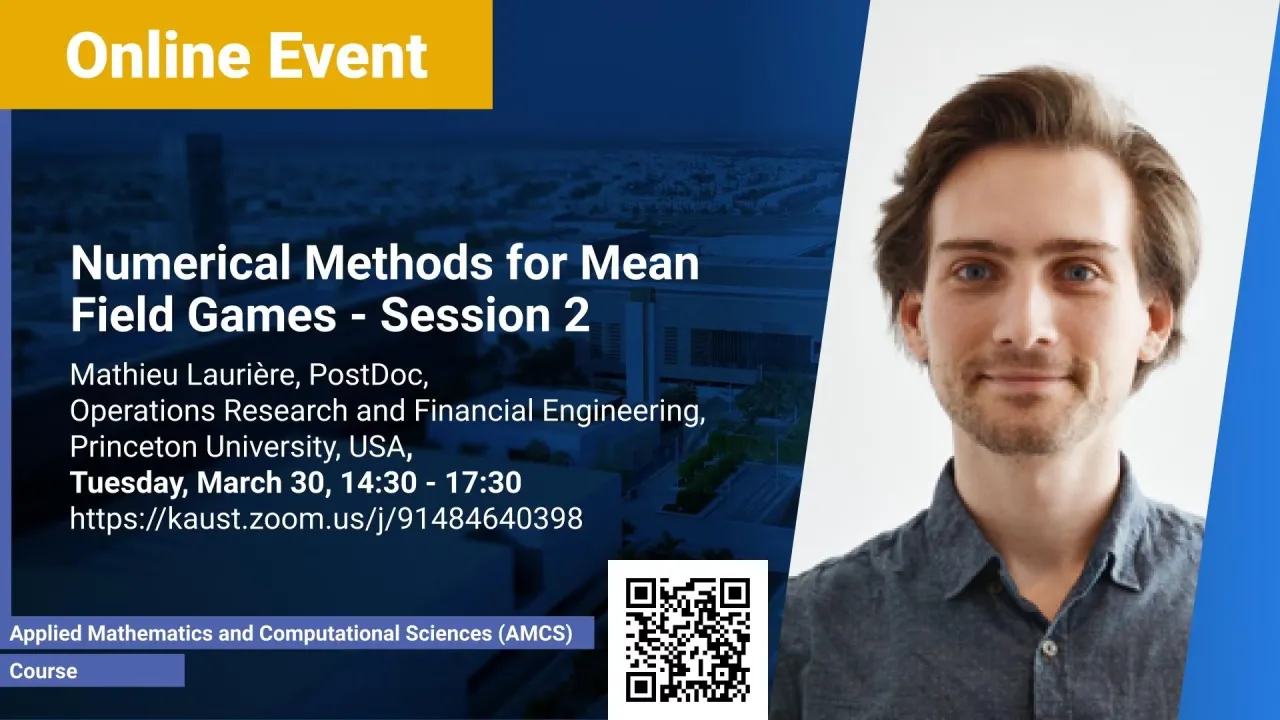
Numerical Methods for Mean Field Games- Session 2
- Mathieu Laurière, Postdoc, Operations Research and Financial Engineering, Princeton University, USA
KAUST
Mean field games and mean field control problems are frameworks to study Nash equilibria or social optima in games with a continuum of agents. These problems can be used to approximate competitive or cooperative situations with a large finite number of agents, and have found a broad range of applications, from economics to crowd motion, energy production and risk management. The solutions are typically characterized by a forward-backward system of partial differential equations (PDE) or stochastic differential equations (SDE).
Overview
Abstract
Mean field games and mean field control problems are frameworks to study Nash equilibria or social optima in games with a continuum of agents. These problems can be used to approximate competitive or cooperative situations with a large finite number of agents, and have found a broad range of applications, from economics to crowd motion, energy production and risk management. The solutions are typically characterized by a forward-backward system of partial differential equations (PDE) or stochastic differential equations (SDE). Due to their complex structure, Mean Field Games (MFG) admit explicit solutions only in some very special cases. For many applications, computational methods are hence a crucial tool. They allow us to get insights into the shape of the solutions and to obtain quantitative results. In this course, we will review several numerical approaches. We will first present methods based on a finite difference scheme for the aforementioned PDE system. We will then discuss stochastic methods relying on neural network approximations. If time permits, we will also present approaches for MFG based on algorithmic game theory. Several numerical illustrations such as crowd motion with congestion effects or systemic risk will be provided
Brief Biography
Mathieu Laurière is currently a Postdoctoral Research Associate at Princeton University, in the Operations Research and Financial Engineering (ORFE) department. He obtained my MS from Sorbonne University and ENS Cachan, and his PhD from University of Paris. Prior to joining Princeton University, he was a Postdoctoral Fellow at the NYU-ECNU Institute of Mathematical Sciences at NYU Shanghai.