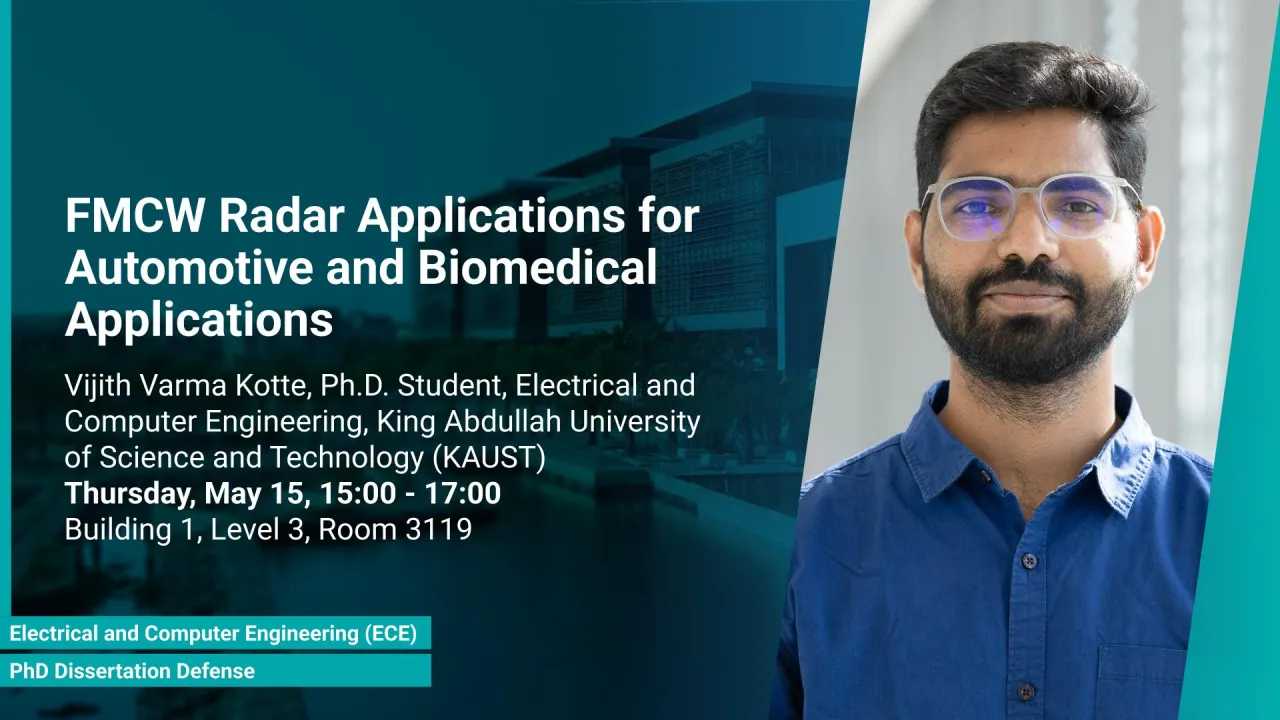
FMCW Radar Applications for Automotive and Biomedical Applications
This dissertation enhances Frequency Modulated Continuous Wave (FMCW) radar effectiveness for automotive forward-looking Synthetic Aperture Radar (SAR) imaging and biomedical vital sign and dehydration monitoring by developing novel methodologies based on advanced signal processing, deep learning, and MIMO radar techniques.
Overview
Frequency Modulated Continuous Wave (FMCW) radar has emerged as a powerful sensing modality in both automotive and biomedical applications due to its ability to provide precise range, velocity, and Doppler measurements. This dissertation investigates novel methodologies to enhance FMCW radar's effectiveness in two critical domains: (1) forward-looking Synthetic Aperture Radar (SAR) imaging for automotive applications, and (2) biomedical monitoring for non-contact vital sign estimation and dehydration assessment. The proposed approaches leverage advanced signal processing, deep learning, and MIMO radar techniques to improve spatial resolution, classification accuracy, and robustness in real-world scenarios.
In the automotive domain, the research focuses on improving the azimuthal resolution of forward-looking SAR imaging by incorporating MIMO radar and deep learning-based reconstruction methods. Two key methodologies are proposed to address the resolution limitations of conventional SAR imaging techniques. The first approach employs an unsupervised Deep Basis Pursuit (DBP) framework, which enhances resolution through sparse recovery, significantly improving target scene clarity. The second approach utilizes a Convolutional Decoder for forward-looking MIMO SAR imaging, enabling high fidelity scene reconstruction in low measurement scenarios. These deep learning-based methods effectively mitigate the challenges associated with limited aperture size and mechanical scanning constraints, making them highly suitable for automotive radar applications, such as autonomous navigation and obstacle detection.
In the biomedical domain, this dissertation explores two critical applications: heart rate and breathing rate estimation from FMCW radar and non-invasive dehydration status monitoring. A novel joint estimation algorithm for high amplitude difference Doppler frequencies is introduced to accurately extract cardiac and respiratory signals from radar data, overcoming limitations posed by amplitude disparities in physiological motion. Additionally, a dehydration classification framework is developed using 77 GHz FMCW radar, combined with Wavelet Scattering Transform (WST) for feature extraction and machine learning classifiers for dehydration state identification. A custom fasting dataset is utilized for validation, demonstrating the feasibility of radar based hydration monitoring for continuous and remote healthcare applications.
The methodologies proposed in this dissertation are rigorously validated through extensive simulations and real-world experiments, showcasing their effectiveness in enhancing FMCW radar's performance for both automotive and biomedical applications. The findings contribute to the advancement of high-resolution radar imaging, physiological monitoring, and intelligent sensing technologies, paving the way for next generation autonomous systems and healthcare solutions.
Presenters
Brief Biography
Vijith Varma Kotte is a Ph.D. candidate in Electrical and Computer Engineering at King Abdullah University of Science and Technology (KAUST), Saudi Arabia. He joined KAUST in 2020 under the supervision of Prof. Tareq Y. Al-Naffouri. He received his B.Tech. degree in Electronics and Communication Engineering from Amrita School of Engineering, Bengaluru, India, in 2015, and his M.Tech. degree from Indian Institute of Technology Delhi (IIT Delhi) in 2018.
Prior to joining KAUST, Vijith worked as a Research Engineer at the Centre for Development of Telematics (C-DOT) in New Delhi from 2018 to 2019.