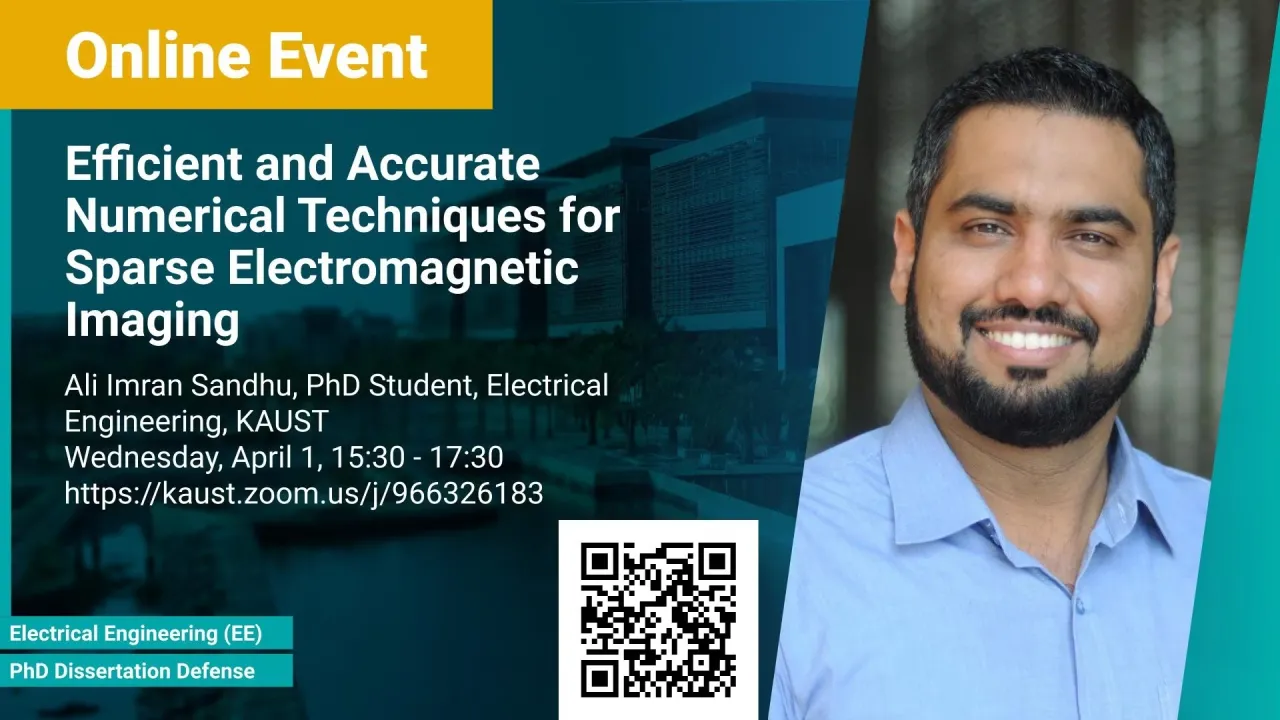
Efficient and Accurate Numerical Techniques for Sparse Electromagnetic Imaging
In this thesis, efficient solutions are sought out to fundamental problems in Electromagnetic (EM) imaging that determines the shape, location, and material properties of an (unknown) object of interest in an investigation domain from the scattered field measured away from it. The solution of an EM inverse scattering problem inherently poses two main challenges: (i) non-linearity, since the scattered field is a non-linear function of the material properties and (ii) ill-posedness, since the integral operator has a smoothing effect and the number of measurements is finite in dimension and they are contaminated with noise. The non-linearity is tackled incorporating a multitude of techniques (ranging from Born approximation (linear), inexact Newton (linearized) to complete non-linear iterative Landweber schemes) that can account for weak to strong scattering problems. The ill-posedness of the EM inverse scattering problem is circumvented by formulating the above methods into a minimization problem with a sparsity constraint, which assumes that the dimension of the unknown object relative to the investigation domain is much smaller. Numerical experiments, which are carried out using synthetically generated measurements, show that the images recovered by these sparsity-regularized methods are sharper and more accurate than those produced by existing methods. The methods developed in this work have potential application areas ranging from oil/gas reservoir engineering to biological imaging where sparse domains naturally exist.
Overview
Abstract
Electromagnetic (EM) imaging is aimed at determining the shape, location, and material properties of an (unknown) object of interest in an investigation domain from the scattered field measured away from it. The solution of an EM inverse scattering problem inherently poses two main challenges: (i) non-linearity, since the scattered field is a non-linear function of the material properties and (ii) ill-posedness, since the integral operator has a smoothing effect and the number of measurements is finite in dimension and they are contaminated with noise. Albeit there exist remedies to these fundamental problems, more efficient solutions are still being sought. To this end, in this thesis, the non-linearity is tackled incorporating a multitude of techniques (ranging from Born approximation (linear), inexact Newton (linearized) to complete non-linear iterative Landweber schemes) that can account for weak to strong scattering problems. The ill-posedness of the EM inverse scattering problem is circumvented by formulating the above methods into a minimization problem with a sparsity constraint, which assumes that the dimension of the unknown object relative to the investigation domain is much smaller. More specifically, four novel inverse scattering schemes are formulated and implemented. (i) A greedy algorithm is used together with a simple artificial neural network (ANN) for efficient and accurate EM imaging of weak scatterers. The ANN is used to predict the sparsity level of the investigation domain, which is then used as the L0 - constraint parameter for the greedy algorithm. The non-linearity is tackled using the Born approximation. A constant is added to the diagonal entries of the scattering matrix (resulting in a Tikhonov-type regularization), which ensures that the restricted isometric property required by the greedy algorithm is satisfied. The resulting EM inversion scheme is computationally efficient since it calls for the solution of a smaller least squares problem on a reduced set determined by the sparsity level. Additionally, unlike the existing sparsity-promoting schemes, this scheme does not require tuning of a thresholding parameter. (ii) An inexact Newton scheme that enforces the sparsity constraint on the derivative of the unknown material properties (not necessarily sparse) is proposed. More precisely, the investigation domain is “sparsified” by applying the 2D gradient to the material properties such that the scattered field is formulated as a non-linear function of this gradient. Each Newton iteration calls for the solution of a linear system involving the Frechet’s derivative matrix of the forward scattering operator. This approach results in significant sparsification where any sparsity regularization method could be efficiently applied. (iii) Sparsity regularized non-linear contrast source (CS) framework is developed to directly solve the non-linear minimization problem using Landweber iterations. This framework is accelerated using a self-adaptive projected steepest descent (A-PASD) algorithm. Projection, which replaces the thresholding operation, is used to enforce the sparsity constraint. The resulting scheme benefits from the faster convergence rate of the A-PASD and the lower computational cost of the CS formulation. (iv) A two and a half dimensional (2.5D) finite difference frequency domain (FDFD) based inverse scattering scheme is developed for imaging scatterers embedded in lossy and inhomogeneous media. The FDFD based inversion algorithm does not require the Green’s function of the background medium and appears a promising technique for biomedical and subsurface imaging with a reasonable computational time. Numerical experiments, which are carried out using synthetically generated measurements, show that the images recovered by these sparsity-regularized methods are sharper and more accurate than those produced by existing methods. The methods developed in this work have potential application areas ranging from oil/gas reservoir engineering to biological imaging where sparse domains naturally exist.
Brief Biography
Ali Imran Sandhu received the B.S. degree in electronics engineering from COMSATS Institute of Information Technology (CIIT), Pakistan, in 2007 and the M.S. degree in communication engineering from CHALMERS University of Science and Technology, Gothenburg, Sweden in 2010. He is currently pursuing the Ph.D. degree in electrical engineering at the Division of Computer, Electrical, and Mathematical Sciences and Engineering (CEMSE), King Abdullah University of Science and Technology (KAUST), Thuwal, Saudi Arabia.
Since September 2007, he has been a Lecturer with the Department of Electrical Engineering, CIIT Lahore, Pakistan. From September 2011 to March 2013, he worked as a Research Engineer with the Microwave Lab, University of Calabria, Italy. His research interests include inverse electromagnetic scattering problems with emphasis on sparsity-promoting regularization schemes, designing microwave devices and phased array antennas.