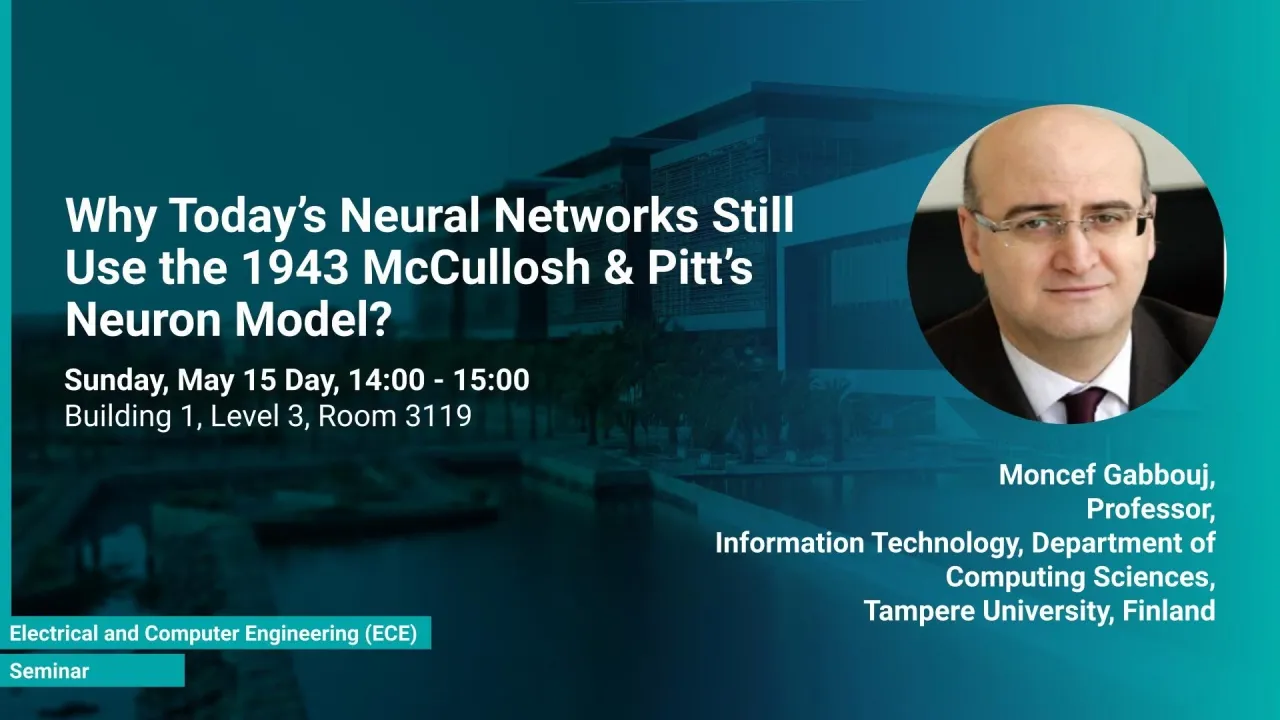
Why Today’s Neural Networks Still Use the 1943 McCullosh and Pitt’s Neuron Model?
- Prof. Moncef Gabbouj, Department of Computing Sciences, Tampere University
B1 L3 R3119
Operational Neural Networks (ONNs) are new generation network models targeting to address two major drawbacks of conventional Convolutional Neural Networks (CNNs): the homogenous network configuration and the “linear” neuron model that can only perform linear transformations over previous layer outputs. ONNs can perform any linear or non-linear transformation with a proper combination of “nodal” and “pool” operators.
Overview
Abstract
Operational Neural Networks (ONNs) are new generation network models targeting to address two major drawbacks of conventional Convolutional Neural Networks (CNNs): the homogenous network configuration and the “linear” neuron model that can only perform linear transformations over previous layer outputs. ONNs can perform any linear or non-linear transformation with a proper combination of “nodal” and “pool” operators. This is a great leap towards expanding the neuron’s learning capacity in ONNs, requiring the use of a single nodal operator for all synaptic connections of every neuron. This restriction has recently been lifted by introducing a superior neuron called the “generative neuron” where each nodal operator can be customized during the training in order to maximize learning. As a result, the network is able to self-organize the nodal operators of its neurons’ connections. Self-Organized ONNs (Self-ONNs) composed with superior generative neurons can achieve diversity even with a compact configuration. We shall explore several signal processing applications of neural network models equipped with the superior neuron.
Brief Biography
Moncef Gabbouj received his BS degree in 1985 from Oklahoma State University, and his MS and PhD degrees from Purdue University, in 1986 and 1989, respectively, all in electrical engineering. Dr. Gabbouj is a Professor of Information Technology at the Department of Computing Sciences, Tampere University, Tampere, Finland. He was Academy of Finland Professor during 2011-2015. His research interests include Big Data analytics, multimedia content-based analysis, indexing and retrieval, artificial intelligence, machine learning, pattern recognition, nonlinear signal and image processing and analysis, voice conversion, and video processing and coding. Dr. Gabbouj is a Fellow of the IEEE and member of the Academia Europaea and the Finnish Academy of Science and Letters. He is the past Chairman of the IEEE CAS TC on DSP and committee member of the IEEE Fourier Award for Signal Processing. He served as associate editor and guest editor of many IEEE, and international journals and Distinguished Lecturer for the IEEE CASS. Dr. Gabbouj served as General Co-Chair of IEEE ISCAS 2019, ICIP 2020 and ICME 2021. Gabbouj is Finland Site Director of the USA NSF IUCRC funded Center for Visual and Decision Informatics (CVDI) and leads the Artificial Intelligence Research Task Force of Finland’s Ministry of Economic Affairs and Employment funded Research Alliance on Autonomous Systems (RAAS).