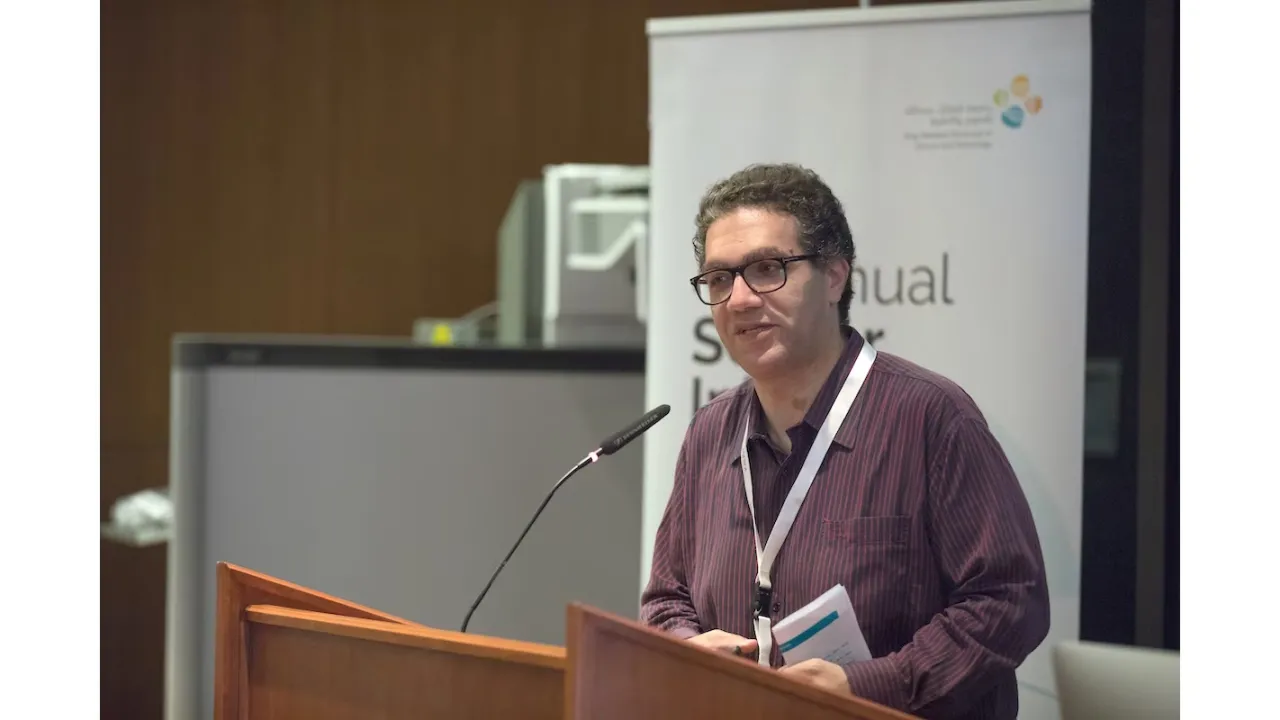
Prof. Salama's paper hits the top for 6 months in the row!
In August 2016, Prof. Salama's article "Stochasticity Modeling in Memristors", was for the sixth time consecutively among the most downloaded paper of the month and the most read article in the IEEE Transactions on Nanotechnology (TNANO).
About
In August 2016, Prof. Salama's article "Stochasticity Modeling in Memristors", was for the sixth time consecutively among the most downloaded paper of the month and the most read article in the IEEE Transactions on Nanotechnology (TNANO).
"The real world is becoming more and more a source of big data problems" explains the team-leader Khaled Nabil Salama, professor of electrical engineering and principal investigator of the Sensors Lab, CEMSE Division, KAUST. "If we were to solve these problems with contemporary systems, we would need huge computing power. Today, we have an alternative though, Stochastic Computing is offering a new way to go cheaply and power efficiently for dealing with 'big data'."
In the article, Salama's team proposed an innovative neural circuit that relies on the stochasticity of the memristor - a nano-scale multi-state device able to switch between states changing its resistive properties in a non-deterministic pattern. The importance of the researcher resides in proposing a new general model for incorporating stochasticity into the modeling of memristors' behavior, whose performance is one of the major obstacles for the development of artificial neural networks.
For the past 3 years, PhD student Rawan Naous and, Master student Maruan al-Shedivat, from the research group of Professor Salama, have being focusing their experiments on new approaches to demonstrate that artificial networks of neural-like computing units can mimic the cognitive performances of a living brain such as visual perception, language understanding, and learning skills. They found out that stochasticity - in other words, the randomness - of memristors' behavior was one of the missing elements to be taken into consideration.
"In our laboratory, we explore brain-inspired probabilistic mechanisms, such as neural and synaptic stochasticity, in the context of generative models" Al Shedivat says "The two questions we want to answer here are: what kind of models can describe a neural learning system built of stochastic components and how can we develop such systems."
The team answered these two questions enhancing the general deterministic model of the memristor to account for abrupt spontaneous switching. "Our model was capable of exhibiting deterministic behavior along with sharp, spontaneous state jumps with a probability that depended on the applied voltage," explains Rawan Naous, the lead author of the work.
The researchers' findings are published in IEEE Transactions on Nanotechnology vol. 15, no. 1, pp. 15-28, 2016 . DOI:10.1109/TNANO.2015.2493960:
http://ieeexplore.ieee.org/xpl/articleDetails.jsp?arnumber=7305797