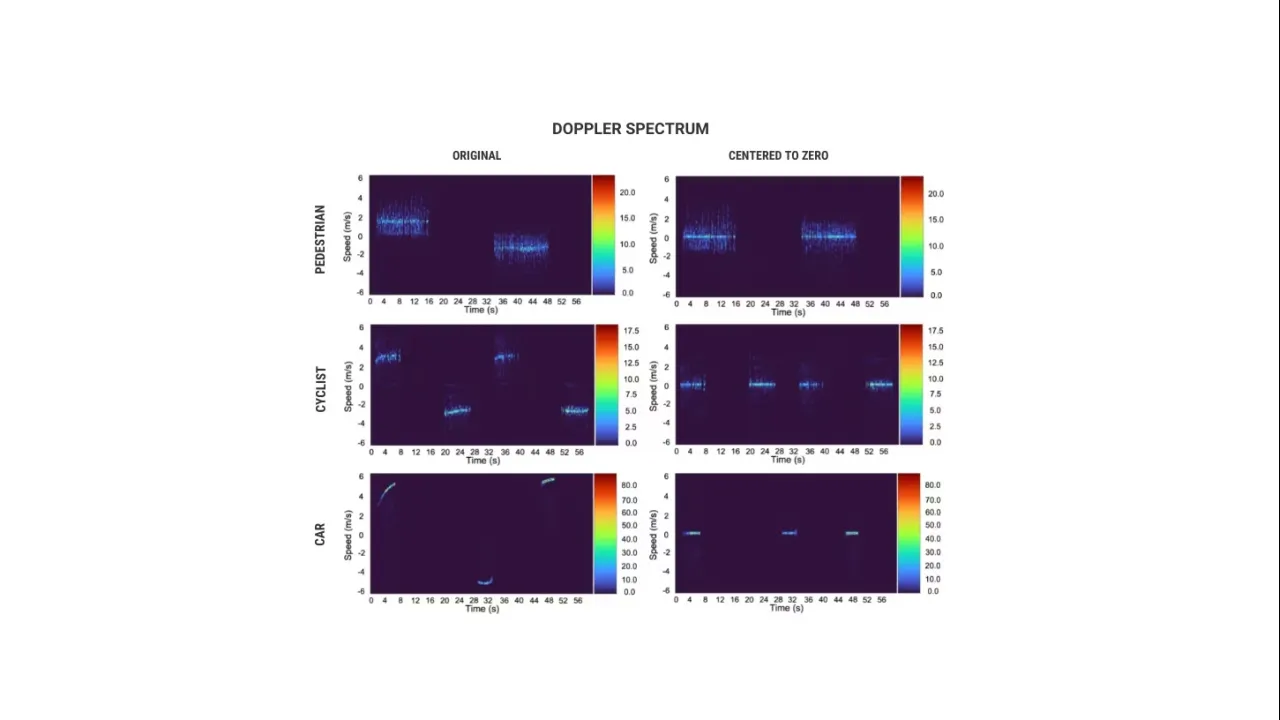
KAUST research wins Radar Challenge at the IEEE Radar Conference
KAUST M.S. Graduate Rudi Coppola, KAUST Research Scientist Dr. Sajid Ahmed, and KAUST Distinguished Professor Dr. Mohamed-Slim Alouini won first prize at the Institute of Electrical and Electronics Engineers (IEEE) Radar Challenge 2021. Their work titled "Road Users Classification Based on Bi-Frame Micro-Doppler with 24-GHz FMCW Radar," presents a case study for a 24 Gigahertz (GHz) frequency modulated continuous wave radar module.
About
By David Murphy
Research by KAUST M.S. Graduate Rudi Coppola, now Ph.D. student at TU Delft, Netherlands, KAUST Research Scientist Dr. Sajid Ahmed, and KAUST Distinguished Professor Dr. Mohamed-Slim Alouini won first prize at the Institute of Electrical and Electronics Engineers (IEEE) Radar Challenge 2021. Hosted by the 2021 IEEE Radar Conference, the challenge was created to build “a community of radar builders that collectively explore the art of the possible in radar applications.”
The work, authored by Coppola as his final M.S. thesis, titled "Road Users Classification Based on Bi-Frame Micro-Doppler with 24-GHz FMCW Radar," presents a case study for a 24 Gigahertz (GHz) frequency modulated continuous wave radar module. The unique properties of radar systems offer the unparalleled ability to estimate distance and hold motion information accurately, penetrate nonmetallic objects, and remain unaffected by surrounding weather conditions.
“Electromagnetic waves centered at frequencies around 24 GHz offer high precision target measurements, compact antenna, and circuitry design, and lower atmospheric absorption than higher frequency-based systems,” Coppola explained.
The thesis investigates how to leverage these estimates and develop an algorithm to recognize different road users, namely pedestrians, cyclists, and cars.
“Besides obvious geometrical differences, each target moves in a unique way: high-frequency radars are especially sensitive to capture (micro-)motions due to a phenomenon called Doppler Effect. This is crucial for the purpose of target classification,” continues Coppola.
The researchers present the classification accuracy—a metric used to summarize the performance of machine learning classification models— for the three classes, specifically pedestrians, cyclists, and cars. The resulting classification features were designed based on theoretical models, and subsequent experiments validated their effectiveness.
“The features are extracted from the available geometrical and motion-related information and used to train different machine learning-based classification models to compare the results,” Coppola noted.
“The proposed technology can be used in various applications such as autonomous vehicles, perimeter surveillance, and to help visually impaired people,” Ahmed concluded.
This recognition by the IEEE is one of a quick succession of personal accolades for Coppola, who, together with Ahmed and Alouini, submitted a patent and received acceptance for publication in Frontiers in Signal Processing on the research topic “Machine Learning Methods for Radar Detection, Classification/Estimation, and Tracking.”