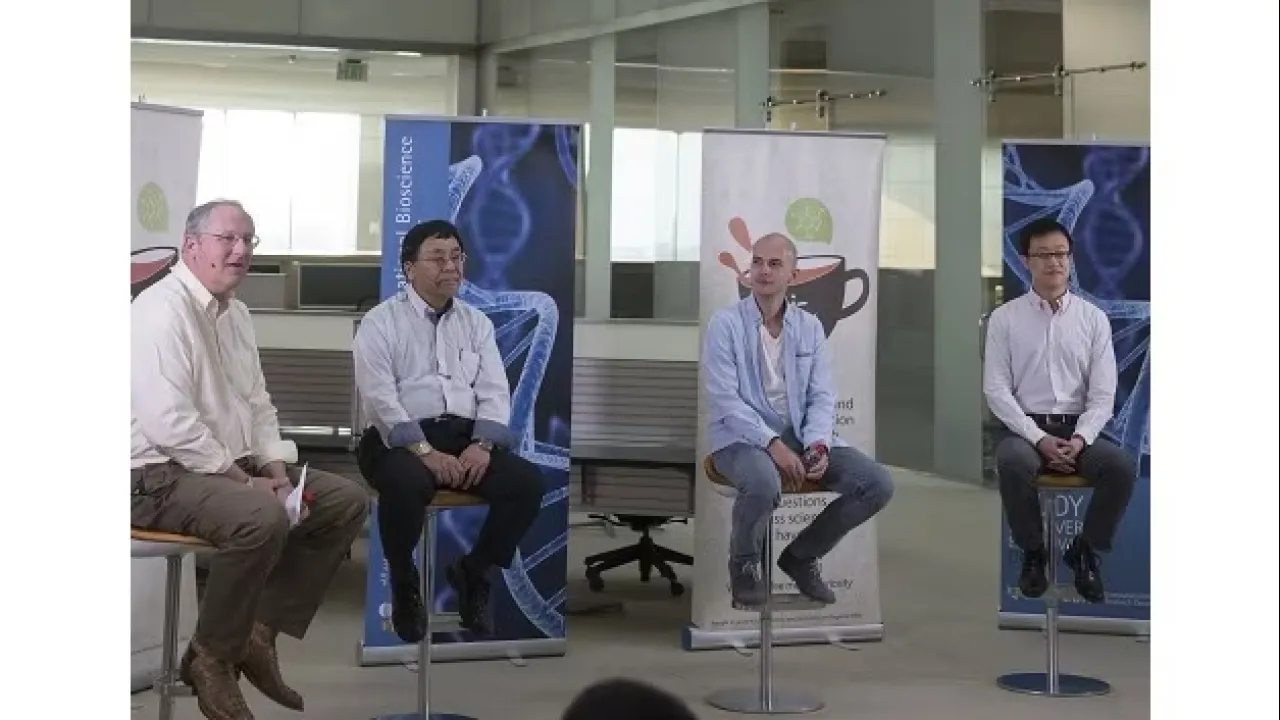
The awesome scope of big data
Sifting through huge amounts of data may bring a better understanding of whale shark social structures, protein targets for drug therapies and disease-causing genes.
About
Anyone looking for answers on personalized medicine, human health, food production, the environment or ecology will turn to big data, says James Calvin, vice president of academic affairs at KAUST; “Big data is a concept that permeates all of the biological sciences.”
Researchers at the University’s Computational Bioscience Research Center work at the intersection of computer science and biology to sift through huge amounts of biomedical and biotechnological data. Their work, and that of international colleagues, could give clues to some major questions in the life sciences.
“In the last 10-15 years, technological breakthroughs have allowed us to produce more and more data,” says computer scientist Robert Hoehndorf. To put things in perspective, he says, tens of thousands of papers have been published on diabetes, producing large amounts of research data that are uploaded to databases; however, “How can we connect all these different research results to provide a big picture?” he asks. Integrating this data could allow a better understanding of disease, guiding researchers toward potential treatments.
Hoehndorf’s main area of interest is the field of symbolic artificial intelligence (AI), which explores how to make machines that are similarly intelligent to humans. AI systems are being used to study health problems and to do biomedical research, he explains. In the area of big data, these systems are being used to integrate huge amounts of data and identify consistencies and contradictions within them.
Hoehndorf and colleagues developed a computational method that allows the integration of data on tens of thousands of observable disease characteristics in yeast, fish, worms, flies, mice and humans. The method, called PhenomeNet, computes similarity between two sets of phenotypes—observable characteristics that result from the interactions of genes with the environment. This can help suggest genes that might underpin disease.
Focusing on a different target, computational scientist Xin Gao is interested in developing computational models and machine-learning techniques to analyze protein structures, determine what they look like three-dimensionally, how they function, and how their behaviors can be controlled in complex biological networks.
"I do not know the answer to whether big data can directly solve our health problems, but I do know with certainty that if these problems can be solved, then big data will be a part of the solutions," says Gao.
Read the full article