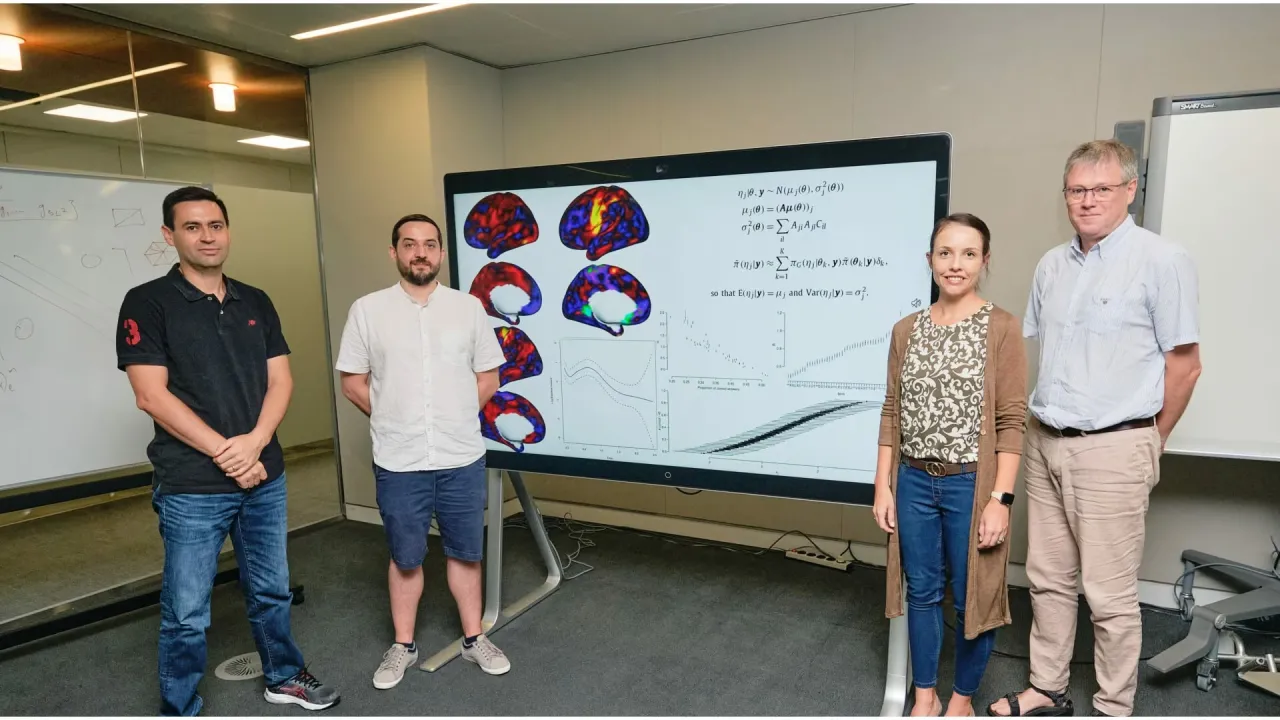
Real-time modeling a step closer to reality
An efficiency upgrade for an already fast approximation method enables accurate near-real-time modeling of complex systems and large datasets.
About
The Integrated Nested Laplace Approximation (INLA) method that revolutionized the speed and accuracy of modeling a little over 10 years ago has received a speed and efficiency upgrade through work by researchers from KAUST.
This advance brings the promise of real-time modeling of large multidimensional datasets typically found in spatial and environmental applications.
Large datasets that consist of many variables or locations and data over time and space are notoriously difficult to interpret. Far beyond human comprehension, these datasets need ways to be processed and statistically reformulated so the data can form an explanatory model.
Using such large data in a complex model is incredibly computationally intensive, even with today’s supercomputers, so statisticians have developed a range of approximation methods to reduce computational time while achieving a reasonably accurate model.
“When INLA was proposed in 2009, it was considered a breakthrough because of its accuracy in a very short computation time compared with other methods popular at that time,” says KAUST research scientist Janet van Niekerk.
Before INLA, the go-to approximation approach was the Markov chain Monte Carlo (MCMC) method, which involves subsampling of data points along a chain to produce an approximation with a smaller dataset. This approach can deal with any kind of model but remains computationally intensive and can suffer from “convergence“ issues that lead to erroneous results.
Håvard Rue proposed INLA in 2009 as a way of using prior information known about the data to get faster and more accurate approximations for models with a latent Gaussian structure — a typical “normal” bell-curve distribution. Such “Bayesian” methods begin with a prior belief about a model, then update that belief by learning from the data. This approach works remarkably well for various models and particularly spatial and temporal models.
Now, van Niekerk, with Rue and colleagues Elias Krainski and Denis Rustand, have seen an opportunity to improve INLA further by replacing a key component in the method.
“INLA has always been a fast and accurate alternative to MCMC in the case of latent Gaussian models, but the original proposal became troublesome on large datasets and for near real-time analysis,” says van Niekerk.
Based on new work by the Statistics Group on correcting Laplace approximations with “variational Bayes“ — replacing a linear predictor with a more efficient way to update the belief about the model after learning from the data — the team was able to reformulate this step to do the same as before but much faster and for much larger datasets and more complex models.
“This work is a step towards near real-time modeling for high volume data and where fast inference is needed, such as in personalized medicine,” van Niekerk says.
For more KAUST Research stories, visit KAUST Discovery.
REFERENCE
- Van Niekerk, J., Krainski, E., Rustand, D. & Rue, H. (2023). A new avenue for Bayesian inference with INLA. Computational Statistics & Data Analysis 181, 107692 (2023). | article