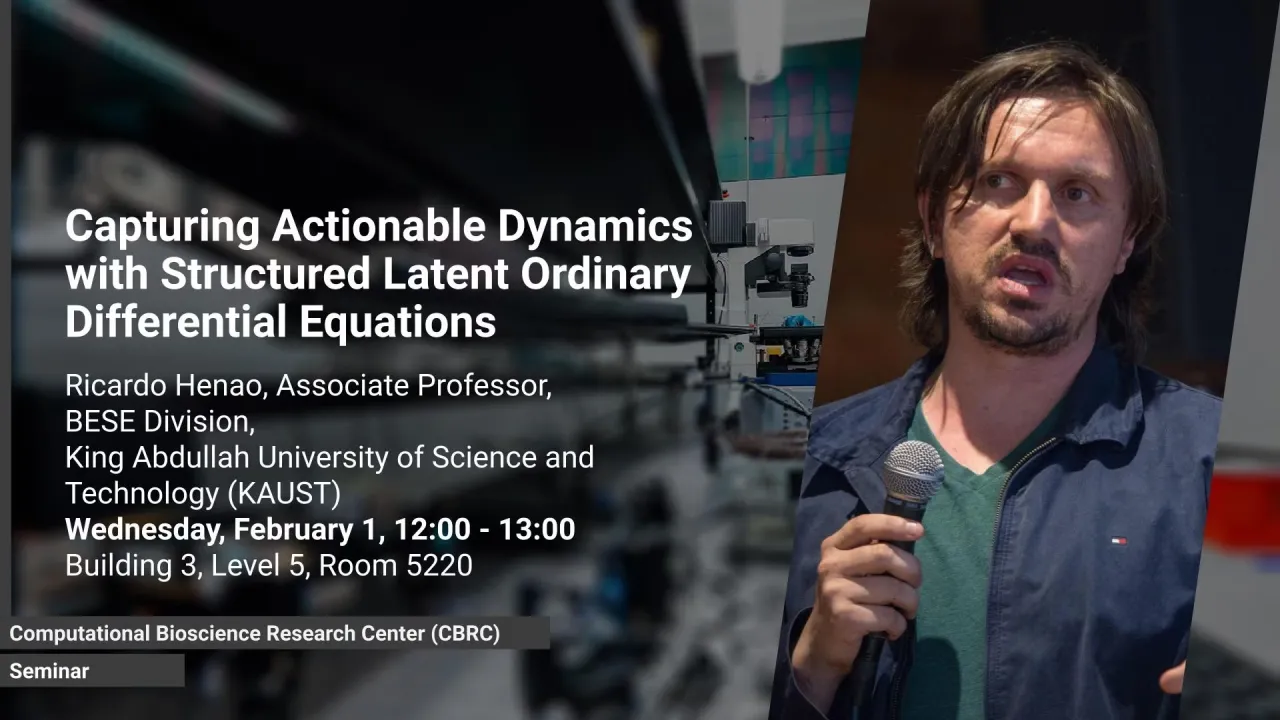
Capturing Actionable Dynamics with Structured Latent Ordinary Differential Equations
-
- Prof. Ricardo Henao, Associate Professor, BESE Division, KAUST
B3 L5 R5220
We propose a structured latent ODE model that explicitly captures system input variations within its latent representation. Building on a static latent variable specification, our model learns (independent) stochastic factors of variation for each input to the system, thus separating the effects of the system inputs in the latent space. This approach provides actionable modeling through the controlled generation of time-series data for novel input combinations (or perturbations). Additionally, we propose a flexible approach for quantifying uncertainties, leveraging a quantile regression formulation.
Overview
Abstract
End-to-end learning of dynamical systems with black-box models, such as neural ordinary differential equations (ODEs), provides a flexible framework for learning dynamics from data without prescribing a mathematical model for the dynamics. Unfortunately, this flexibility comes at the cost of understanding the dynamical system, for which ODEs are used ubiquitously. Further, experimental data are collected under various conditions (inputs), such as treatments, or grouped in some way, such as part of sub-populations. Understanding the effects of these system inputs on system outputs is crucial to have any meaningful model of a dynamical system. To that end, we propose a structured latent ODE model that explicitly captures system input variations within its latent representation. Building on a static latent variable specification, our model learns (independent) stochastic factors of variation for each input to the system, thus separating the effects of the system inputs in the latent space. This approach provides actionable modeling through the controlled generation of time-series data for novel input combinations (or perturbations). Additionally, we propose a flexible approach for quantifying uncertainties, leveraging a quantile regression formulation. Results on challenging biological datasets show consistent improvements over competitive baselines in the controlled generation of observational data and inference of biologically meaningful system inputs.
Brief Biography
Ricardo Henao, a quantitative scientist, is an Associate Professor in the Biological and Environmental Science and Engineering (BESE) Division, member of the Smart Health Initiative (SHI), at KAUST (King Abdullah University of Science and Technology). He is also currently an Associate Professor in the department of Biostatistics and Bioinformatics, Department of Electrical and Computer Engineering (ECE), member of the Information Initiative at Duke (iiD), Duke AI Health and the Duke Clinical Research Institute (DCRI), all at Duke University. The theme of his research is the development of novel statistical methods and machine learning algorithms primarily based on probabilistic modeling. His expertise covers several fields including applied statistics, signal processing, pattern recognition and machine learning. His methods research focuses on hierarchical or multilayer probabilistic models to describe complex data, such as that characterized by high-dimensions, multiple modalities, more variables than observations, noisy measurements, missing values, time-series, multiple modalities, etc., in terms of low-dimensional representations for the purposes of hypothesis generation and improved predictive modeling. Most of his applied work is dedicated to the analysis of biological data such as gene expression, medical imaging, clinical narrative, and electronic health records. His recent work has been focused on the development of sophisticated machine learning models, including deep learning approaches, for the analysis and interpretation of clinical and biological data with applications to predictive modeling for diverse clinical outcomes.