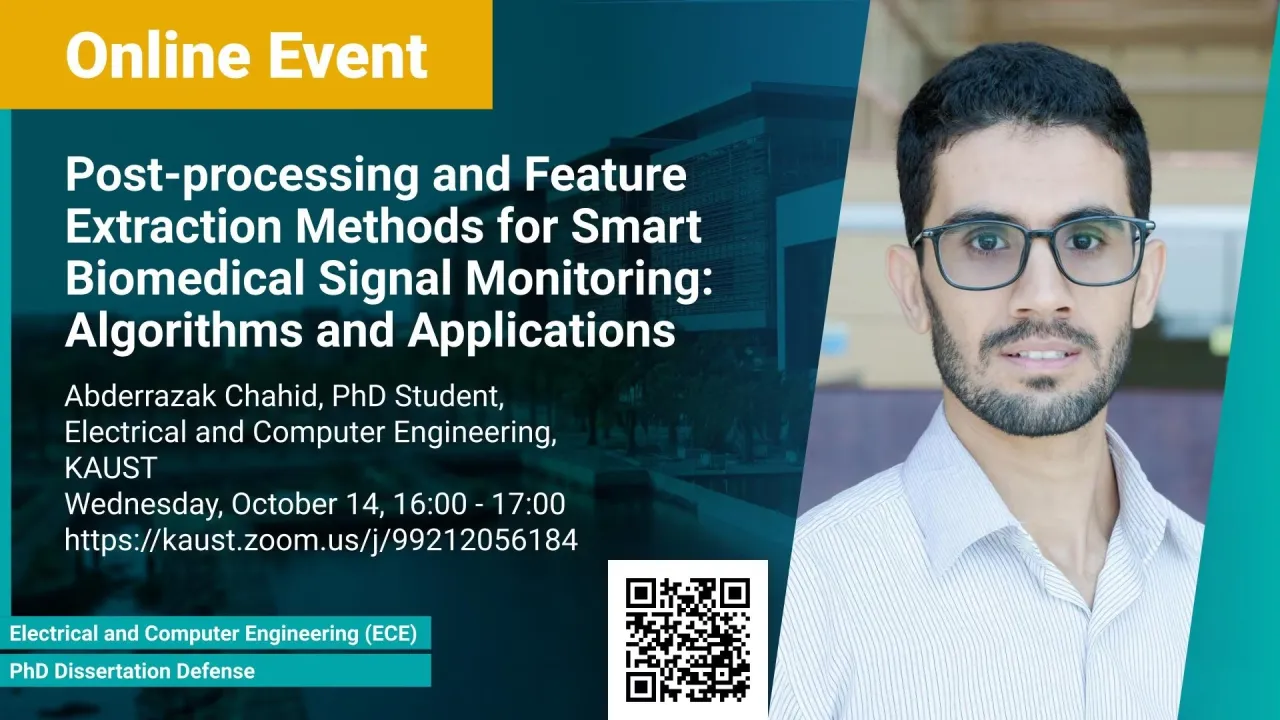
Post-processing and Feature Extraction Methods for Smart Biomedical Signal Monitoring: Algorithms and Applications
Digital health solutions improve healthcare services and help achieve sustainable and higher standards of health and well-being. These solutions are mainly based on Digital Signal Processing (DSP) to record, interpret, and diagnose bio-signals such as Electrocardiogram (ECG) or Magnetoencephalography (MEG). In my thesis, a novel signal/image post-processing algorithm is proposed based on the Semi-Classical Signal Analysis method (SCSA) to enhance biomedical data quality. In addition, new feature extraction algorithms are proposed, based on the SCSA and the new Quantization-based Position Weight Matrix (QuPWM), which opens new tracks toward smart biomedical diagnosis and decision-making assistance in different fields such as predicting true Poly(A) regions in a DNA sequence, multiple hand gesture prediction.
Overview
Abstract
Human health is monitored through several physiological measurements, such as heart rate, blood pressure, oxygen saturation levels, brain activity, etc. These measurements are taken at predefined points in the body and recorded as temporal signals or colorful images for diagnosis purposes. Therefore, the post-processing of these noisy signals and images is a crucial need to provide clinicians with useful information to make the right decisions. Many research studies have been conducted to process signals and images using a variety of mathematical formulas and algorithms.
In my work, I first explored the biomedical field from the signal processing aspect. I showed the usefulness of a novel signal/image post-processing algorithm, based on the Semi-Classical Signal Analysis method (SCSA), to enhance the quality of biomedical signals and images. This approach shows great potential in denoising, and residual water-peak suppression for Magnetic Resonance Spectroscopy (MRS) signals compared to the existing methods. In addition, it shows very promising noise removal in particular from pulse shaped signals and from Magnetic Resonance (MR) images. All these results are achieved thanks to the non-locality of the eigenfunctions of a Semi-Classical Schrodinger operator, which led us to think about extracting more informative features from the recordings in order to perform more advanced processing. To do so, I shifted toward using the SCSA algorithm for feature extraction and combining it with the machine learning models to predict epileptic spikes in Magnetoencephalography (MEG). The SCSA-based features give comparable and encouraging results compared to the existing methods. Moreover, I proposed a new Quantization-based Position Weight Matrix (QuPWM) method. The proposed features have lightened new tracks toward more advancements in biomedical signal classification, such as predicting true Poly(A) regions in a DNA sequence, multiple hand gesture prediction, etc.
Brief Biography
Abderrazak Chahid is a PhD candidate in Electrical and Computer Engineering department within the CEMSE division. He obtained his Bachelor of Science degree in Electrical Engineering and Power Electronics from Sultan Moulay Slimane University in Morocco in 2010. He then got a Master degree in Electrical Engineering from the National School of Applied Sciences in Morocco in 2013 before obtaining a second Master of Science degree in Embedded Systems from Lorraine University in France.