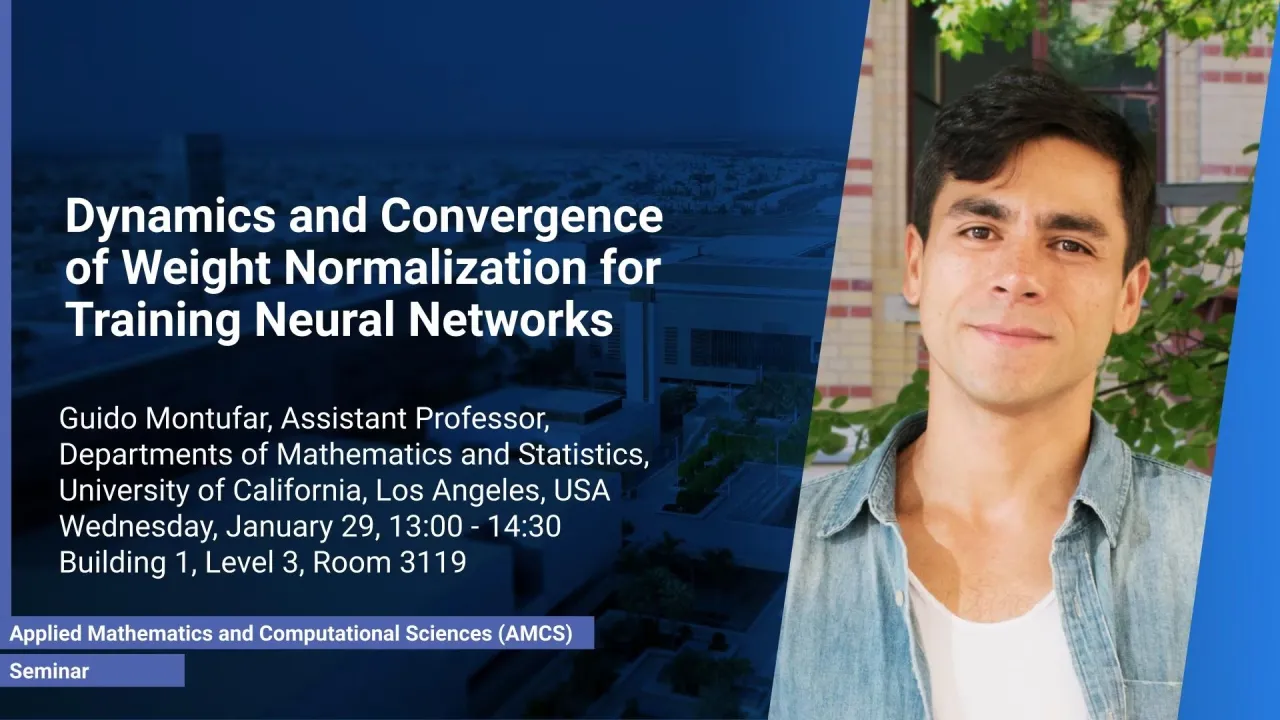
Dynamics and Convergence of Weight Normalization for Training Neural Networks
- Guido Montufar, Assistant Professor, Departments of Mathematics and Statistics, University of California, Los Angeles (UCLA)
B1 L3 R3119
We present a result on the convergence of weight normalized training of artificial neural networks. In the analysis, we consider over-parameterized 2-layer networks with rectified linear units (ReLUs) initialized at random and trained with batch gradient descent and a fixed step size. The proof builds on recent theoretical works that bound the trajectory of parameters from their initialization and monitor the network predictions via the evolution of a ''neural tangent kernel'' (Jacot et al. 2018). We discover that training with weight normalization decomposes such a kernel via the so called ''length-direction decoupling''. This in turn leads to two convergence regimes. From the modified convergence we make a few curious observations including a natural form of ''lazy training'' where the direction of each weight vector remains stationary.
Overview
Abstract
We present a result on the convergence of weight normalized training of artificial neural networks. In the analysis, we consider over-parameterized 2-layer networks with rectified linear units (ReLUs) initialized at random and trained with batch gradient descent and a fixed step size. The proof builds on recent theoretical works that bound the trajectory of parameters from their initialization and monitor the network predictions via the evolution of a ''neural tangent kernel'' (Jacot et al. 2018). We discover that training with weight normalization decomposes such a kernel via the so called ''length-direction decoupling''. This in turn leads to two convergence regimes. From the modified convergence we make a few curious observations including a natural form of ''lazy training'' where the direction of each weight vector remains stationary.
This is joint work with Yonatan Dukler and Quanquang Gu.
Brief Biography
Guido is an Assistant Professor at the Departments of Mathematics and Statistics at the University of California, Los Angeles (UCLA), and he is PI in the ERC Project „Deep Learning Theory: Geometric Analysis of Capacity, Optimization, and Generalization for Improving Learning in Deep Neural Networks" at MPI MiS. — at Max Planck Institute for Mathematics in the Sciences