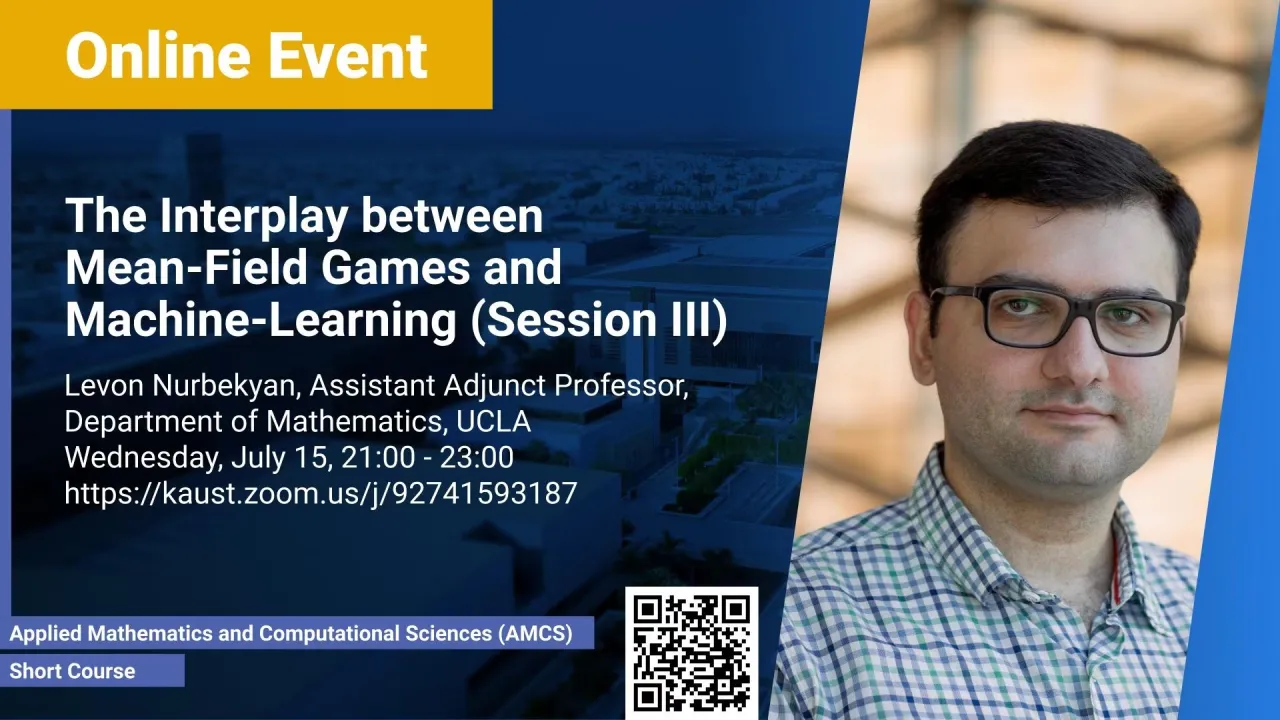
The Interplay between Mean-Field Games and Machine-Learning (Session III)
- Prof. Levon Nurbekyan, Department of Mathematics, UCLA
KAUST
In this short course, I will discuss connections between mean-field games (MFG) systems and modern machine-learning (ML) techniques and problems. In the first part of the course, roughly the first two lectures, I will present how various ML techniques can be applied to solve high-dimensional MFG systems that are far out of reach for traditional methods. In the second part of the course, I will discuss the reverse relation, namely, how the MFG framework can be useful for solving specific ML problems.
Overview
Abstract
In this short course, I will discuss connections between mean-field games (MFG) systems and modern machine-learning (ML) techniques and problems. In the first part of the course, roughly the first two lectures, I will present how various ML techniques can be applied to solve high-dimensional MFG systems that are far out of reach for traditional methods. In the second part of the course, I will discuss the reverse relation, namely, how the MFG framework can be useful for solving specific ML problems.
Brief Biography
I am currently an Assistant Adjunct Professor at the Department of Mathematics at UCLA. I previously held postdoctoral and visiting positions at McGill University, King Abdullah University of Science and Technology, National Academy of Sciences of Armenia, and Technical University of Lisbon. I have also been a Senior Fellow at the Institute for Pure and Applied Mathematics (IPAM) at UCLA for its Spring 2020 Program on High Dimensional Hamilton-Jacobi PDEs and a Simons CRM Scholar at the Centre de Recherches Mathématiques (CRM) at the University of Montreal for its Spring 2019 Program on Data Assimilation: Theory, Algorithms, and Applications. I obtained my Ph.D. in the framework of UT Austin -- Portugal CoLab under the supervision of Professors Diogo Gomes and Alessio Figalli. My research interests include calculus of variations, optimal control theory, mean-field games, partial differential equations, mathematics applied to machine learning, dynamical systems, and shape optimization problems.