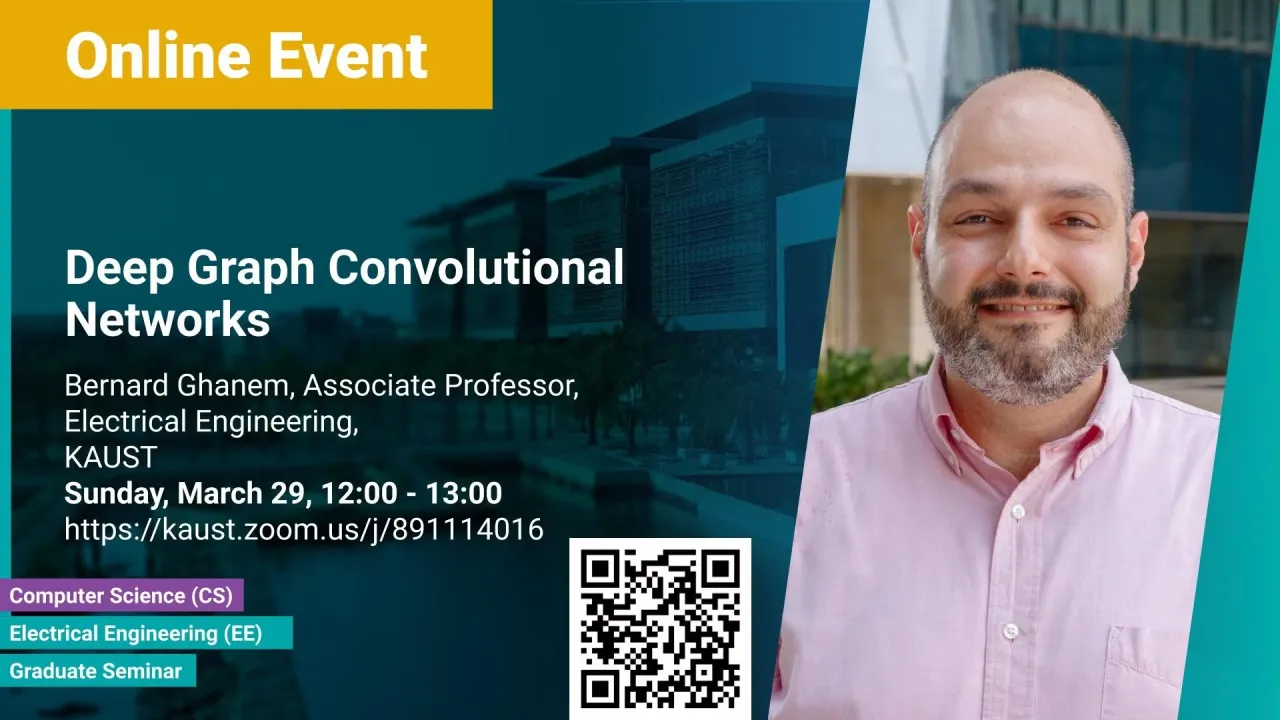
EE+CS Graduate Seminar: Deep Graph Convolutional Networks
In this talk, I will present a line of work done at the Image and Video Understanding Lab (IVUL), which focuses on developing deep graph convolutional networks (DeepGCNs). A GCN is a deep learning network that processes generic graph inputs, thus extending the impact of deep learning to irregular grid data including 3D point clouds and meshes, social graphs, protein interaction graphs, etc. By adapting architectural operations from the CNN realm and reformulating them for graphs, we were the first to show that GCNs can go as deep as CNNs. Developing such a high capacity deep learning platform for generic graphs opens up many opportunities for exciting research, which spans applications in the field of computer vision and beyond, architecture design, and theory. In this talk, I will showcase some of the GCN research done at IVUL and highlight some interesting research questions for future work.
Overview
Abstract
In this talk, I will present a line of work done at the Image and Video Understanding Lab (IVUL), which focuses on developing deep graph convolutional networks (DeepGCNs). A GCN is a deep learning network that processes generic graph inputs, thus extending the impact of deep learning to irregular grid data including 3D point clouds and meshes, social graphs, protein interaction graphs, etc. By adapting architectural operations from the CNN realm and reformulating them for graphs, we were the first to show that GCNs can go as deep as CNNs. Developing such a high capacity deep learning platform for generic graphs opens up many opportunities for exciting research, which spans applications in the field of computer vision and beyond, architecture design, and theory. In this talk, I will showcase some of the GCN research done at IVUL and highlight some interesting research questions for future work.
Brief Biography
Bernard Ghanem is currently an Associate Professor in the CEMSE division and a theme leader at the Visual Computing Center (VCC) at KAUST. Before that, he was a Senior Research Scientist at the University of Illinois Urbana-Champaign (UIUC) in Singapore. His research interests lie in computer vision, machine learning, and optimization. He received his Bachelor’s degree from the American University of Beirut (AUB) in 2005 and his MS/Ph.D. in from UIUC in 2010. His work has received several awards and honors, including the Henderson Graduate Award and CSE Fellowship awards from UIUC, three Best Workshop Paper Awards (CVPRW 2013, ECCVW 2018, and CVPRW2019), a two-year KAUST Seed Fund, and a Google Faculty Research Award in 2015. He has co-authored more than 100 peer-reviewed conference and journal papers in his field as well as two issued patents.