Teaching has the power to test the limits of one's knowledge. Teaching algorithms to learn using machine learning is making it possible for cars to do away with human drivers in the near future, but this has also opened up new questions about the limits of our knowledge of the brain and learning.
Bernard Ghanem, KAUST associate professor of electrical engineering from the University's Visual Computing Center and principal investigator of the Image and Video Understanding Lab, applies machine learning techniques to computer vision for automated navigation (for example, self-driving cars and self-flying unmanned aerial vehicles [UAVs]), video content searching and object tracking, among other topics.
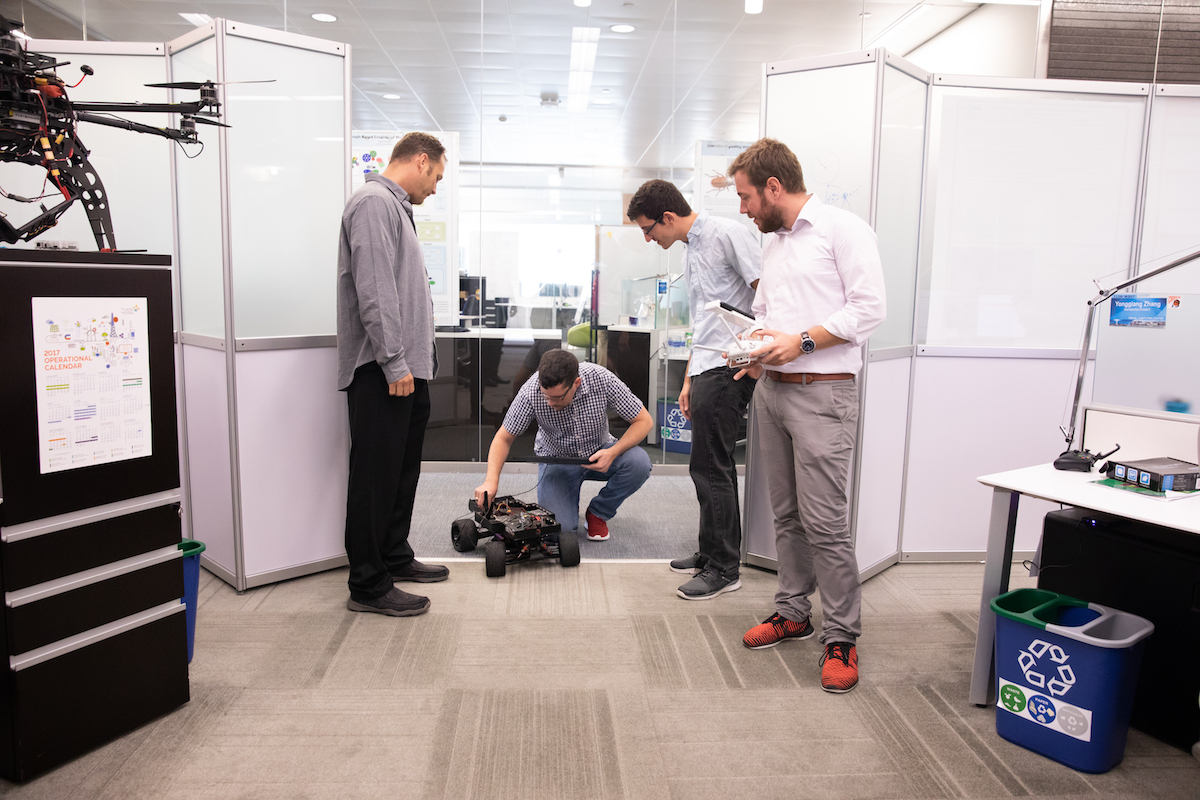
"Our brains can do complicated tasks like activity and object recognition with low energy consumption, yet we are doing research to understand how we can replicate this functionality in a machine. I find it very interesting that we are aiming to imitate a system that we have inside [of] us. It basically means we don't really understand how our brains work," explained Ghanem.
Man vs. machine
"Creativity is a specific intelligence that is central to what it means to be human. A creative algorithm would be real artificial intelligence—possessing the ability to create. Humans are able to transfer skills from task to task, evolving knowledge without direct training. That's creative because something was learned without being taught. We'd love to reach creativity in machine learning, but we're not at that point yet," Ghanem said.
When developing machine learning algorithms with the ability to create, researchers must first understand what mechanism underlies the transfer of knowledge from one task to another. There are a variety of challenges to overcome, but Ghanem surmises that perhaps researchers need to question the fundamentals: is deep learning the right technique?; what type of learning does this skill require?; and even if we assume deep neural networks is the best method, how do you actually teach transfer?
Read the full article