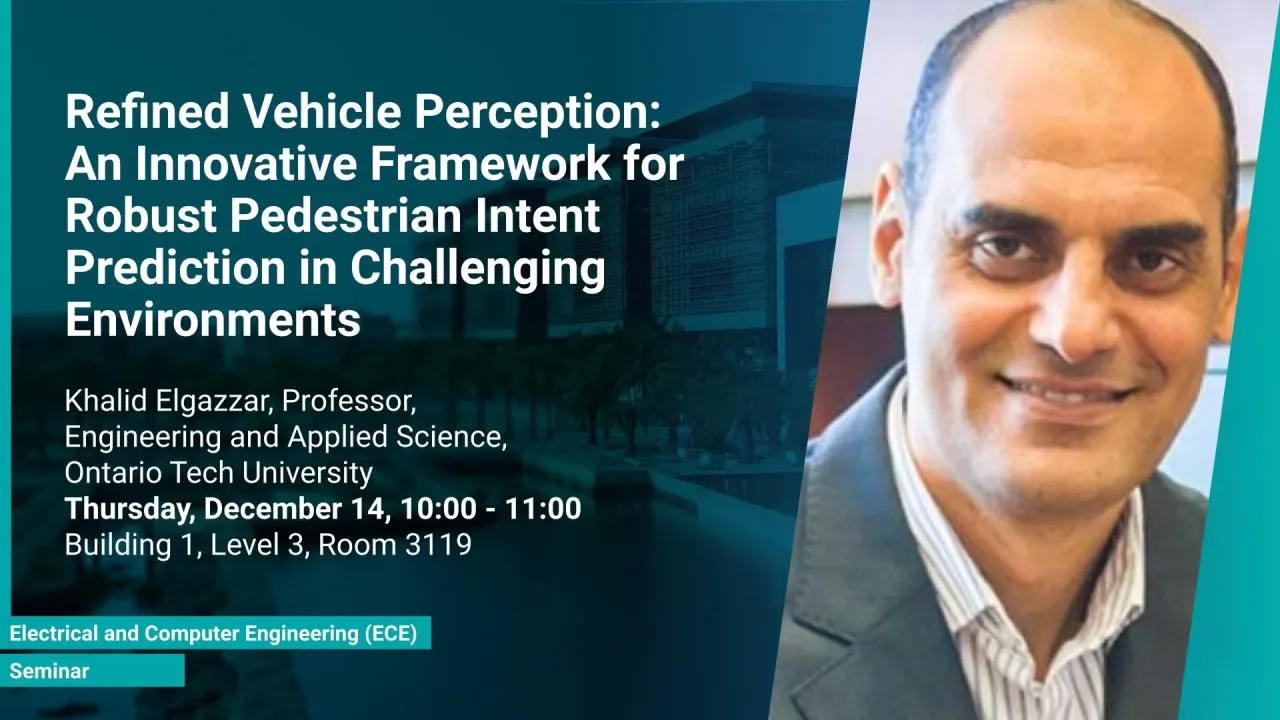
Refined Vehicle Perception: An Innovative Framework for Robust Pedestrian Intent Prediction in Challenging Environments
- Khalid Elgazzar, Professor, Engineering and Applied Science, Ontario Tech University
B1 L3 R3119
In this talk, I will present an innovative framework we developed to address these limitations and accurately predict pedestrian crossing intentions. At the core of the framework, we implement an image enhancement pipeline to enable the detection and rectification of various defects that may arise during unfavorable weather conditions. Following this, we employ a transformer-based network with a self-attention mechanism to predict the crossing intentions of pedestrians. This pipeline enhances the model's robustness and accuracy in classification tasks. We assessed our framework using the famous JAAD dataset. Performance metrics indicate that our model achieves state-of-the-art results while ensuring significantly low inference times.
Overview
Abstract
Enhancing vehicle perception models is crucial for the successful integration of assisted and autonomous driving vehicles. By refining these perceptual capabilities of the model to accurately anticipate the actions of vulnerable road users, the overall driving experience can be significantly improved, ensuring higher levels of safety. Existing research efforts focused on the prediction of pedestrians' crossing intentions have predominantly relied on vision-based deep learning models. However, these models continue to exhibit shortcomings in terms of their robustness when faced with adverse weather conditions and domain adaptation challenges. Furthermore, little attention has been given to evaluating the real-time performance of these models. In this talk, I will present an innovative framework we developed to address these limitations and accurately predict pedestrian crossing intentions. At the core of the framework, we implement an image enhancement pipeline to enable the detection and rectification of various defects that may arise during unfavorable weather conditions. Following this, we employ a transformer-based network with a self-attention mechanism to predict the crossing intentions of pedestrians. This pipeline enhances the model's robustness and accuracy in classification tasks. We assessed our framework using the famous JAAD dataset. Performance metrics indicate that our model achieves state-of-the-art results while ensuring significantly low inference times.
Brief Biography
Prof. Khalid Elgazzar is a Canada Research Chair in the Internet of Things and an Associate Professor with the Faculty of Engineering and Applied Science at Ontario Tech University, Canada. He is also an adjunct professor at Queen's University. Dr. Elgazzar is the founder and director of the IoT Research Laboratory at Ontario Tech University. Prior to joining Ontario Tech, he was an assistant professor at the University of Louisiana at Lafayette and a research scientist at Carnegie Mellon School of Computer Science. Dr. Elgazzar was named the recipient of the Outstanding Achievement in Sponsored Research award from UL Lafayette in 2017 and the Distinguished Research Award from Queen's University in 2014. He also received several recognition and best paper awards at top international venues. Dr. Elgazzar is a leading authority in the areas of the Internet of Things (IoT), intelligent software systems, real-time data analytics, and mobile computing. Dr. Elgazzar is currently an associate editor for Frontiers Internet of Things Journal, Springer Peer-to-Peer Networking and Applications, Future Internet, and others. He also chaired several IEEE conferences and symposia on mobile computing, communications, and IoT. Dr. Elgazzar is a Senior IEEE Member and an active volunteer in technical program committees and organizing committees in both IEEE and ACM events.