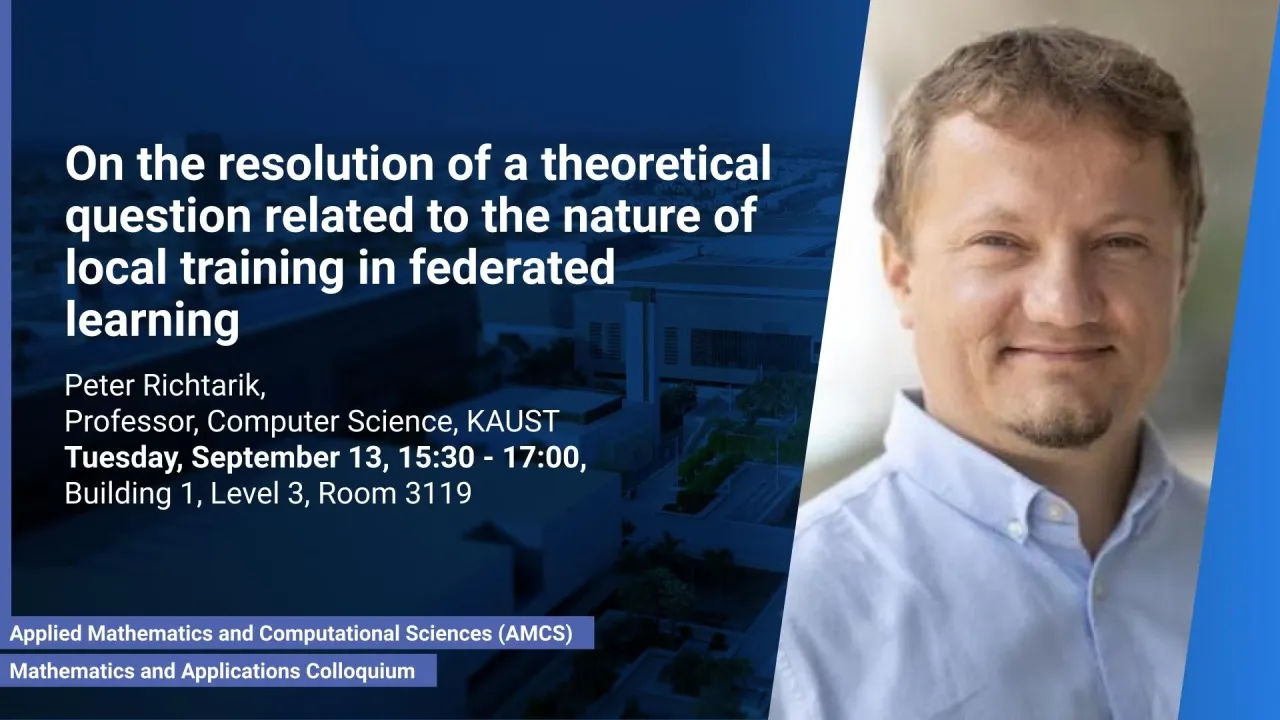
On the resolution of a theoretical question related to the nature of local training in federated learning
In this talk, I will explain the problem, its solution, and some subsequent work generalizing, extending and improving the ProxSkip method in various ways. We study distributed optimization methods based on the local training (LT) paradigm - achieving improved communication efficiency by performing richer local gradient-based training on the clients before parameter averaging - which is of key importance in federated learning. Looking back at the progress of the field in the last decade, we identify 5 generations of LT methods: 1) heuristic, 2) homogeneous, 3) sublinear, 4) linear, and 5) accelerated. The 5th generation, initiated by the ProxSkip method of Mishchenko et al (2022) and its analysis, is characterized by the first theoretical confirmation that LT is a communication acceleration mechanism.
Overview
Abstract
We study distributed optimization methods based on the local training (LT) paradigm - achieving improved communication efficiency by performing richer local gradient-based training on the clients before parameter averaging - which is of key importance in federated learning. Looking back at the progress of the field in the last decade, we identify 5 generations of LT methods: 1) heuristic, 2) homogeneous, 3) sublinear, 4) linear, and 5) accelerated. The 5th generation, initiated by the ProxSkip method of Mishchenko et al (2022) and its analysis, is characterized by the first theoretical confirmation that LT is a communication acceleration mechanism. In this talk, I will explain the problem, its solution, and some subsequent work generalizing, extending and improving the ProxSkip method in various ways.
References:
- Konstantin Mishchenko, Grigory Malinovsky, Sebastian Stich and Peter Richtárik. ProxSkip: Yes! Local gradient steps provably lead to communication acceleration! Finally! Proceedings of the 39th International Conference on Machine Learning, 2022
- Grigory Malinovsky, Kai Yi and Peter Richtárik. Variance reduced ProxSkip: Algorithm, theory and application to federated learning, arXiv:2207.04338, 2022
- Laurent Condat and Peter Richtárik. RandProx: Primal-dual optimization algorithms with randomized proximal updates, arXiv:2207.12891, 2022
- Abdurakhmon Sadiev, Dmitry Kovalev and Peter Richtárik. Communication acceleration of local gradient methods via an accelerated primal-dual algorithm with inexact prox, arXiv:2207.03957, 2022
Brief Biography
Peter Richtárik is a Professor of Computer Science at KAUST. He is an EPSRC Fellow in Mathematical Sciences, Fellow of the Alan Turing Institute, and is affiliated with the Visual Computing Center and the Extreme Computing Research Center at KAUST.